Enhancing CNNs Performance on Object Recognition Tasks with Gabor Initialization
ELECTRONICS(2023)
摘要
The use of Gabor filters in image processing has been well-established, and these filters are recognized for their exceptional feature extraction capabilities. These filters are usually applied through convolution. While convolutional neural networks (CNNs) are designed to learn optimal filters, little research exists regarding any advantages of initializing CNNs with Gabor filters. In this study, the performance of CNNs initialized with Gabor filters is compared to traditional CNNs with random initialization on six object recognition datasets. The results indicated that the Gabor-initialized CNNs outperformed the traditional CNNs in terms of accuracy, area under the curve, minimum loss, and convergence speed. A statistical analysis was performed to validate the performance of the classifiers, and the results showed that the Gabor classifiers outperformed the baseline classifiers. The findings of this study provide robust evidence in favor of using Gabor-based methods for initializing the receptive fields of CNN architectures.
更多查看译文
关键词
cnns performance,object recognition tasks,gabor initialization
AI 理解论文
溯源树
样例
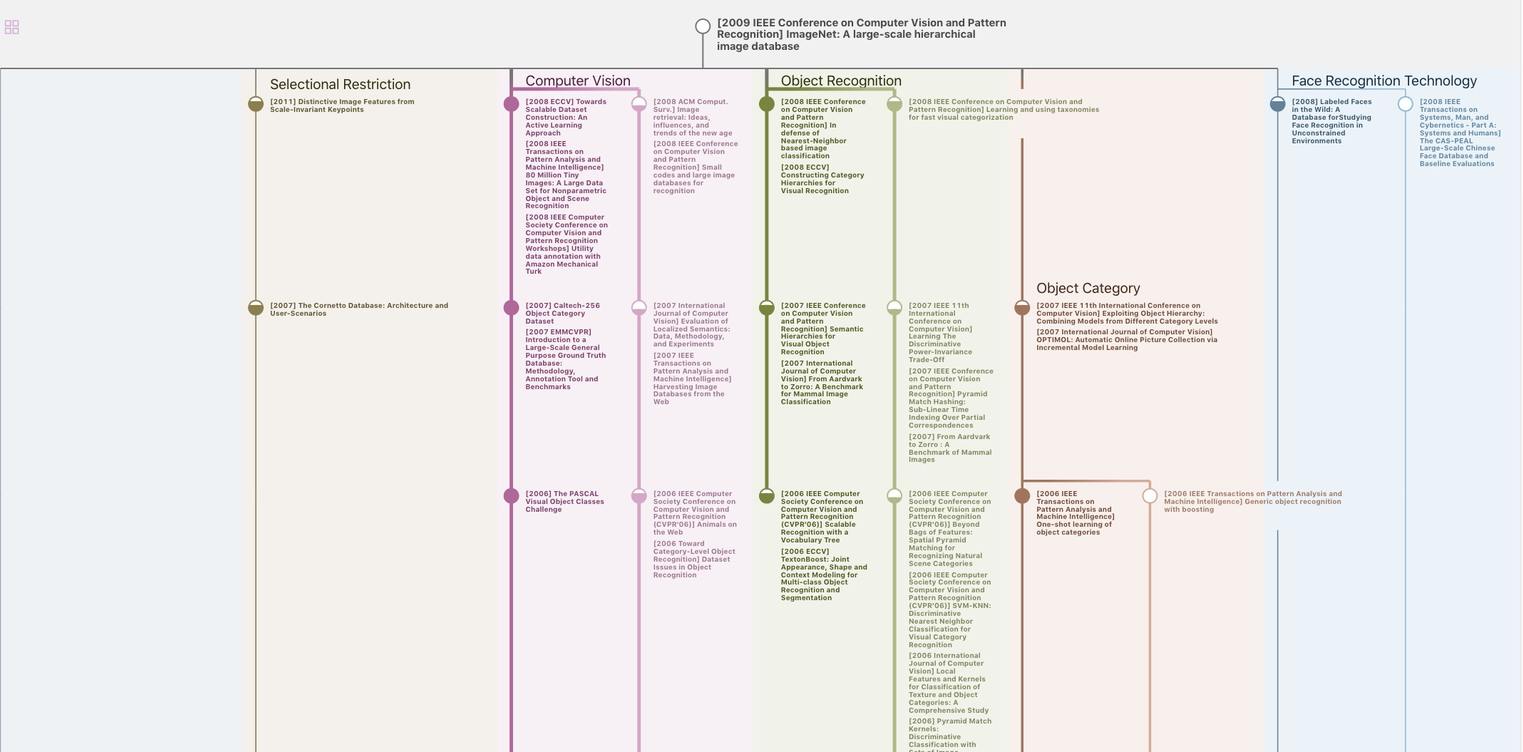
生成溯源树,研究论文发展脉络
Chat Paper
正在生成论文摘要