Evaluating the accuracy of predicted bridge condition using machine learning: the role of condition history
STRUCTURE AND INFRASTRUCTURE ENGINEERING(2023)
摘要
Effective maintenance decisions for bridges depend on accurate performance prediction. Machine learning (ML) models use historical bridge performance data to learn and predict performance. However, in many agencies, the condition history of bridges is limited and does not go beyond a few years. The question, therefore, is, to what extent does condition history help us make better predictions? To address this question, a ML model was developed that analysed more than 600,000 bridge decks with 27 years of condition history. Two data selection methods were designed: non-overlapping and overlapping data. The non-overlapping data are typically used to train the model. The overlapping data introduced in this study uses the data more efficiently for model training recognising that strings of historical data convey more information. Longer term predictions were found to be positively impacted by every additional year of condition history. Short-term condition prediction (one or two years) does not need significant historical data. It was also found that overlapping data, compared to non-overlapping data, produced larger training samples and had higher prediction accuracy in the majority of experiments, but at the cost of higher running time due to a larger sample size.
更多查看译文
关键词
Artificial intelligence, big data, infrastructure asset management, machine learning, bridge structure deterioration, bridge condition, neural network, performance prediction
AI 理解论文
溯源树
样例
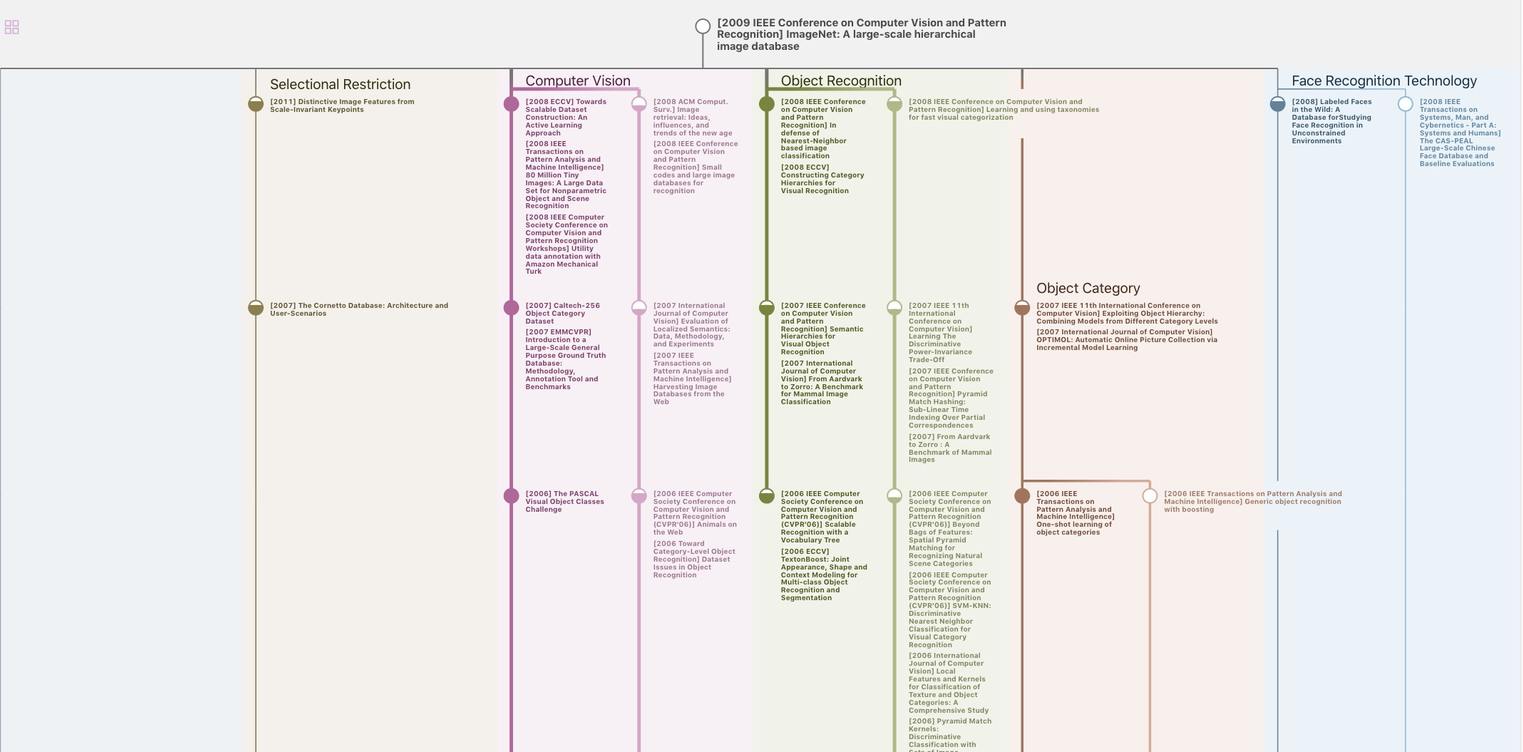
生成溯源树,研究论文发展脉络
Chat Paper
正在生成论文摘要