A scalable neural network architecture for self-supervised tomographic image reconstruction
DIGITAL DISCOVERY(2023)
摘要
We present a lightweight and scalable artificial neural network architecture which is used to reconstruct a tomographic image from a given sinogram. A self-supervised learning approach is used where the network iteratively generates an image that is then converted into a sinogram using the Radon transform; this new sinogram is then compared with the sinogram from the experimental dataset using a combined mean absolute error and structural similarity index measure loss function to update the weights of the network accordingly. We demonstrate that the network is able to reconstruct images that are larger than 1024 x 1024. Furthermore, it is shown that the new network is able to reconstruct images of higher quality than conventional reconstruction algorithms, such as the filtered back projection and iterative algorithms (SART, SIRT, CGLS), when sinograms with angular undersampling are used. The network is tested with simulated data as well as experimental synchrotron X-ray micro-tomography and X-ray diffraction computed tomography data. We present a lightweight and scalable artificial neural network architecture which is used to reconstruct a tomographic image from a given sinogram.
更多查看译文
关键词
scalable neural network architecture,neural network,reconstruction,self-supervised
AI 理解论文
溯源树
样例
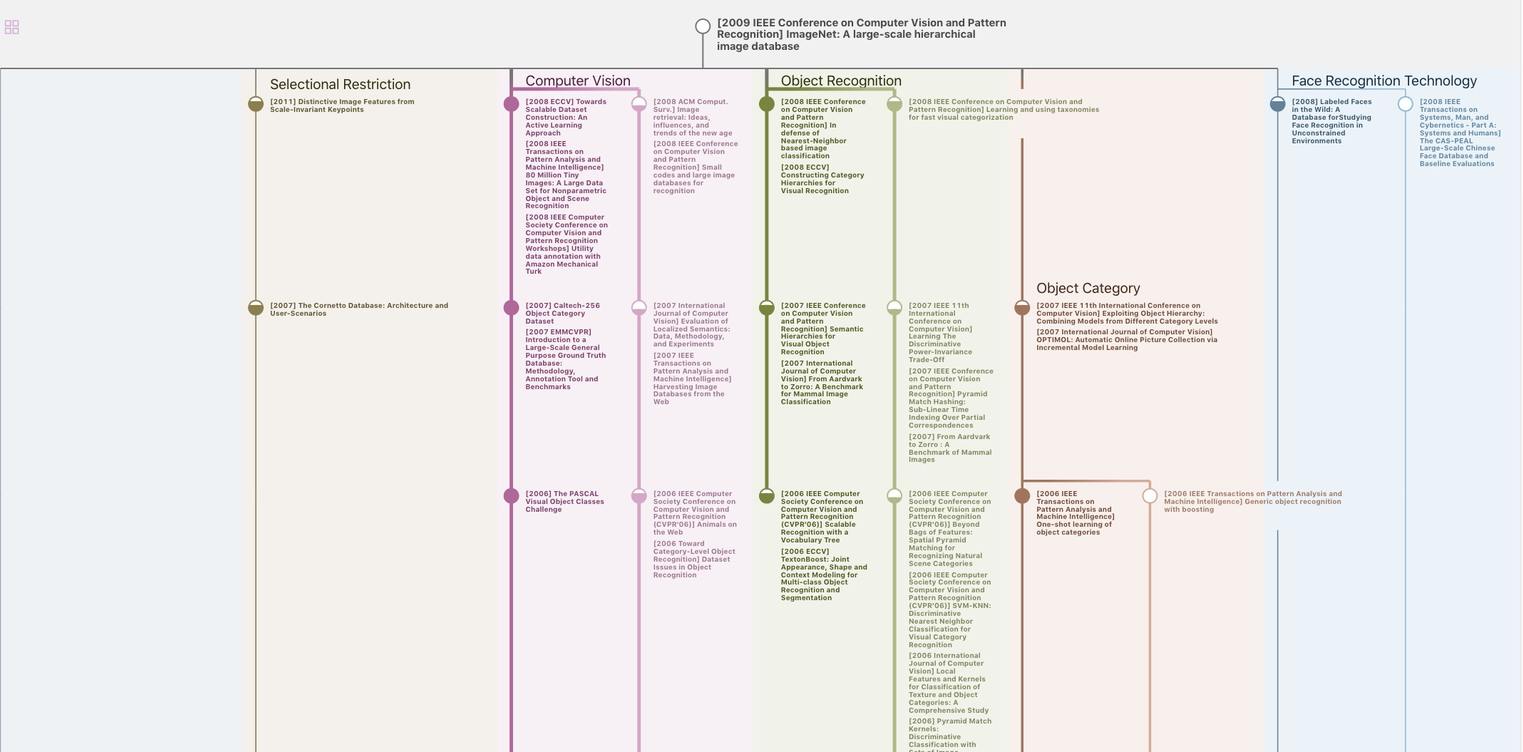
生成溯源树,研究论文发展脉络
Chat Paper
正在生成论文摘要