Advanced Meteorological Hazard Defense Capability Assessment: Addressing Sample Imbalance with Deep Learning Approaches
APPLIED SCIENCES-BASEL(2023)
摘要
With the rise in meteorological disasters, improving evaluation strategies for disaster response agencies is critical. This shift from expert scoring to data-driven approaches is challenged by sample imbalance in the data, affecting accurate capability assessments. This study proposes a solution integrating adaptive focal loss into the cross-entropy loss function to address sample distribution imbalances, facilitating nuanced evaluations. A key aspect of this solution is the Encoder-Adaptive-Focal deep learning model coupled with a custom training algorithm, adept at handling the data complexities of meteorological disaster response agencies. The model proficiently extracts and optimizes capability features from time series data, directing the evaluative focus toward more complex samples, thus mitigating sample imbalance issues. Comparative analysis with existing methods like UAE-NaiveBayes, UAE-SVM, and UAE-RandomForest illustrates the superior performance of our model in ability evaluation, positioning it as a robust tool for dynamic capability evaluation. This work aims to enhance disaster management strategies, contributing to mitigating the impacts of meteorological disasters.
更多查看译文
关键词
meteorological hazard defense,sample imbalance,loss function,classification
AI 理解论文
溯源树
样例
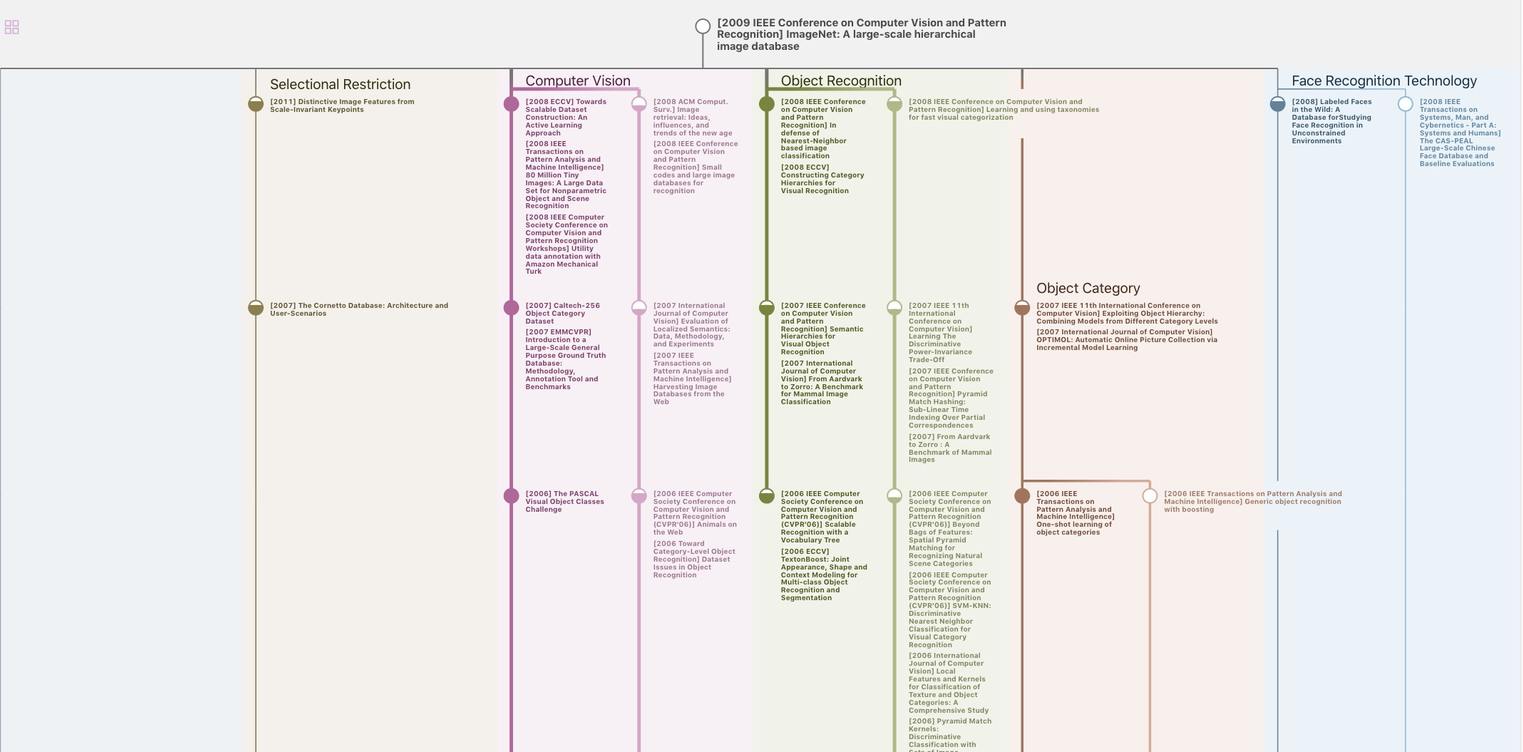
生成溯源树,研究论文发展脉络
Chat Paper
正在生成论文摘要