First-Principles Study Combined with Interpretable Machine-Learning Models of Bayesian Optimization for the Design of Ultrawide Bandgap Double Perovskites
JOURNAL OF PHYSICAL CHEMISTRY C(2023)
摘要
Recently, ultrawide bandgap semiconductor materials defined as those with a forbidden bandwidth greater than 3.4 eV have attracted much attention and received extensive research. These materials have important applications in extreme environment devices, deep or extreme ultraviolet optical devices, power devices, etc. However, very few ultrawide bandgap materials have been discovered in the field of double perovskite. We have designed a hierarchical material screening framework to rapidly discover novel ultrawide bandgap double perovskite materials that combine first-principles calculations and interpretable machine-learning models. This framework can solve the time-consuming and inefficient issues posed by traditional trial-and-error screening methods. In terms of machine learning, we have successfully established the mapping between material chemical components and material properties in which we adjusted the hyperparameters in the machine-learning algorithm by the Bayesian optimization method to achieve a higher prediction accuracy. We successfully screened four ultrawide bandgap double perovskites with a suitable forbidden bandwidth, excellent thermal stability, response range for deep UV light, and low electron effective mass from 71492 candidate materials. These four undiscovered ultrawide bandgap materials are expected to be synthesized and prepared in the future.
更多查看译文
关键词
bayesian optimization,bandgap,machine-learning machine-learning,first-principles
AI 理解论文
溯源树
样例
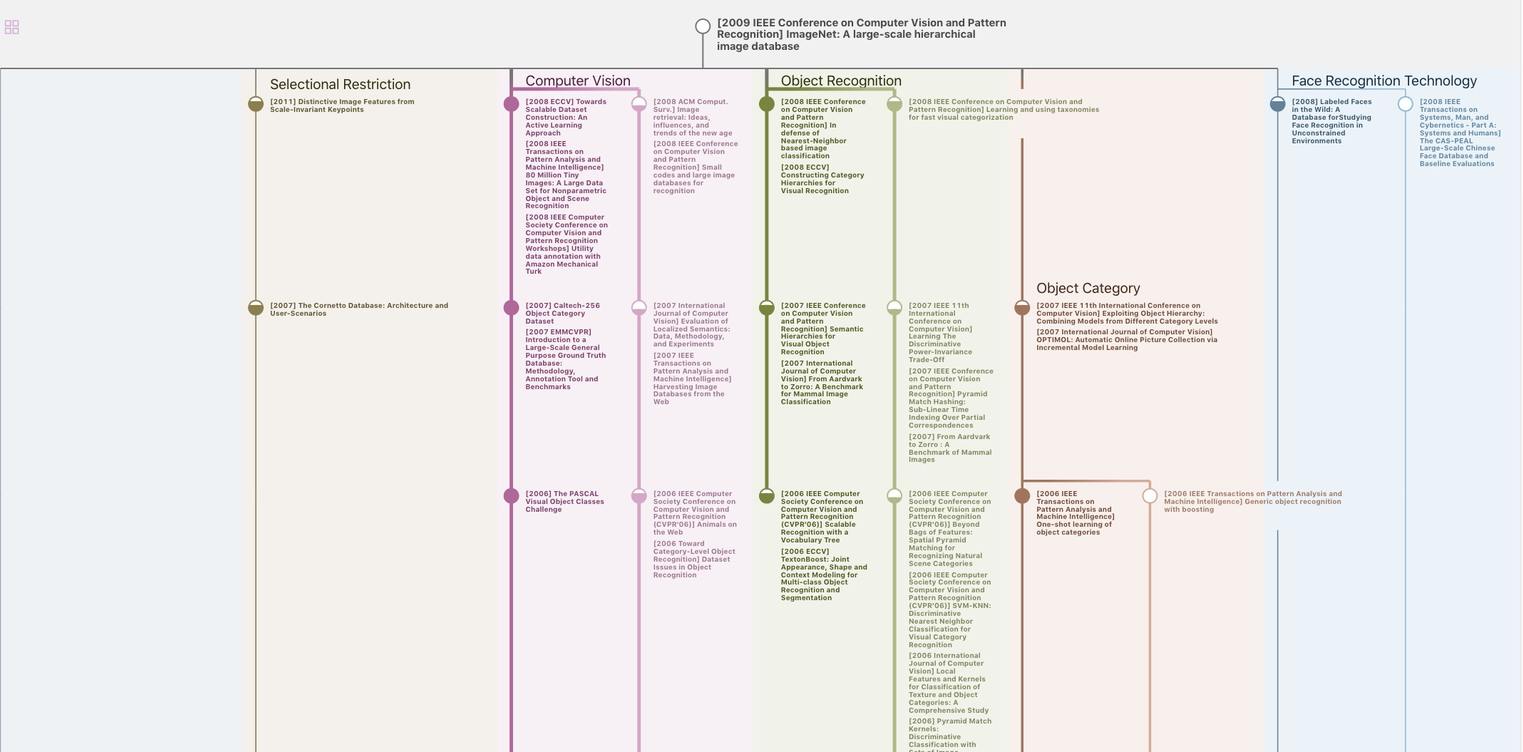
生成溯源树,研究论文发展脉络
Chat Paper
正在生成论文摘要