Object Detection Based on an Improved YOLOv7 Model for Unmanned Aerial-Vehicle Patrol Tasks in Controlled Areas
ELECTRONICS(2023)
摘要
When working with objects on a smaller scale, higher detection accuracy and faster detection speed are desirable features. Researchers aim to endow drones with these attributes in order to improve performance when patrolling in controlled areas for object detection. In this paper, we propose an improved YOLOv7 model. By incorporating the variability attention module into the backbone network of the original model, the association between distant pixels is increased, resulting in more effective feature extraction and, thus, improved model detection accuracy. By improving the original network model with deformable convolution modules and depthwise separable convolution modules, the model enhances the semantic information extraction of small objects and reduces the number of model parameters to a certain extent. Pretraining and fine-tuning techniques are used for training, and the model is retrained on the VisDrone2019 dataset. Using the VisDrone2019 dataset, the improved model achieves an mAP50 of 52.3% on the validation set. Through the visual comparative analysis of the detection results in our validation set, we find that the model shows a significant improvement in detecting small objects compared with previous iterations.
更多查看译文
关键词
drone patrol,control area,object detection,deformable attention,deformable convolution,depthwise separable convolution,YOLOv7
AI 理解论文
溯源树
样例
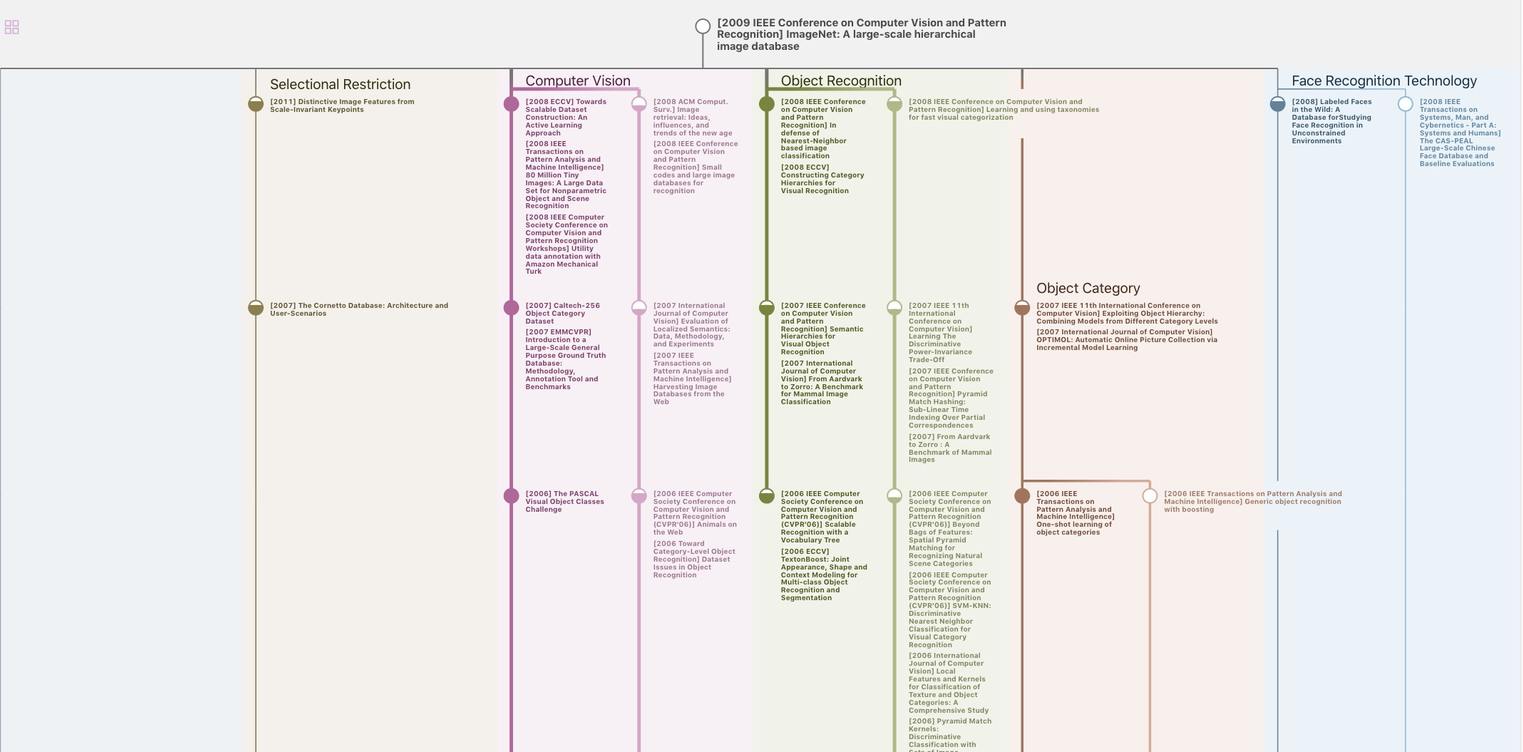
生成溯源树,研究论文发展脉络
Chat Paper
正在生成论文摘要