FuFaction: Fuzzy Factual Inconsistency Correction on Crowdsourced Documents With Hybrid-Mask at the Hidden-State Level
IEEE TRANSACTIONS ON KNOWLEDGE AND DATA ENGINEERING(2024)
摘要
Nowadays, crowdsourced documents like Wikipedia pages and comments on products are all over the Internet. However, documents generated by crowdsourcing participants may contain inconsistent facts, implicit semantics and fabricated contents, thus threatening the trustworthiness of information content security available in Internet. To address this problem, we propose FuFaction, enabled by an enhanced observation mechanism based on the notion of hybrid-mask consisting of a hard-mask and a soft-mask, to eliminate factual inconsistencies on crowdsourced documents at the hidden-state level (or in a fuzzy way), according to the given evidence retrieved from an external open domain. Specifically, instead of focusing on a specific category of factual inconsistency, FuFaction captures anomalous hidden-states between a crowdsourced document and evidence obtained via a reverse-attention mechanism, where a hard-mask controls the attending direction as bidirectional and unidirectional for better understanding on semantics. Then, a soft-mask is generated with the help of the hard-masked reverse-attention to revise or mask anomalous hidden-states on the crowdsourced document. Afterwards, the masked hidden-states are further refined by a cross reverse-attention and factual consistency reinforcement strategy, based on which a new crowdsourced document with higher factual consistency is generated via neural text generation. According to our experimental results, FuFaction can effectively deal with the fuzzy factual inconsistencies on crowdsourced documents, achieving the overall best performance in terms of factual consistency metrics with a little higher (yet still competitive) editing cost on literal vocabulary, so as to reflect factually consistent semantics supported by the given evidence.
更多查看译文
关键词
Crowdsourcing,Online services,Measurement,factual inconsistency,attention mechanism,neural text generation,natural language processing
AI 理解论文
溯源树
样例
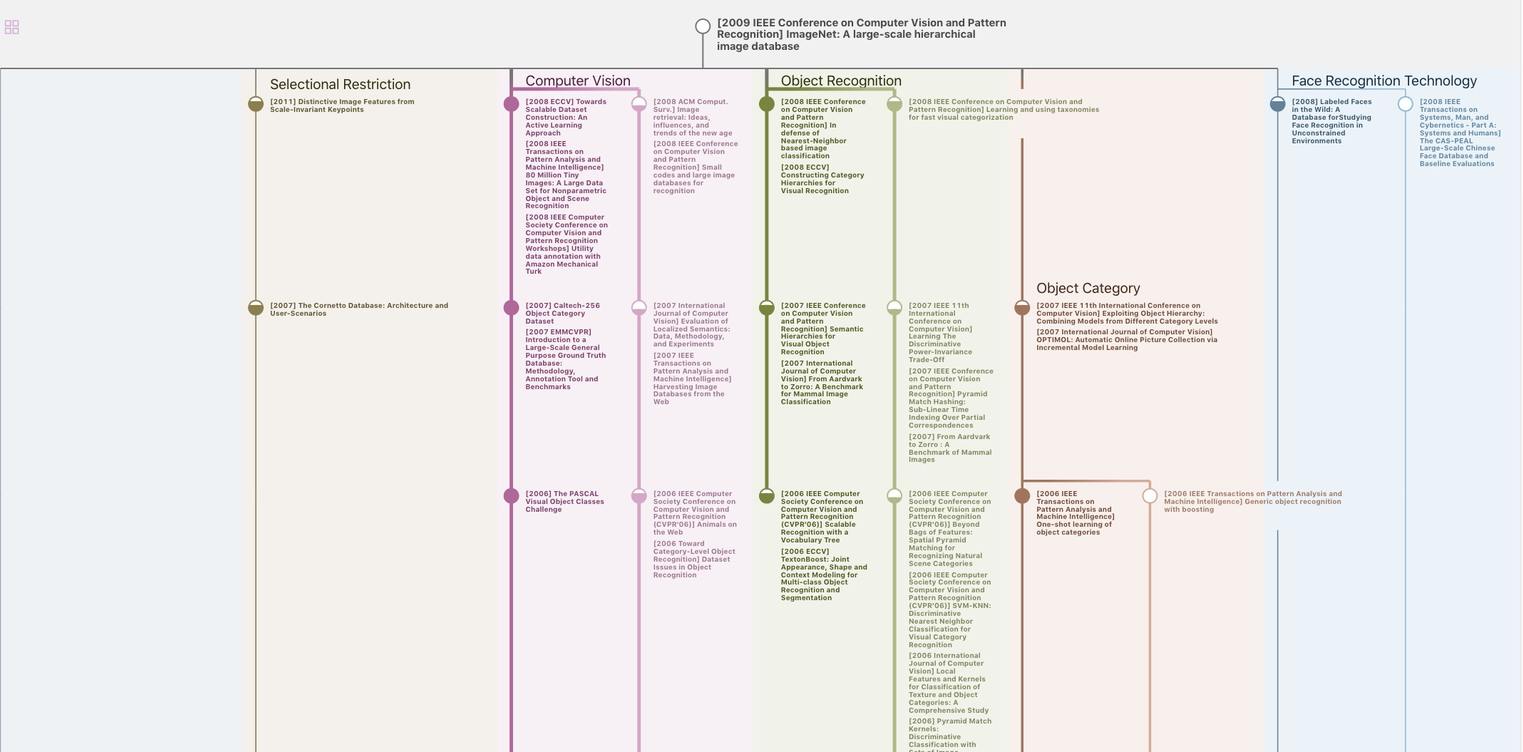
生成溯源树,研究论文发展脉络
Chat Paper
正在生成论文摘要