Predicting Generation of Different Demolition Waste Types Using Simple Artificial Neural Networks
SUSTAINABILITY(2023)
摘要
In South Korea, demolition waste (DW) management has become increasingly significant owing to the rising number of old buildings. Effective DW management requires an efficient approach that accurately quantifies and predicts the generation of DW (DWG) of various types, which necessitates access to the required information or technology capable of achieving this. Hence, we developed an artificial intelligence-based model that predicts the generation of ten DW types, specifically from buildings in redevelopment areas. We used an artificial neural network algorithm with <10 neurons in the hidden layer to derive individual input variables and optimal hyperparameters for each DW type. All DWG prediction models achieved an average validation and test prediction performance (R-2) of 0.970 and 0.952, respectively, with their ratios of percent deviation >= 2.5, verifying them as excellent models. Moreover, Shapley additive explanations analysis revealed that DWG was most impacted by the floor area for all DW types, with a positive correlation with DWG. Conversely, other factors showed either a positive or negative correlation with DWG, depending on the DW type. The study findings may assist demolition companies and local governments in making informed decisions for efficient DW management and resource allocation by accurately predicting the generation of various types of DW.
更多查看译文
关键词
waste management,demolition waste generation,machine learning,artificial neural network,SHAP analysis
AI 理解论文
溯源树
样例
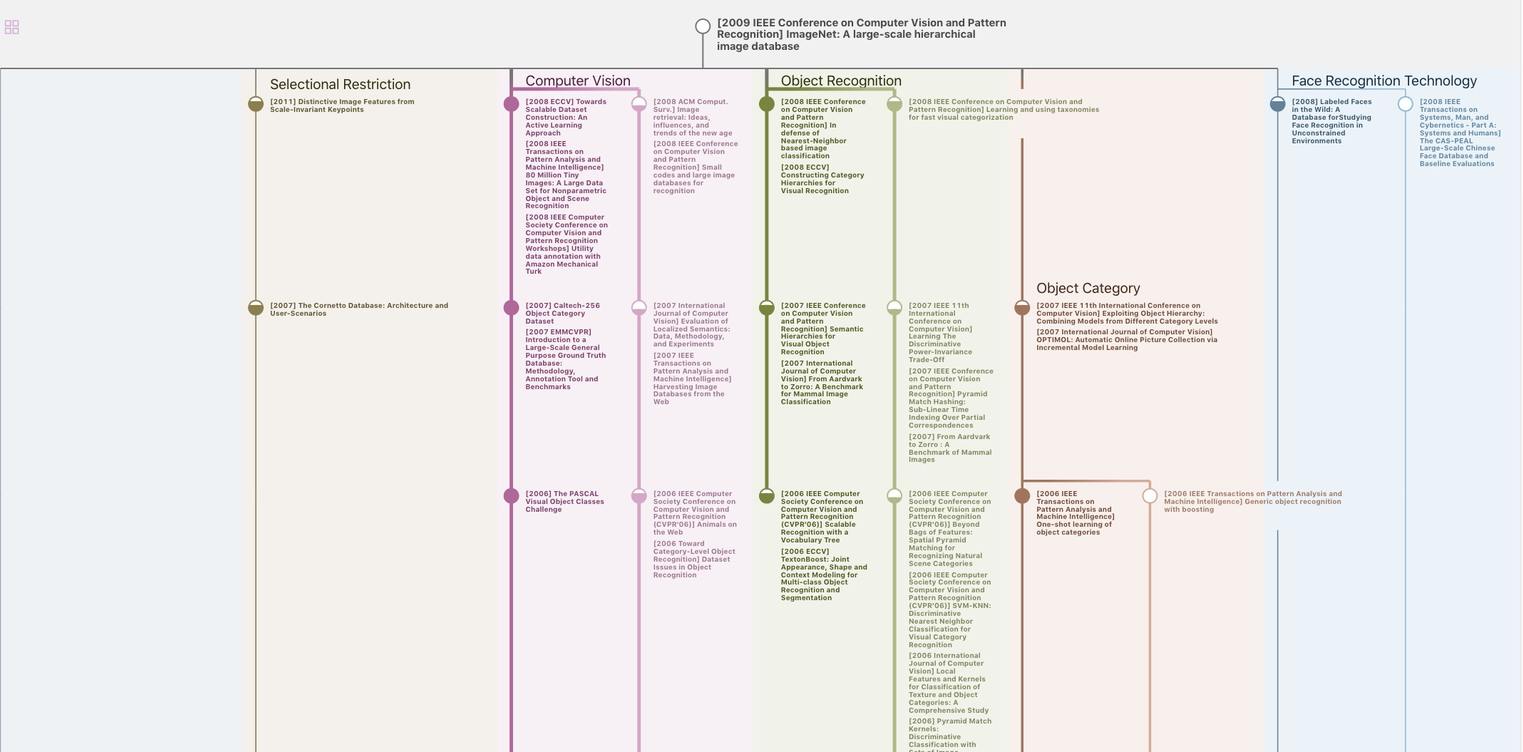
生成溯源树,研究论文发展脉络
Chat Paper
正在生成论文摘要