Online Optimization Method of Learning Process for Meta-Learning
COMPUTER JOURNAL(2023)
摘要
Meta-learning is a pivotal and potentially influential machine learning approach to solve challenging problems in reinforcement learning. However, the costly hyper-parameter tuning for training stability of meta-learning is a known shortcoming and currently a hotspot of research. This paper addresses this shortcoming by introducing an online and easily trainable hyper-parameter optimization approach, called Meta Parameters Learning via Meta-Learning (MPML), to combine online hyper-parameter adjustment scheme into meta-learning algorithm, which reduces the need to tune hyper-parameters. Specifically, a basic learning rate for each training task is put forward. Besides, the proposed algorithm dynamically adapts multiple basic learning rate and a shared meta-learning rate through conducting gradient descent alongside the initial optimization steps. In addition, the sensitivity with respect to hyper-parameter choices in the proposed approach are also discussed compared with model-agnostic meta-learning method. The experimental results on reinforcement learning problems demonstrate MPML algorithm is easy to implement and delivers more highly competitive performance than existing meta-learning methods on a diverse set of challenging control tasks.
更多查看译文
关键词
Meta-learning,deep reinforcement learning,hyper-parameter,gradient descent
AI 理解论文
溯源树
样例
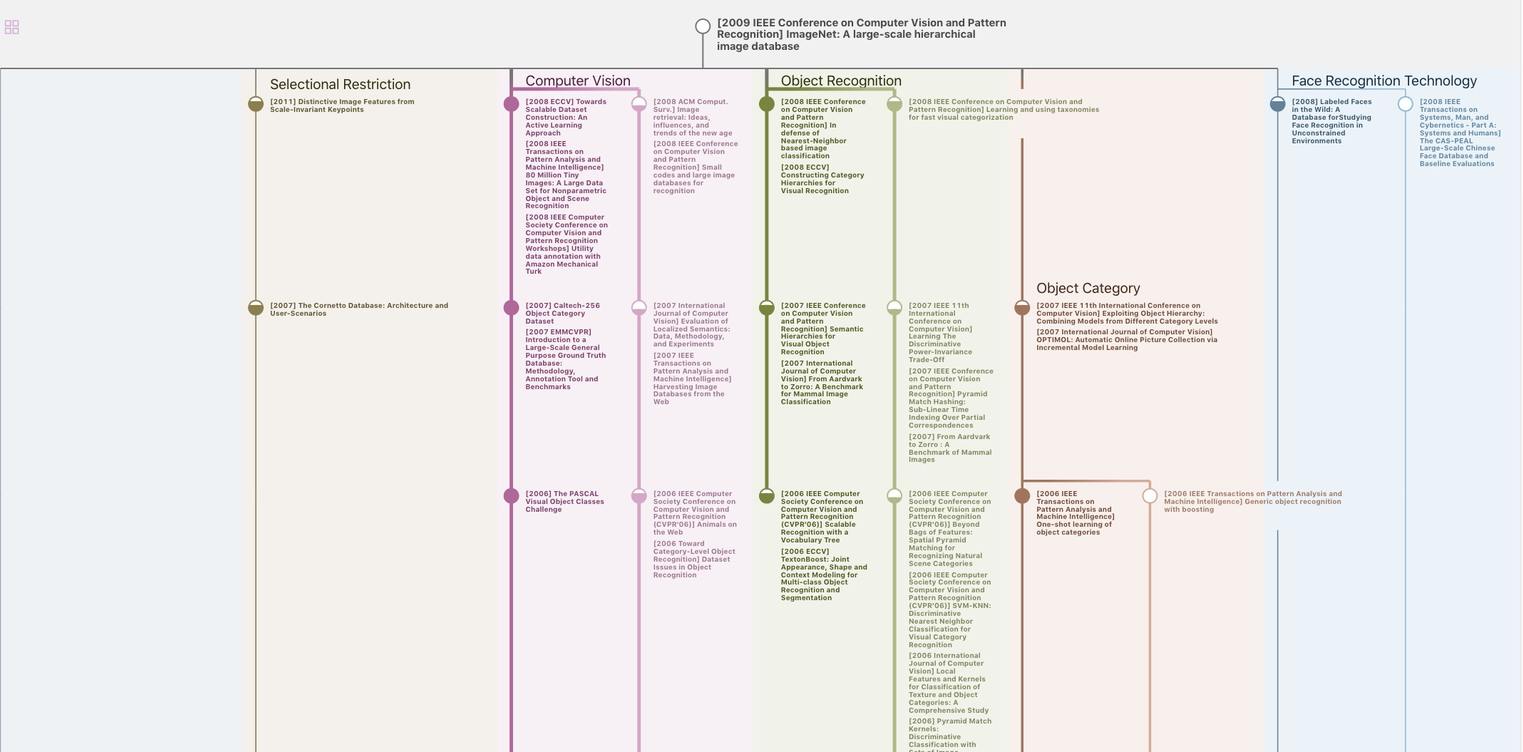
生成溯源树,研究论文发展脉络
Chat Paper
正在生成论文摘要