Identification of ICD-code misclassifications in cardiac disease using natural language processing
European Journal of Preventive Cardiology(2023)
摘要
Abstract Funding Acknowledgements Type of funding sources: Public grant(s) – National budget only. Main funding source(s): FWO - Flanders Research Foundation. Background/Introduction The international classification of disease (ICD) codes are used worldwide for classification of hospitalisations. The codes are used for administrative, financial and research purposes. It is known however that errors occur in the coding process. Purpose To investigate methods for automatic classification of disease in unstructured medical records using natural language processing (NLP) and to compare these to conventional ICD-10 coding. Methods Two datasets were used: the open-source Medical Information Mart for Intensive Care (MIMIC)-III dataset (n=40.000) for algorithm testing and a dataset from a hospital in Belgium (n = 8041). In the Belgian dataset automated pseudonymization was performed prior to further analysis. A training, validation and test set was used in all methods. Automated searches using NLP algorithms were performed for the diagnoses "atrial fibrillation" and "heart failure". The information extraction methods that were used were: rule-based search, logistic regression, term frequency-inverse document frequency (TF-IDF), XGBoost and bio-bidirectional encoder representations from transformers (BioBERT). All algorithms were tested on the MIMIC-III dataset. Precision (or positive predictive value), recall (or sensitivity) and accuracy were calculated for each model. The best performing algorithm was then deployed on the Belgian dataset. Results and discussion Results for the different NLP models are depicted in Table 1. Non-compatible results in NLP versus ICD-coding often indicated errors in ICD-coding. NLP algorithms could thus be used to improve the ICD-coding process in hospitals. Conclusion A high accuracy can be achieved when using NLP algorithms for diagnostic classification of patient records. Also, NLP algorithms can be used to identify ICD-coding errors and optimise the ICD-coding process.
更多查看译文
关键词
cardiac disease,natural language processing,icd-code
AI 理解论文
溯源树
样例
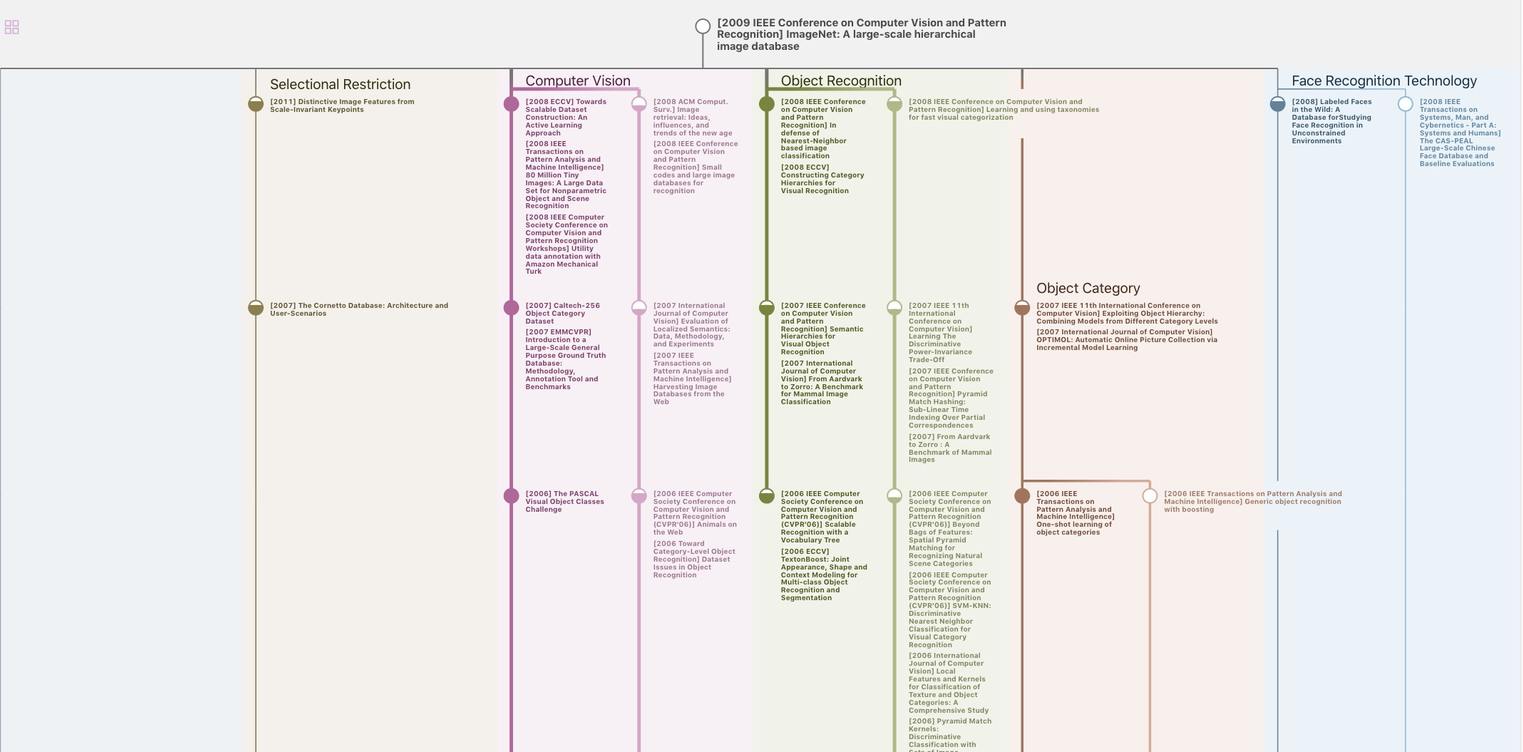
生成溯源树,研究论文发展脉络
Chat Paper
正在生成论文摘要