Deep Adaptive Quadruplet Hashing With Probability Sampling for Large-Scale Image Retrieval
IEEE TRANSACTIONS ON CIRCUITS AND SYSTEMS FOR VIDEO TECHNOLOGY(2023)
摘要
With the preferable efficiency in storage and computation, hashing has shown potential application in large-scale multimedia retrieval. Compared with traditional hashing algorithms using hand-crafted characteristics, deep hashing inherits the representational capacity of deep neural networks to jointly learn semantic features and hash functions, encoding raw data into compact binary codes with significant discrimination. Generally, most of the current multi-wise hashing methods view the similarity margins between image pairs as constant values in training process. When the distance between sample pairs exceeds the fixed margin, the hashing network would not learn anything. Besides, available hashing methods commonly introduce the random sampling strategy to build training batches and ignore the sample distribution, which is harmful to parameter optimization. In this paper, we propose a novel Deep Adaptive Quadruplet Hashing with probability sampling (DAQH) for discriminative binary code learning. Specifically, with exploring the distribution relationship of raw samples, a non-uniform probability sampling strategy is proposed to build more informative and representative training batches, while maintaining the diversity of training samples. By introducing the prior similarity of sample pairs to calculate corresponding margins, an adaptive margin quadruplet loss is designed to dynamically preserve the underlying semantic relationships with its neighbors. To tune the attributes of binary codes, by combining quadruple regularization and orthogonality optimization, binary code constraint is developed to make the learned embedding with significant discrimination. Extensive experimental results on various benchmark datasets demonstrate our proposed DAQH framework achieves state-of-the-art visual similarity search performance.
更多查看译文
关键词
Multimedia retrieval,deep hashing,adaptive margin,probability sampling,semantic relationships,orthogonality optimization
AI 理解论文
溯源树
样例
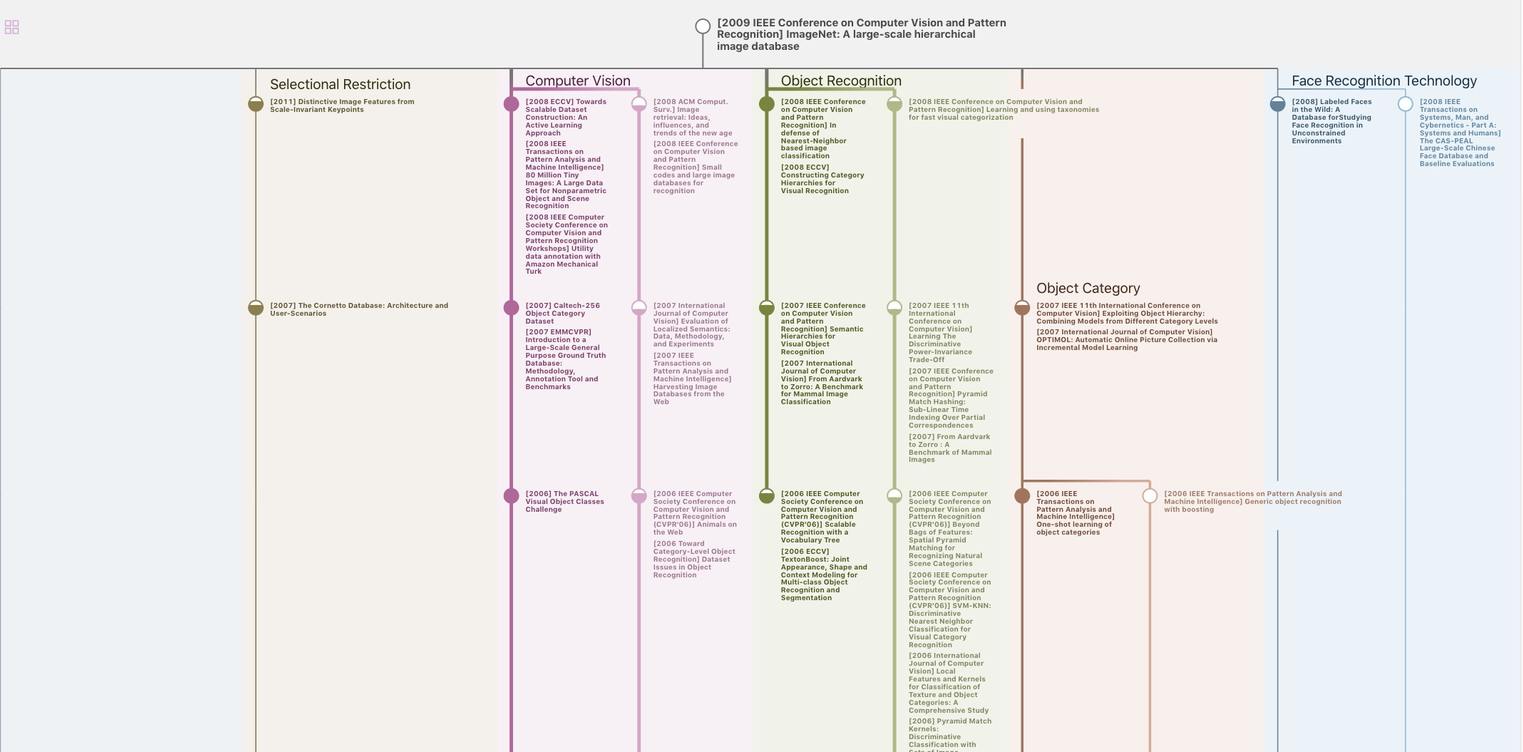
生成溯源树,研究论文发展脉络
Chat Paper
正在生成论文摘要