A multi-domain mixture density network for tool wear prediction under multiple machining conditions
INTERNATIONAL JOURNAL OF PRODUCTION RESEARCH(2023)
摘要
Accurate tool wear prediction is an essential task in machining processes because it helps to schedule efficient tool maintenance and maximise the tool's useful life, thus contributing to sustainable production via zero defect manufacturing (ZDM). However, there are limitations to existing methods; these cannot be used under multiple machining conditions, which is common practice. This problem not only hinders accurate tool wear monitoring but also necessitates the use of multiple models, which increases operation and modelling costs. Therefore, the multi-domain learning problem should be addressed to enable tool wear prediction under various machining conditions. To this end, this work presents a novel method, a multi-domain mixture density network (MD $ <^>2 $ 2N). In particular, a Bayesian learning-based feature extractor is proposed to learn domain-invariant representations. Additionally, an adversarial learning approach is developed to lead the predictive model in learning domain-invariant features. Lastly, a mixture density network-based predictor is used to generate probabilistic tool wear outputs. Experiments that use datasets from real-world milling processes under multiple conditions prove the proposed method's promising efficacy, with the best mean absolute error (MAE), root mean squared error (RMSE), and mean absolute percentage error (MAPE) of 2.1748, 5.6422, and 0.0350, respectively, indicating the ability to learn multi-domain representations.
更多查看译文
关键词
Bayesian approach,deep learning,smart manufacturing,sustainability,tool wear prediction,zero defect manufacturing
AI 理解论文
溯源树
样例
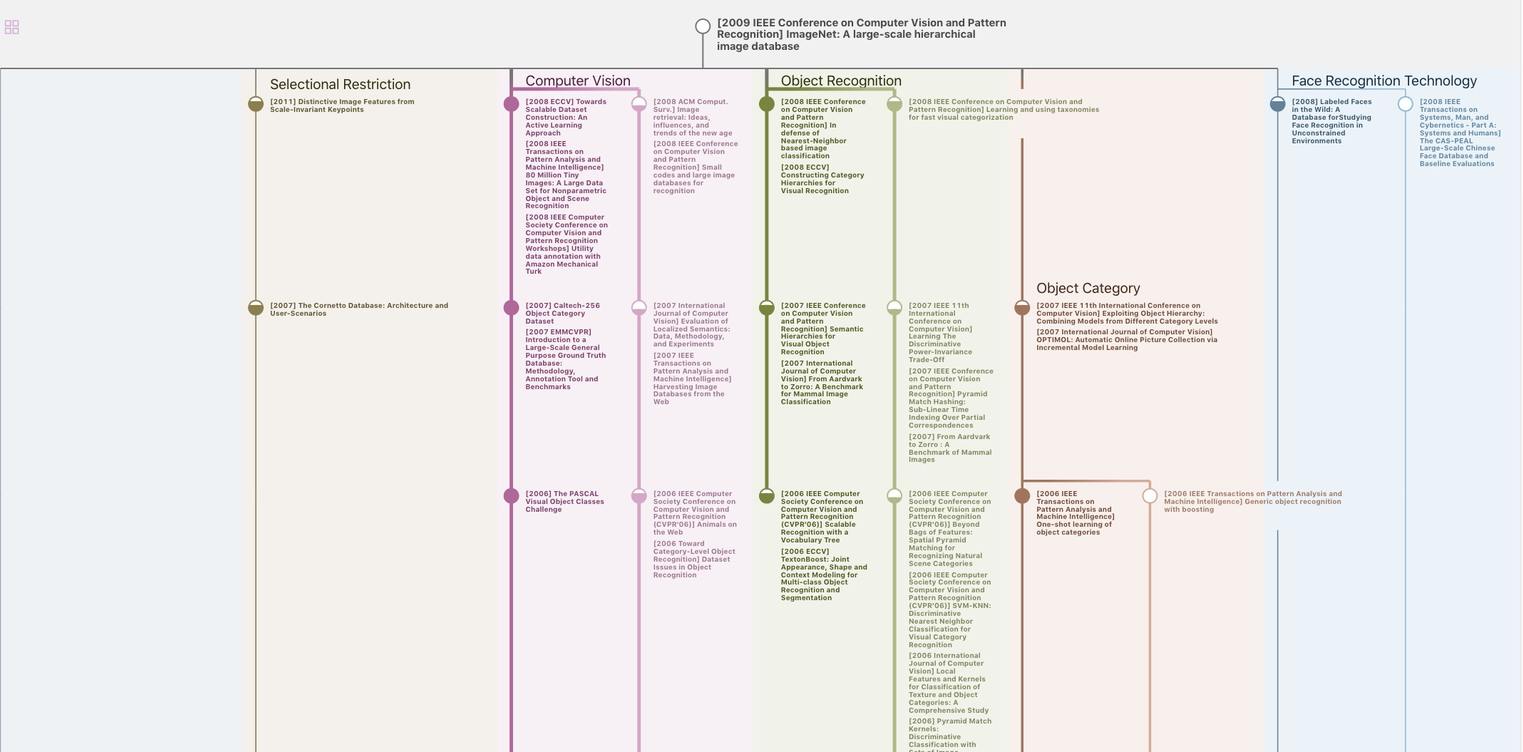
生成溯源树,研究论文发展脉络
Chat Paper
正在生成论文摘要