Driving style-aware energy management for battery/supercapacitor electric vehicles using deep reinforcement learning
JOURNAL OF ENERGY STORAGE(2023)
摘要
Driving style can significantly affect the energy consumption, battery lifespan, and driving economy of electric vehicles. In this context, this paper proposes a novel driving style-aware energy management strategy for electric vehicles with battery/supercapacitor hybrid energy storage systems based on deep reinforcement learning. Firstly, a semi-supervised support vector machine-based driving style recognition method is presented to recognize the driving style, where twenty features are extracted from limited labeled velocity/acceleration data and then reduced to six dimensions by locally linear embedding. The six dimension features are used to obtain accurate recognition results. Then a proximal policy optimization-based energy management strategy is proposed with the driving style as an additional input state, to optimize the power allocation and minimize the battery capacity loss cost. Extensive results illustrate the effectiveness of the proposed methods, e.g., the proposed driving style recognition method can recognize the real-time driving style with an accuracy of over 95%. Taking the recognized style as input, the proposed driving style-aware energy management strategy can reduce the battery capacity loss cost by 3.30–4.19% and 1.77–8.15%, compared with no driving style and incorrect driving style input energy management methods, respectively.
更多查看译文
关键词
Deep reinforcement learning,Proximal policy optimization,Driving style,S3VM,Energy management,Hybrid energy storage system
AI 理解论文
溯源树
样例
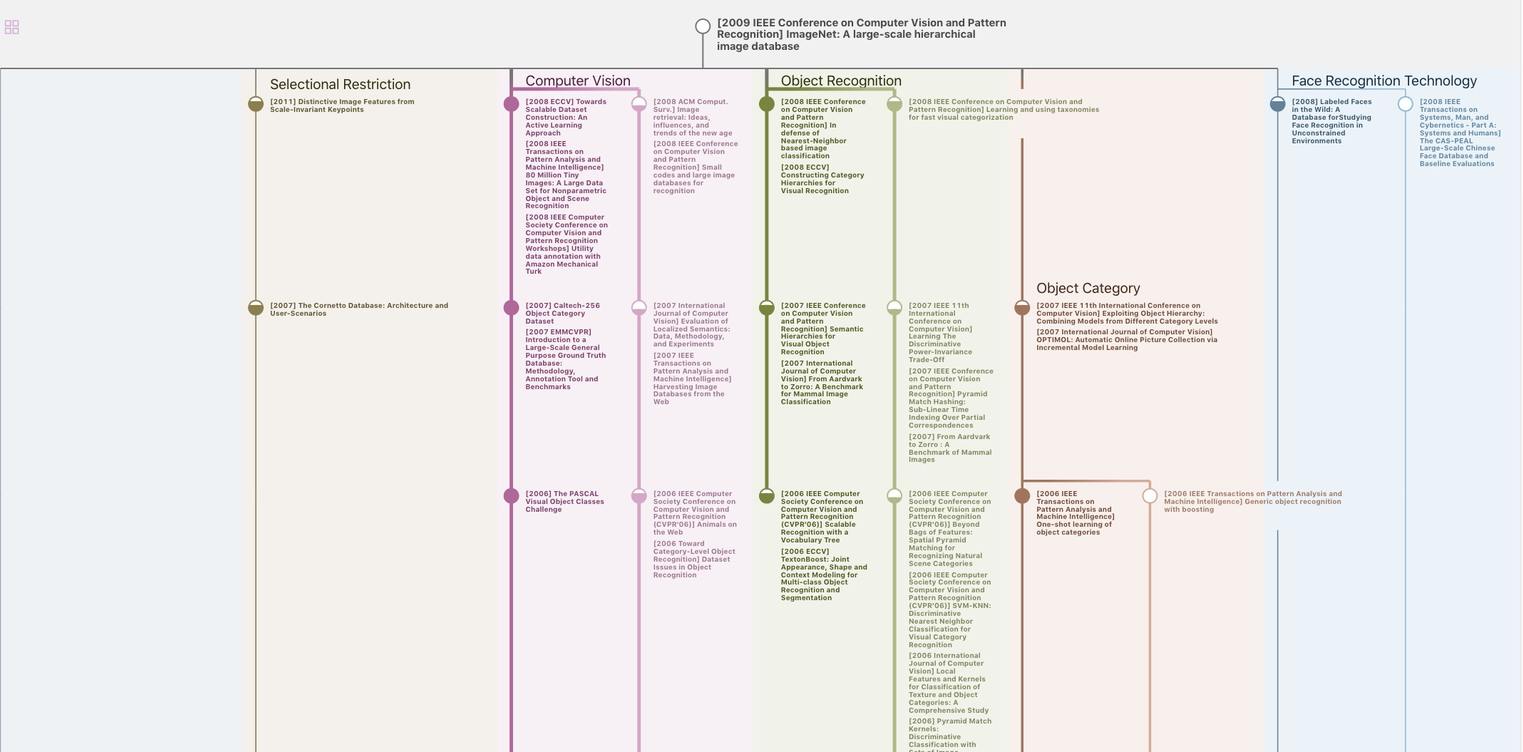
生成溯源树,研究论文发展脉络
Chat Paper
正在生成论文摘要