A Data-Driven Approach to Generating Stochastic Mesoscale 3D Shale Volume Elements From 2D SEM Images and Predicting the Equivalent Modulus
INTERNATIONAL JOURNAL OF APPLIED MECHANICS(2023)
摘要
Research on the mechanical properties of shale has contributed to the success of shale exploitation. These studies have revealed a strong correlation between the complex mesoscale structure of shale, its pronounced heterogeneity, and the varying equivalent modulus. However, conventional numerical methods face efficiency challenges in investigating the equivalent modulus of mesoscale three-dimensional (3D) shale samples. This research proposes a data-driven workflow for stochastic generation and equivalent modulus prediction of 3D shale volume elements, utilizing a limited set of two-dimensional (2D) SEM images from shale samples. First, 3D volume elements of mesoscale shale, which maintain the distribution characteristics of the mineral constituents observed in the 2D samples, are generated based only on the 2D SEM images using SliceGAN. Second, a dataset comprising the 3D mesoscale shale volume elements and their corresponding equivalent moduli is constructed using the finite element method. Then, a prediction model based on ResNet-18 is developed to predict the equivalent moduli of the shale volume elements. The proposed workflow provides a practical method for generating stochastic 3D samples and efficiently evaluating their mechanical properties. Furthermore, it fosters a better understanding of the behavior of mesoscale shale and paves the way for exploring similar applications in materials with complex mesoscale components.
更多查看译文
关键词
3d shale volume elements,2d sem images,stochastic mesoscale,data-driven
AI 理解论文
溯源树
样例
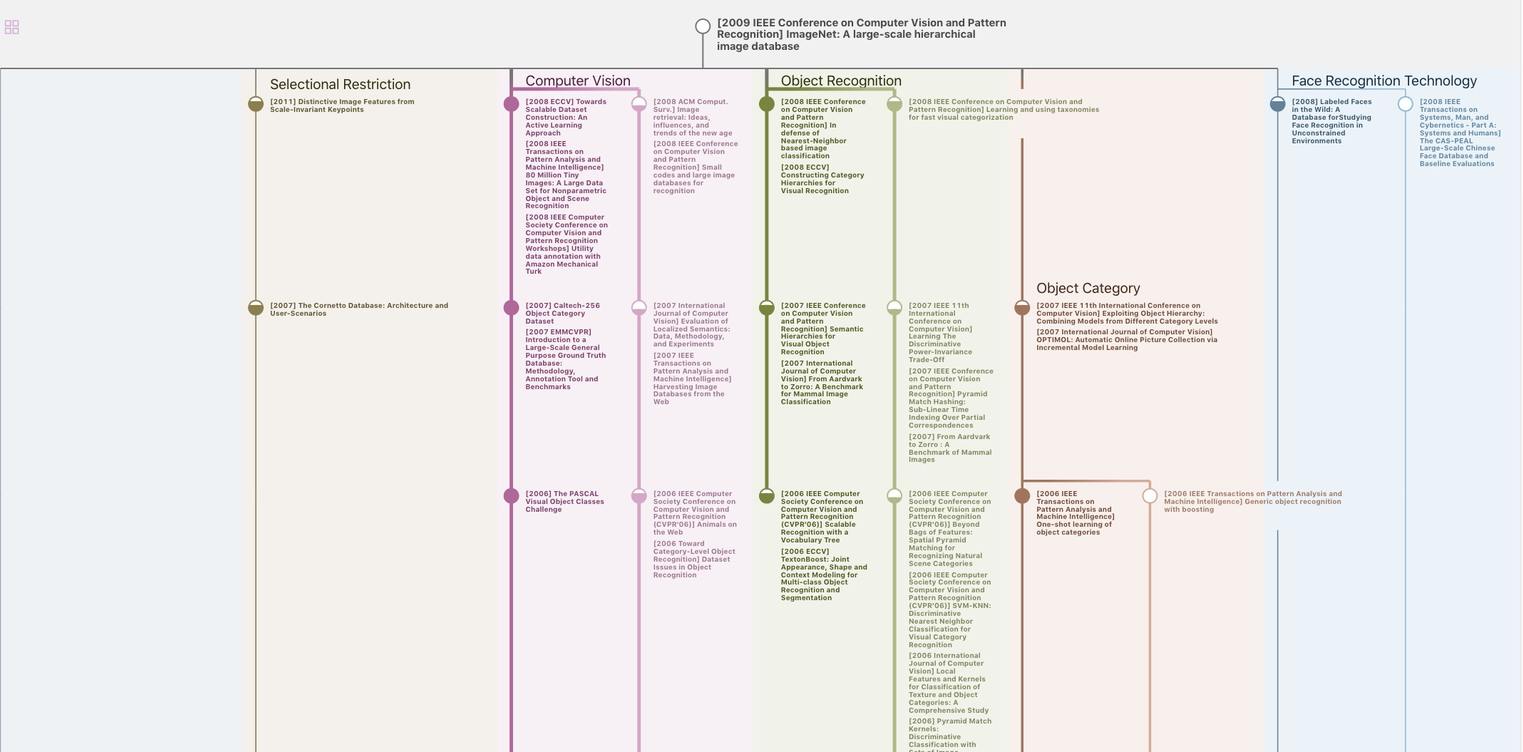
生成溯源树,研究论文发展脉络
Chat Paper
正在生成论文摘要