A Federated Learning and Deep Q-Network-Based Cooperative Resource Allocation Algorithm for Multi-Level Services in Mobile-Edge Computing Networks
IEEE TRANSACTIONS ON COGNITIVE COMMUNICATIONS AND NETWORKING(2023)
摘要
This paper studies the cooperative resource allocation problem for multi-level services in mobile edge Computing (MEC) networks. The problem is formulated as an optimization problem with the objective of maximizing the successful processing ratio of users' service requests while meeting users' service delay requirements. To solve the formulated problem, we propose a federated learning and deep Q-network (DQN) based cooperative resource allocation (FD-CRA) algorithm. The proposed FD-CRA algorithm uses a couple of DQNs at each edge node to perform spectrum resource pre-allocation, and offloading node selection and computing resource pre-allocation, respectively, for each user's service request. Meanwhile, it uses horizontal federated learning as the basic framework to consistently update all DQNs at each edge node. Simulation results show that the FD-CRA algorithm can significantly improve the successful processing ratio of users' service requests while meeting users' delay requirements.
更多查看译文
关键词
Delays,Resource management,Servers,Federated learning,Virtual machining,Multi-access edge computing,Optimization,DQN,federated learning,cooperative resource allocation,mobile edge computing
AI 理解论文
溯源树
样例
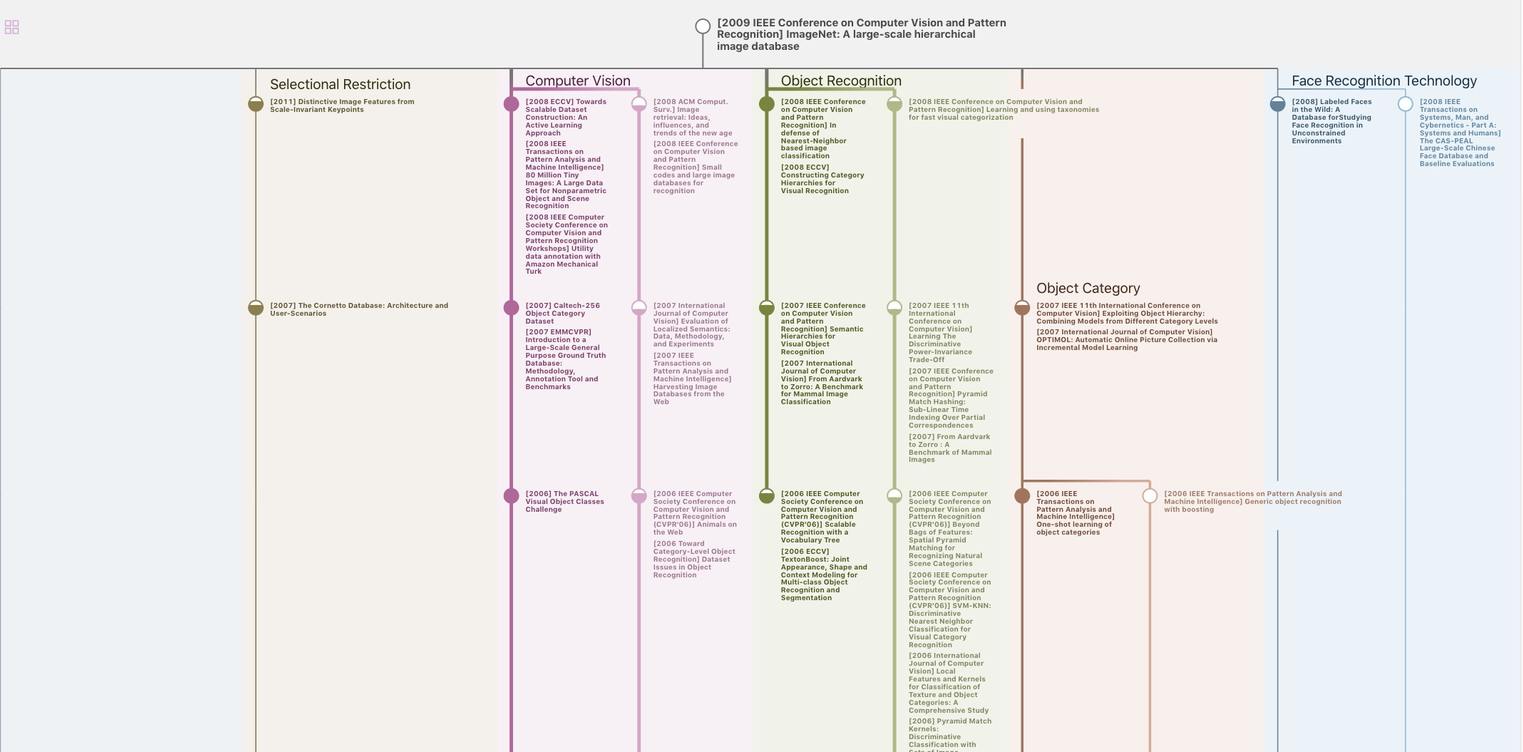
生成溯源树,研究论文发展脉络
Chat Paper
正在生成论文摘要