A Comparative Study of Landslide Susceptibility Mapping Using Bagging PU Learning in Class-Prior Probability Shift Datasets
REMOTE SENSING(2023)
摘要
Landslide susceptibility mapping is typically based on binary prediction probabilities. However, non-landslide samples in modeling datasets are often unlabeled data, and the phenomenon of class-priori shift, that is, the proportion of landslide samples frequently deviates from real-world scenarios and is spatially heterogeneous. By comparing the classification performance and predicted probability distributions across multiple unbalanced datasets with known and unknown sample proportions, this study assesses the landslide susceptibility model's generalization ability in the context of class-prior shifts. The study investigates the potential of Bagging PU Learning, a semi-supervised learning approach, in improving the generalization performance of landslide susceptibility models and proposes the Bagging PU-GDBT algorithm. Our findings highlight the effectiveness of Bagging PU Learning in enhancing the recall of landslides and the generalization capabilities of models on unbalanced datasets. This method reduces prediction uncertainties, especially in high and very high susceptibility zones. Furthermore, results emphasize the superiority of models trained on balanced datasets with 1:1 sample ratio for landslide susceptibility mapping over those trained on unbalanced datasets.
更多查看译文
关键词
landslide susceptibility mapping,class-prior probability shift,generalization performance evaluation,Bagging PU learning
AI 理解论文
溯源树
样例
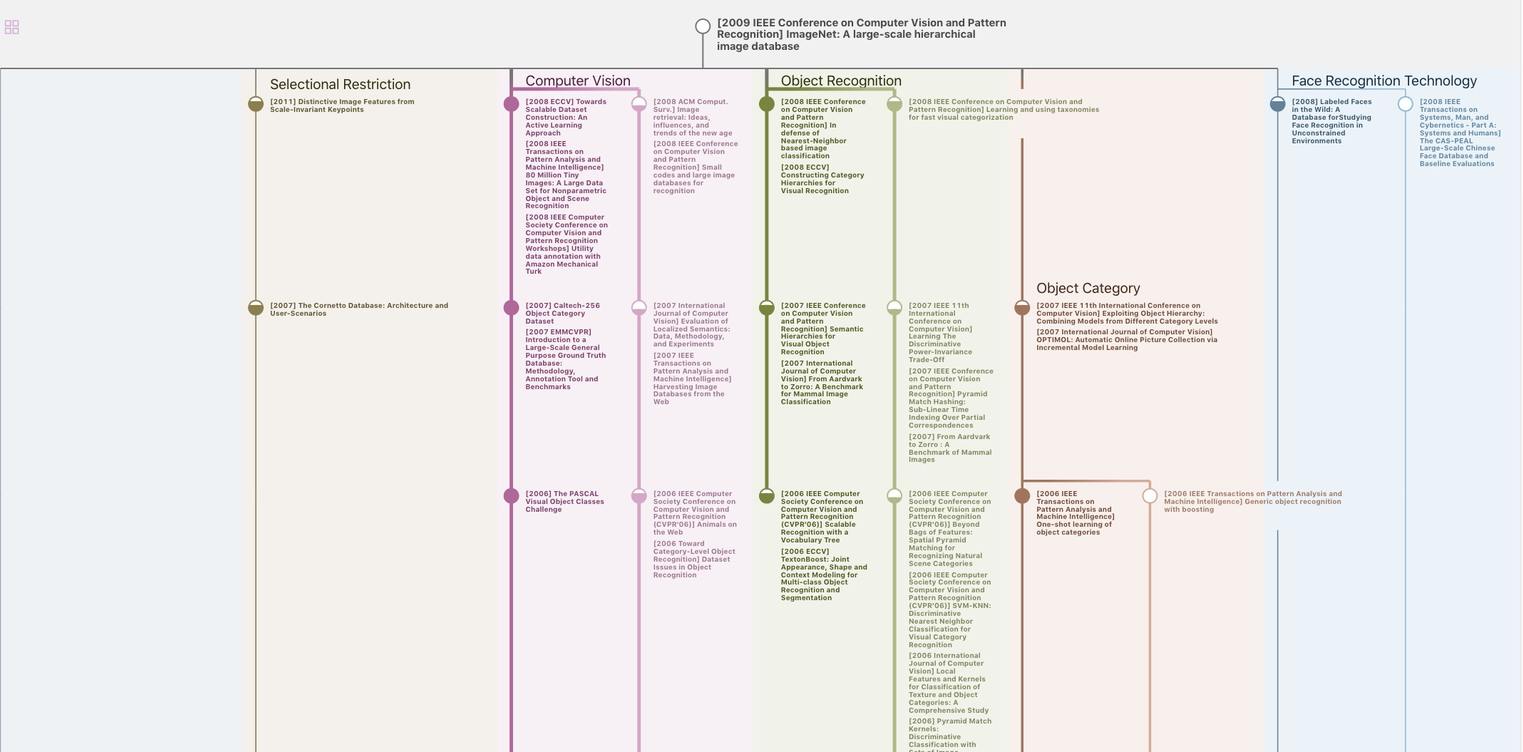
生成溯源树,研究论文发展脉络
Chat Paper
正在生成论文摘要