Identifying Genetic Mutation Status in Patients with Colorectal Cancer Liver Metastases Using Radiomics-Based Machine-Learning Models
CANCERS(2023)
摘要
Simple Summary For patients with colorectal cancer with liver metastases, it is important to determine the genetic mutations (e.g., KRAS mutations) of the liver metastases. Around 35-45% of patients with colorectal cancer liver metastases (CRLM) have a KRAS mutation, and genetic mutations are used in treatment planning and prognostication. The aim of this study was to assess if KRAS mutations could be identified on CT scans using radiomics. In the discovery cohort of 255 patients, KRAS mutations could be identified with a good accuracy. In the external validation cohort consisting of 129 patients, the radiomics model performed poorly. These results indicate that radiomics might be used to determine genetic mutations such as KRAS, but foremost emphasize the importance of the external validation of radiomics models. External validation is crucial for the assessment of clinical applicability and should be mandatory in all future studies in the field of radiomics.Abstract For patients with colorectal cancer liver metastases (CRLM), the genetic mutation status is important in treatment selection and prognostication for survival outcomes. This study aims to investigate the relationship between radiomics imaging features and the genetic mutation status (KRAS mutation versus no mutation) in a large multicenter dataset of patients with CRLM and validate these findings in an external dataset. Patients with initially unresectable CRLM treated with systemic therapy of the randomized controlled CAIRO5 trial (NCT02162563) were included. All CRLM were semi-automatically segmented in pre-treatment CT scans and radiomics features were calculated from these segmentations. Additionally, data from the Netherlands Cancer Institute (NKI) were used for external validation. A total of 255 patients from the CAIRO5 trial were included. Random Forest, Gradient Boosting, Gradient Boosting + LightGBM, and Ensemble machine-learning classifiers showed AUC scores of 0.77 (95%CI 0.62-0.92), 0.77 (95%CI 0.64-0.90), 0.72 (95%CI 0.57-0.87), and 0.86 (95%CI 0.76-0.95) in the internal test set. Validation of the models on the external dataset with 129 patients resulted in AUC scores of 0.47-0.56. Machine-learning models incorporating CT imaging features could identify the genetic mutation status in patients with CRLM with a good accuracy in the internal test set. However, in the external validation set, the models performed poorly. External validation of machine-learning models is crucial for the assessment of clinical applicability and should be mandatory in all future studies in the field of radiomics.
更多查看译文
关键词
colorectal cancer,liver metastases,radiomics,CT scan,genetic mutation,KRAS mutation
AI 理解论文
溯源树
样例
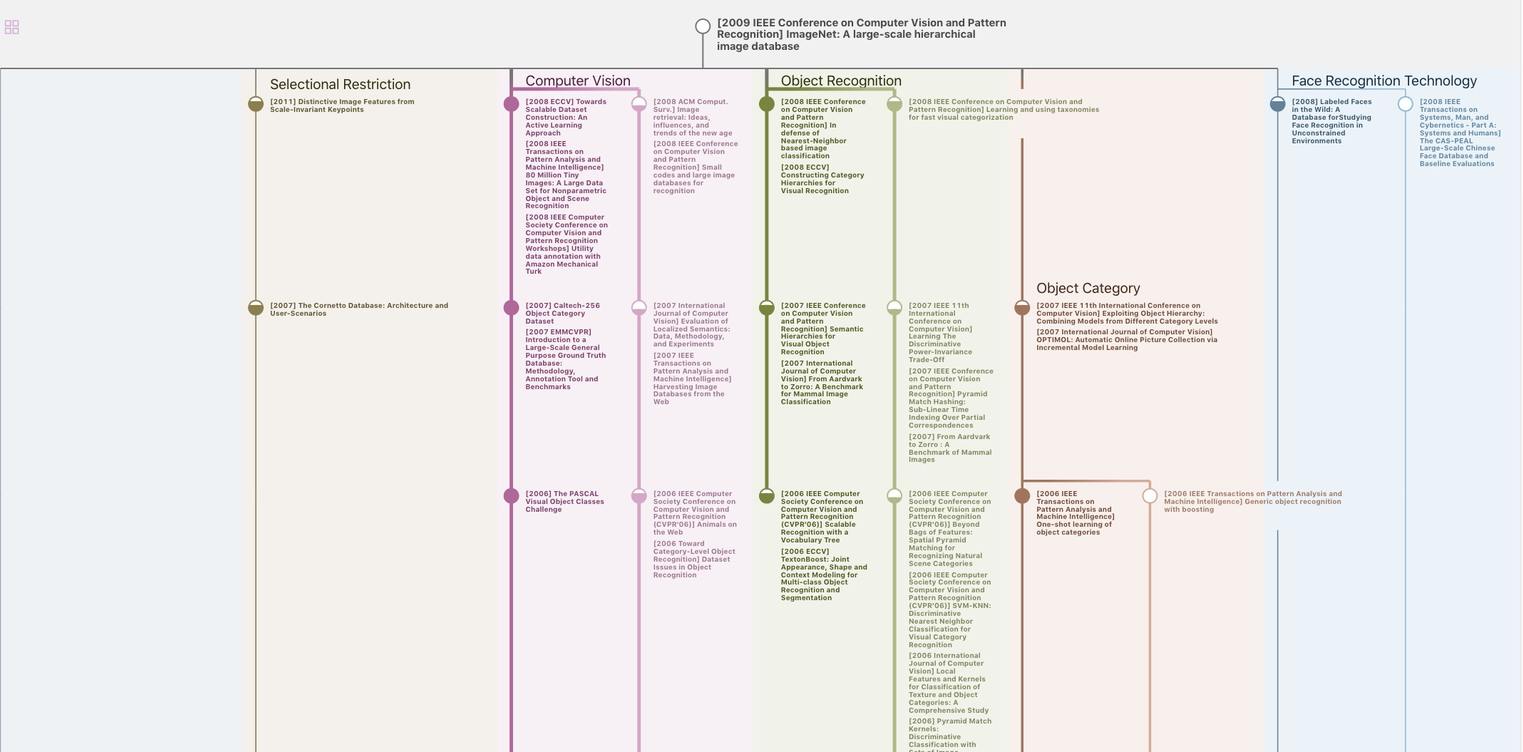
生成溯源树,研究论文发展脉络
Chat Paper
正在生成论文摘要