AG-NAS: An Attention GRU-Based Neural Architecture Search for Finger-Vein Recognition
IEEE TRANSACTIONS ON INFORMATION FORENSICS AND SECURITY(2024)
摘要
Finger-vein recognition has attracted extensive attention due to its exceptional level of security and privacy. Recently, deep neural networks (DNNs), such as convolutional neural networks (CNNs) showing robust capacity for feature representation, have been proposed for vein recognition. The architectures of these DNNs, however, have primarily been manually designed based on human prior knowledge, which is both time-consuming and error-prone. To overcome these problems, we propose AG-NAS, an Attention Gated recurrent unit-based Neural Architecture Search to automatically search for the optimal network architecture, thereby improving the recognition performance for different finger-vein recognition tasks. First, we combine the self-attention mechanism and gated recurrent unit (GRU) to propose an attention GRU module employed as a controller to generate the architectural hyperparameters of candidate neural networks automatically. Second, we investigate a parameter-sharing supernet policy to reduce the search space, computation, and time costs. Finally, we conduct rigorous experiments on our finger-vein database and two public finger-vein databases. The experimental results demonstrate that the proposed AG-NAS outperforms the representative approaches and achieves state-of-the-art recognition accuracy.
更多查看译文
关键词
Computer architecture,Task analysis,Neural networks,Veins,Convolutional neural networks,Logic gates,Fingerprint recognition,Finger-vein recognition,deep learning,neural architecture search (NAS),gated recurrent unit (GRU),self-attention
AI 理解论文
溯源树
样例
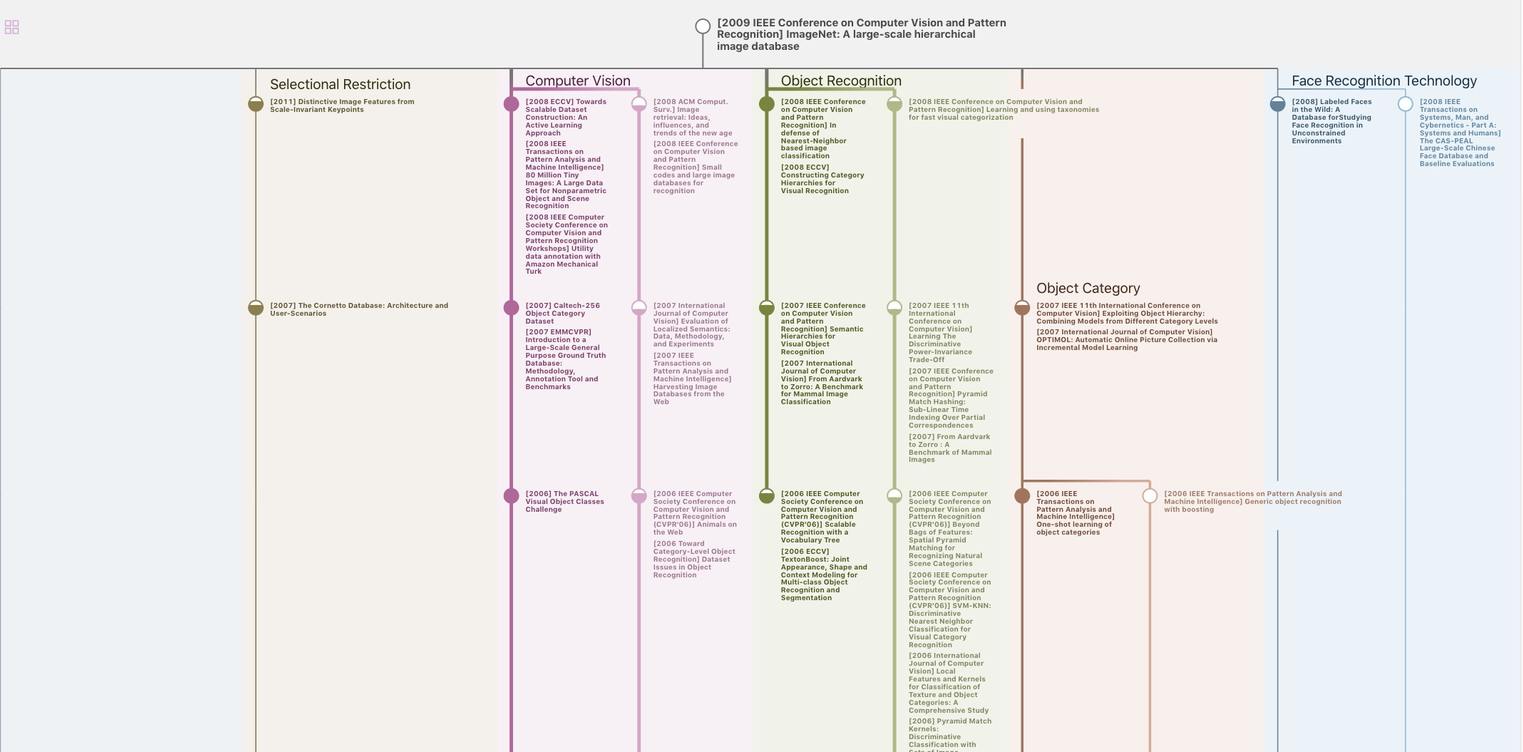
生成溯源树,研究论文发展脉络
Chat Paper
正在生成论文摘要