A Noniterative Neural Algorithm Involving Dini Derivatives for Visual Servoing of Joint-Constrained Robotic Endoscope
IEEE TRANSACTIONS ON INDUSTRIAL ELECTRONICS(2023)
摘要
In minimally invasive surgery (MIS), surgical endoscope can be automated using visual servoing by incorporating motion constraints into a time-variant quadratic programming (TVQP). Zeroing neural network (ZNN) is a superior recurrent neural network (RNN) for solving TVQP in real time. However, ZNNs cannot handle bound constraints in a TVQP directly and require differentiable elements, meaning that visual servoing requires the accelerations of feature points. Unfortunately, accelerations usually cannot be accurately retrieved. Motivated by the expected low accelerations in MIS, this article proposes a novel noniterative algorithm termed Dini-RNN to solve TVQP by introducing a Dini derivative operator. Unlike the existing ZNNs, the Dini-RNN can handle bound constraints directly and allows partial elements to be continuous but not differentiable everywhere. The convergence of the Dini-RNN is theoretically analyzed and proved. Subsequently, simulative and experimental results show that the Dini-RNN solution is effective to achieve visual servoing with superior performance.
更多查看译文
关键词
Dini derivative,quadratic programming (QP),recurrent neural network (RNN),robotic endoscope,visual servoing
AI 理解论文
溯源树
样例
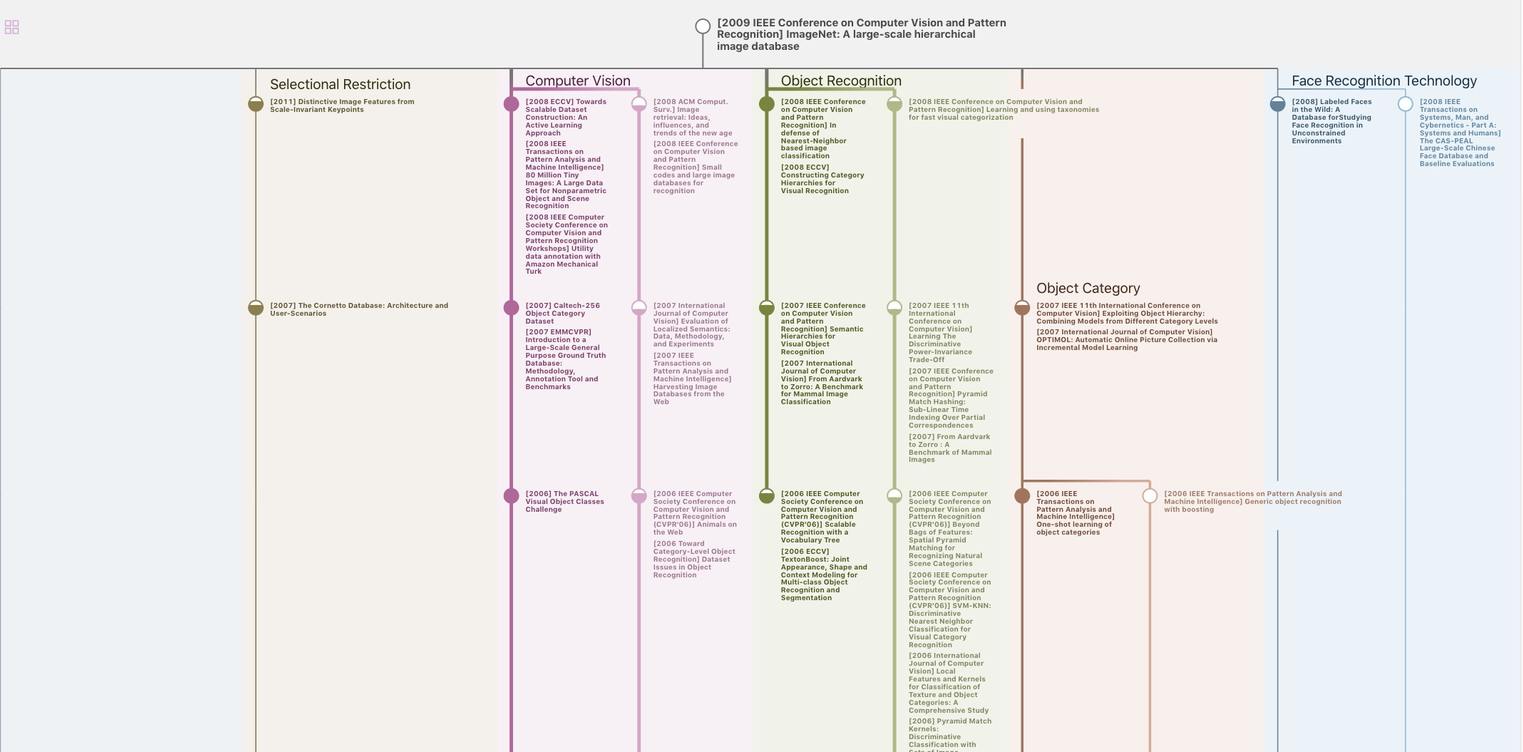
生成溯源树,研究论文发展脉络
Chat Paper
正在生成论文摘要