Continuous-Time and Discrete-Time Representation Learning for Origin-Destination Demand Prediction
IEEE TRANSACTIONS ON INTELLIGENT TRANSPORTATION SYSTEMS(2023)
摘要
Origin-Destination demand prediction is a fundamental and important task in the urban transportation system. It is more challenging and complex than region demand prediction since it needs to predict traffic demand for each pair of regions rather than a single region, which means $N<^>2$ time series need to be predicted given $N$ stations. Most existing works are mainly proposed for the region (or station) demand prediction. On the other hand, previous Origin-Destination demand prediction methods only follow a single discrete-time setting while the input data are continuous-time OD orders, which means these methods have not sufficiently leveraged the rich information for demand prediction. To solve these challenges, we propose a novel framework consisting of both continuous-time and discrete-time representation learning modules for Origin-Destination demand prediction. Firstly, we construct memorable representations of all nodes and design a continuous-time learning module to update the nodes' representations once a time-stamped OD transaction is observed. Then, a discrete-time representation learning module is proposed to generate discrete-time messages containing information across a fixed time interval from a macro perspective. Next, a co-updater module is designed to fuse messages from both continuous-time and discrete-time channels into node memory. Last, a graph attention module is developed, which generates final node embeddings using updated node memory and predicts the forthcoming OD demand matrix based on them. The experimental results on real-world datasets show that our method leads to significant and consistent improvements compared to other methods.
更多查看译文
关键词
Origin-destination demand prediction,continuous-time,discrete-time,urban transportation systems
AI 理解论文
溯源树
样例
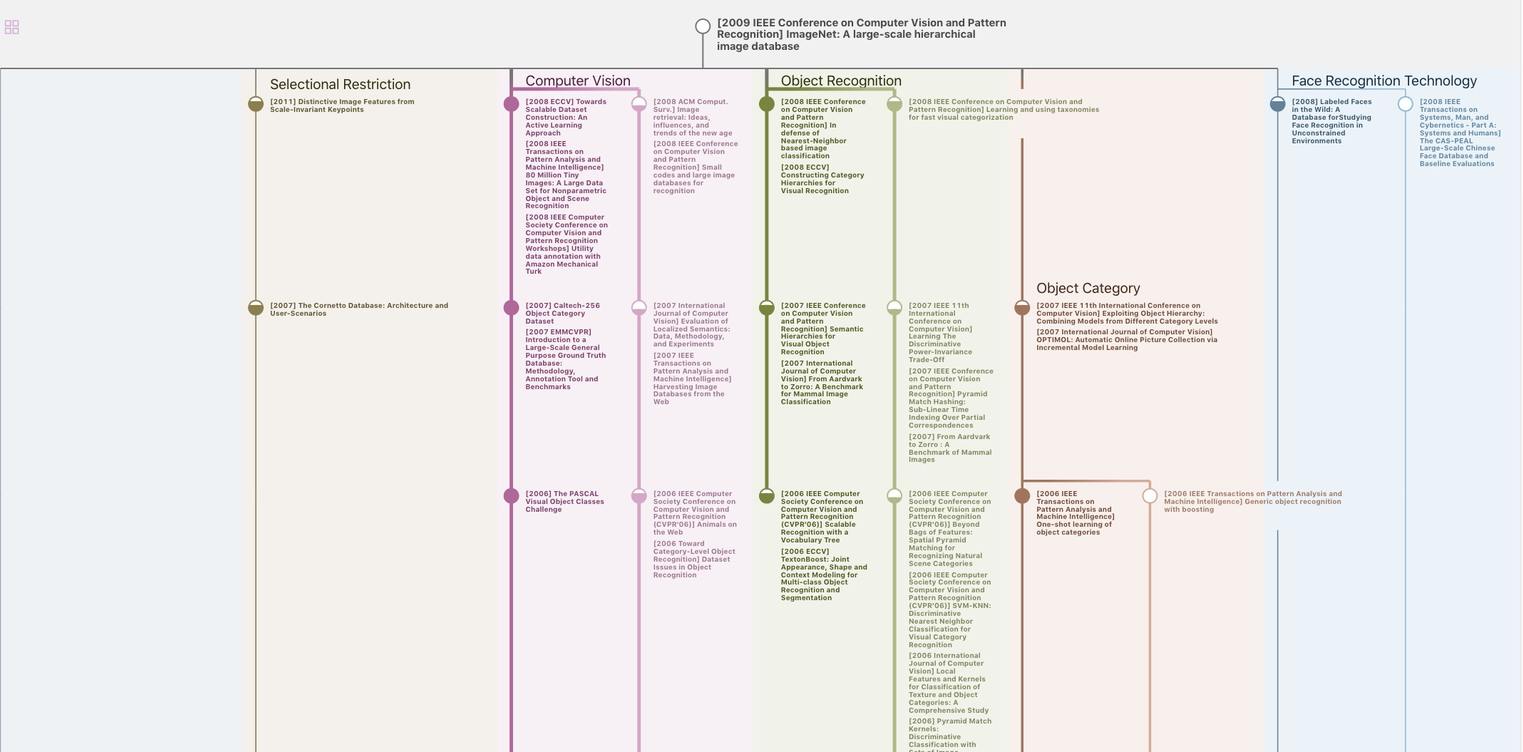
生成溯源树,研究论文发展脉络
Chat Paper
正在生成论文摘要