All-Day Vehicle Detection From Surveillance Videos Based on Illumination-Adjustable Generative Adversarial Network
IEEE TRANSACTIONS ON INTELLIGENT TRANSPORTATION SYSTEMS(2023)
摘要
Vehicle detection from surveillance videos is of great significance for various Intelligent Transportation System (ITS) applications. However, existing deep learning methods oftentimes fail under nighttime conditions on account of the lack of sufficient labeled nighttime data. To fill this gap, this paper proposes a novel framework for all-day vehicle detection, by introducing an illumination-adjustable GAN (IA-GAN). The IA-GAN transforms labeled daytime images into multiple nighttime images with diverse illumination, using an adjustable illumination vector as input. Notably, we utilize gray histogram distributions to automatically generate illumination labels, by which IA-GAN gains the knowledge of simulating lights. Following that, we construct a large dataset containing both labeled daytime images and all generated synthetic nighttime images with bounding box labels. Finally, a detector named Day-Night Balanced EfficientDet (DNBED) is developed for all-day vehicle detection. The experiments show that the proposed framework yields promising performance for all-day vehicle detection and competitive results for nighttime vehicle detection compared to existing GANs, indicating the effectiveness of proposed framework. The privacy-sanitized image data and its corresponding labels will be made publicly available at https://github.com/vvgoder/SEU_PML_Dataset.
更多查看译文
关键词
Computer vision,vehicle detection,generative adversarial network,adjustable illumination
AI 理解论文
溯源树
样例
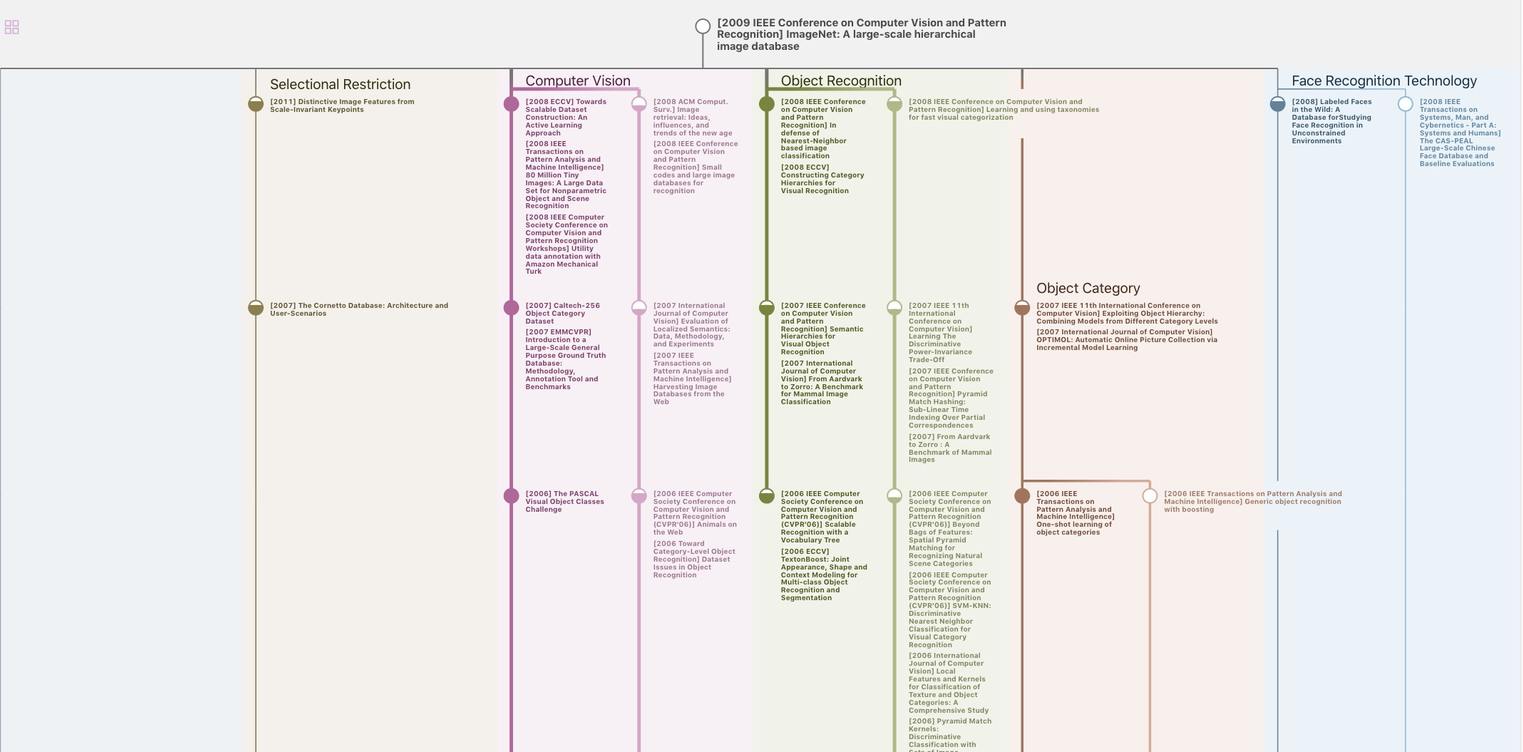
生成溯源树,研究论文发展脉络
Chat Paper
正在生成论文摘要