Development and Validation of a Prediction Model for Differentiation of Benign and Malignant Fat-Poor Renal Tumors Using CT Radiomics
APPLIED SCIENCES-BASEL(2023)
摘要
Objectives: To develop and validate a machine learning-based CT radiomics classification model for distinguishing benign renal tumors from malignant renal tumors. Methods: We reviewed 499 patients who underwent nephrectomy for solid renal tumors at our institution between 2003 and 2021. In this retrospective study, patients who had undergone a computed tomography (CT) scan within 3 months before surgery were included. We randomly divided the dataset in a stratified manner as follows: 75% as the training set and 25% as the test set. By using various feature selection methods and a dimensionality reduction method exclusively for the training set, we selected 160 radiomic features out of 1,288 radiomic features to classify malignant renal tumors. Results: The training set included 396 patients, and the test set included 103 patients. The percentage of extracted radiomic features from patients was 32% (385/1218) after the reproducibility test. In terms of the average Area Under the Receiver Operating Characteristic Curve (AU-ROC) and the average Area Under the Precision-Recall Curve (AU-PRC), the Random Forest model achieved better performance (AU-ROC = 0.725; AU-PRC = 0.899). An average accuracy of 0.778 was obtained on evaluation with the hold-out test set. At the optimal threshold, the Random Forest model showed an F1 score of 0.746, precision of 0.862, sensitivity of 0.657, specificity of 0.651, and Negative Predictive Value (NPV) of 0.364. Conclusions: Our machine learning-based CT radiomics classification model performed well for the independent test set, indicating that it could be a useful tool for discriminating between malignant and benign solid renal tumors.
更多查看译文
关键词
tumors,malignant,prediction model,fat-poor
AI 理解论文
溯源树
样例
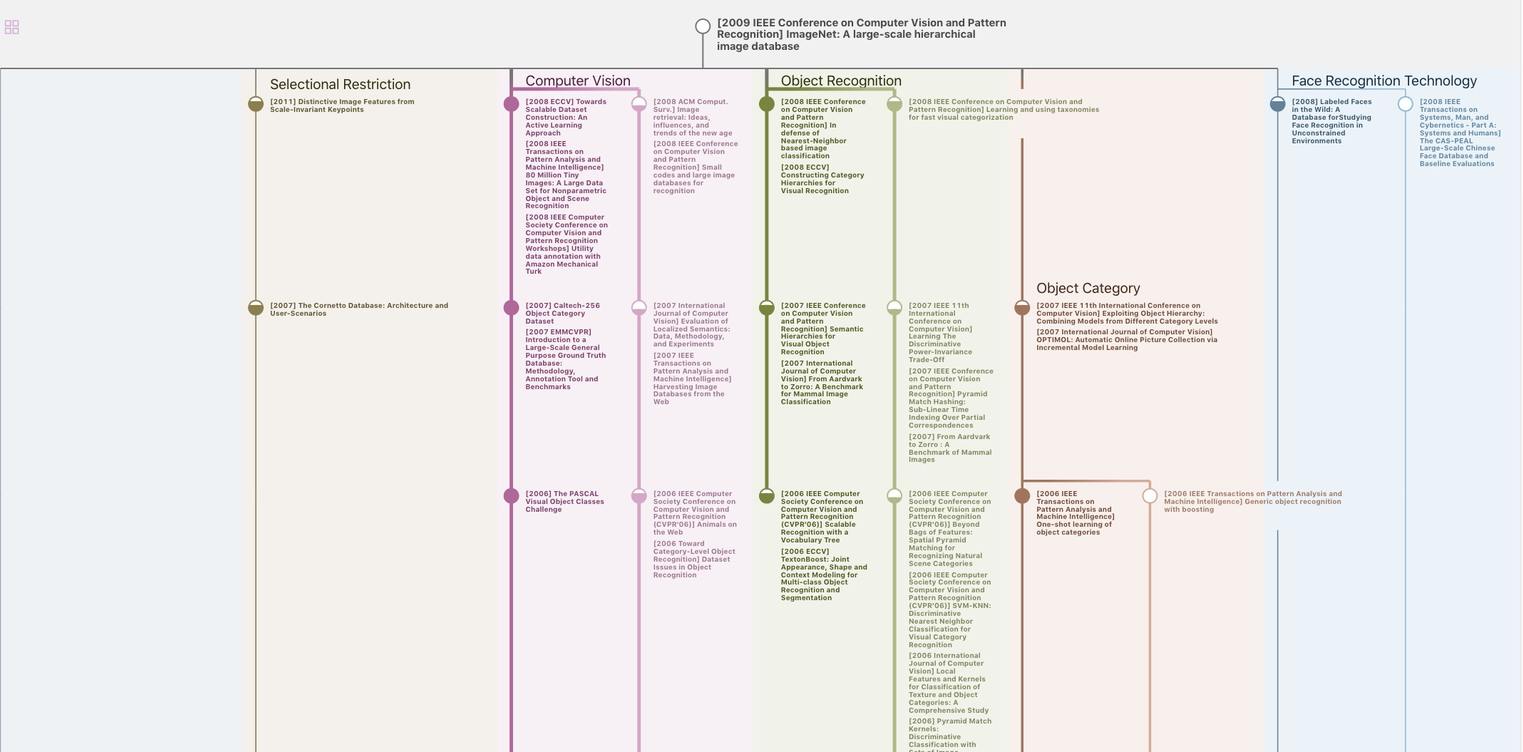
生成溯源树,研究论文发展脉络
Chat Paper
正在生成论文摘要