Magnetic tile fault detection of high-voltage electric machines: A multiview feature selection method via consistent soft label
INTERNATIONAL JOURNAL OF CIRCUIT THEORY AND APPLICATIONS(2023)
摘要
Image-based scheme has attracted wide attention in the fault detection of high-voltage permanent magnet motors, but it often suffers from the shooting conditions. Multiview feature selection allows multisource information to be fused, which can improve the accuracy and robustness of image detection. Therefore, we propose multiview unsupervised consistency via soft-label feature selection (MUCSFS). This method constructs consistent pseudo labels through soft labels from clustering affinity of each view sample and builds the model by integrating selection constraints into the graph model. This model filters the fault data set to obtain the feature subset, which is used for clustering. We verify the method's effectiveness by simulating multiview data. The fault clustering experiment on the high-voltage motors' magnetic tile fault data set confirm that our method can effectively cluster the fault categories. A fault detection method based on the multiview feature selection method, MUCSFS, is proposed for a high-voltage motor magnet tile fault. This method constructs a consistent soft label by clustering the affinity of each view sample, and combines the L2,0 norm to obtain the feature subset for fault detection. image
更多查看译文
关键词
fault clustering, multiview learning, soft label, unsupervised feature selection
AI 理解论文
溯源树
样例
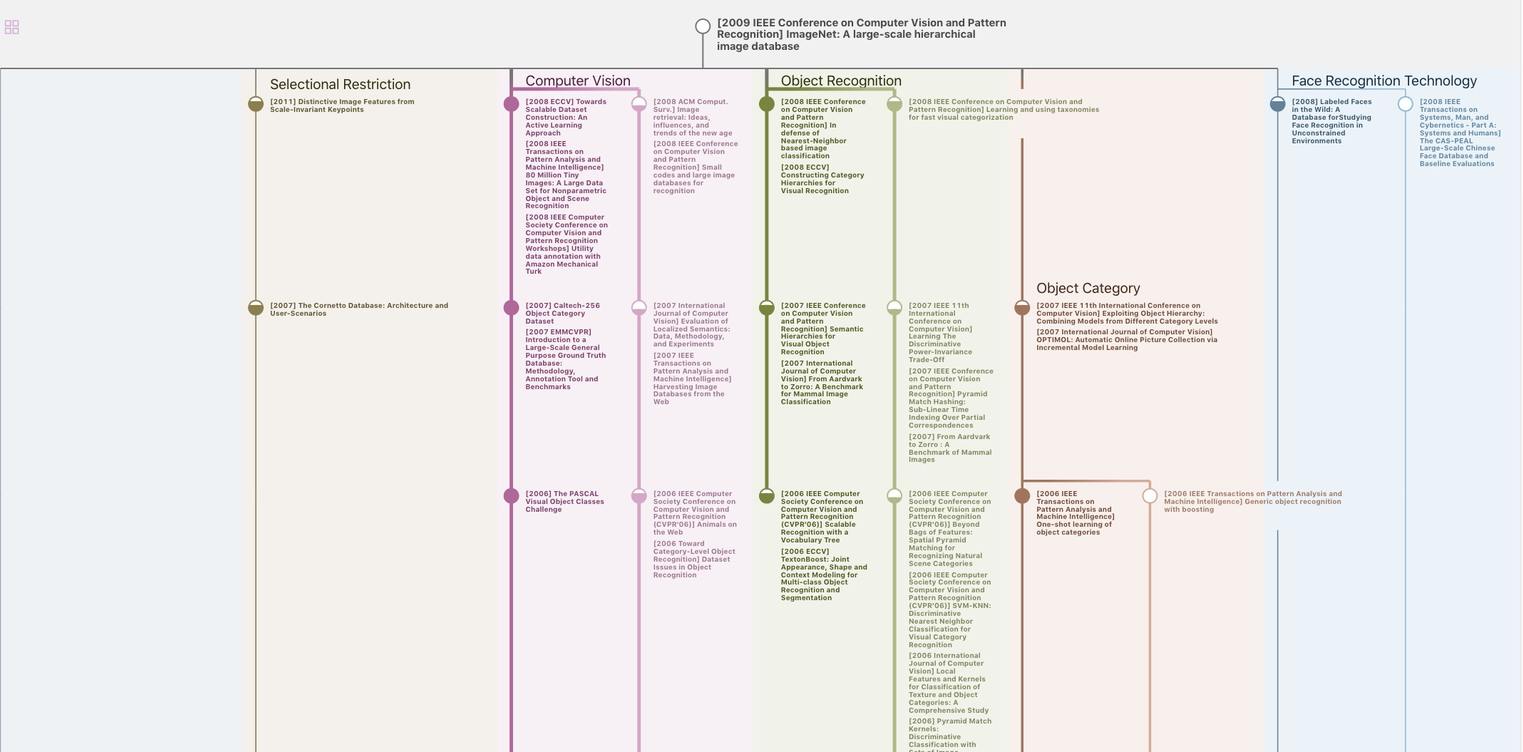
生成溯源树,研究论文发展脉络
Chat Paper
正在生成论文摘要