A Credit Card Fraud Detection Model Based on Multi-Feature Fusion and Generative Adversarial Network
CMC-COMPUTERS MATERIALS & CONTINUA(2023)
摘要
Credit Card Fraud Detection (CCFD) is an essential technology for banking institutions to control fraud risks and safeguard their reputation. Class imbalance and insufficient representation of feature data relating to credit card transactions are two prevalent issues in the current study field of CCFD, which significantly impact classification models' performance. To address these issues, this research proposes a novel CCFD model based on Multi feature Fusion and Generative Adversarial Networks (MFGAN). The MFGAN model consists of two modules: a multi-feature fusion module for integrating static and dynamic behavior data of cardholders into a unified high dimensional feature space, and a balance module based on the generative adversarial network to decrease the class imbalance ratio. The effectiveness of the MFGAN model is validated on two actual credit card datasets. The impacts of different class balance ratios on the performance of the four resampling models are analyzed, and the contribution of the two different modules to the performance of the MFGAN model is investigated via ablation experiments. Experimental results demonstrate that the proposed model does better than state-of-the-art models in terms of recall, F1, and Area Under the Curve (AUC) metrics, which means that the MFGAN model can help banks find more fraudulent transactions and reduce fraud losses.
更多查看译文
关键词
Credit card fraud detection, imbalanced classification, feature fusion, generative adversarial networks, anti-fraud systems
AI 理解论文
溯源树
样例
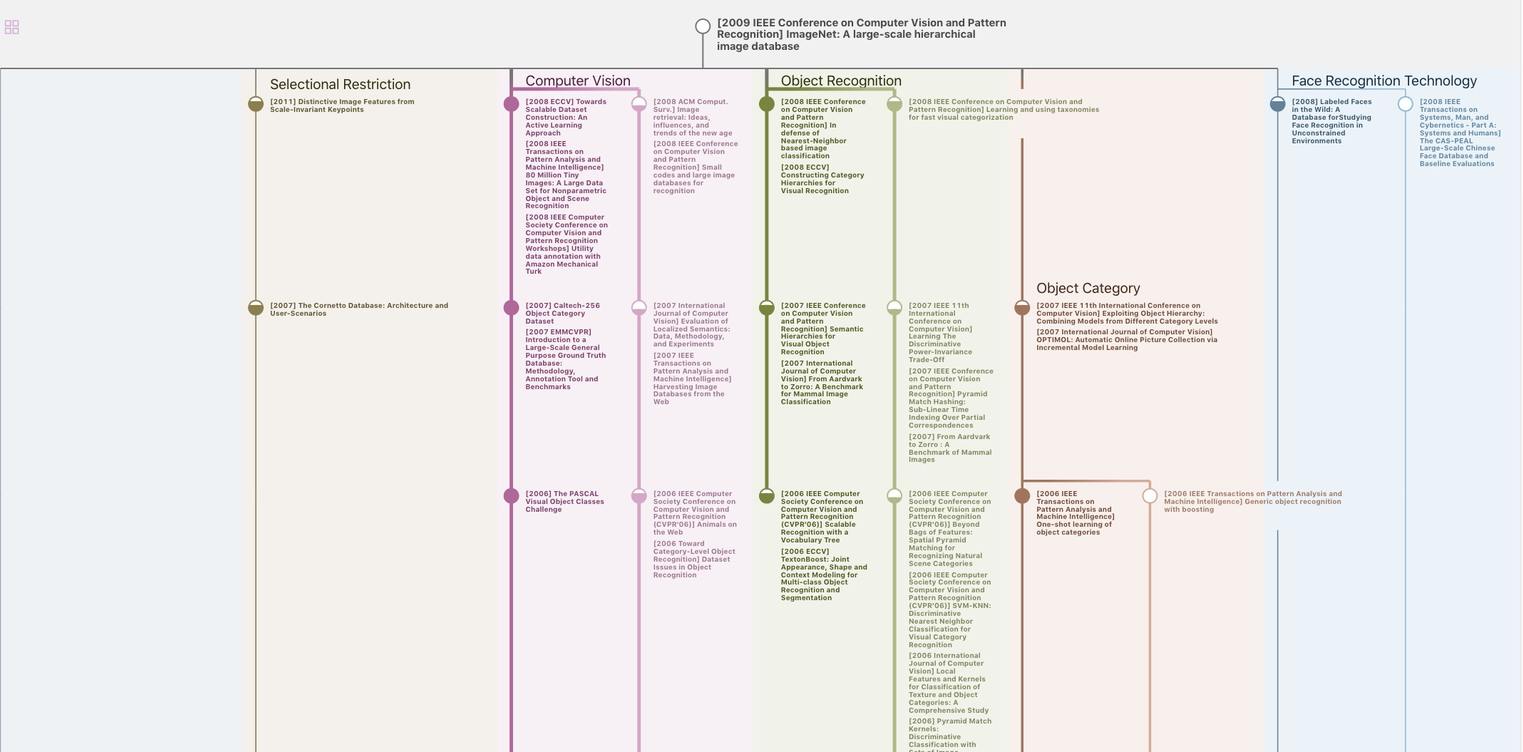
生成溯源树,研究论文发展脉络
Chat Paper
正在生成论文摘要