MC3Net: Multimodality Cross-Guided Compensation Coordination Network for RGB-T Crowd Counting
IEEE TRANSACTIONS ON INTELLIGENT TRANSPORTATION SYSTEMS(2023)
摘要
Owing to the expansion in processing of industrial information through advances in machine learning, the demand for accurate crowd counting in various applications is increasing.We propose a multimodality cross-guided compensation coor-dination network (MC3Net) for accurate red-green-blue and thermal (RGB-T) crowd counting. The network includes modules of intricate interactive fusion, feature difference compensation, and complementary attention enhancement. We use Conv Next as the backbone and process the three streams from RGB, thermal, and spliced RGB-T inputs. The multimodality data are sequentially guided and fused hierarchically, fully combining features extracted from the RGB and thermal images. There after, difference compensation is applied to compress fusion and splic-ing features. Redundant information is removed. Then, feature mismatch is mitigated to enhance complementary information, reduce the loss of details, and finally obtain crowd statistics. Results from extensive experiments on the RGBT-CC data setindicate the robustness and effectiveness of MC(3)Net, which also achieves high performance on the DroneRGBT dataset and Shanghai Tech RGBD dataset, outperforming existing crowdcounting methods. The code and models are available at:https://github.com/WBangG/MC3Net
更多查看译文
关键词
Complementary attention enhancement,cross-modality cross-guided fusion,feature difference compensation,RGB-T crowd counting
AI 理解论文
溯源树
样例
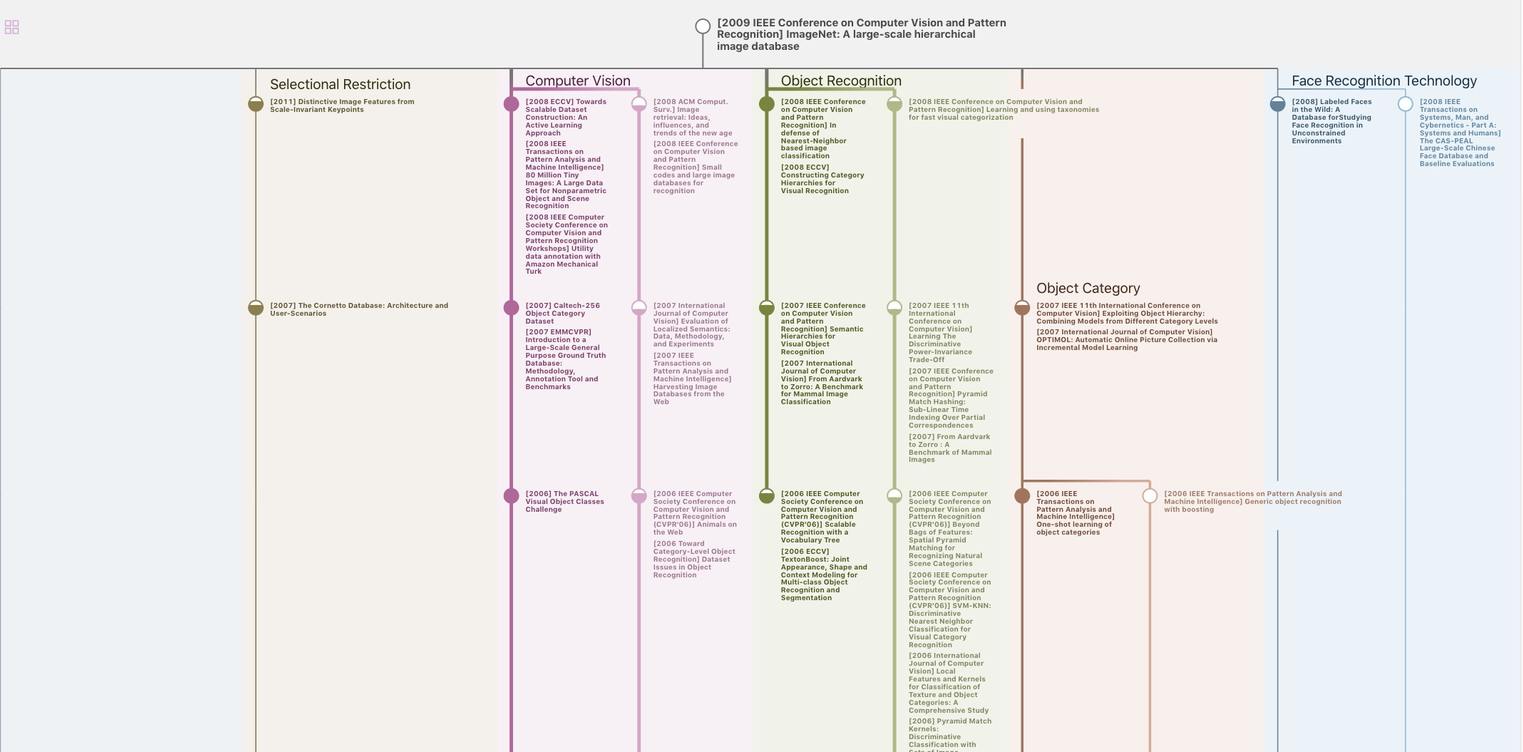
生成溯源树,研究论文发展脉络
Chat Paper
正在生成论文摘要