Landslide Inventory Mapping Based on Independent Component Analysis and UNet3+: A Case of Jiuzhaigou, China.
IEEE Journal of Selected Topics in Applied Earth Observations and Remote Sensing(2024)
摘要
Landslide inventory mapping (LIM) is an important prerequisite for disaster emergency rescue and landslide sensitivity analysis. It has been proven that convolutional neural networks have better performance for LIM than traditional machine learning methods such as support vector machines, and random forests. However, the accuracy of existing methods based only on optical images is low due to the complex landslide background. Moreover, the multiscale features of landslides are not considered in convolutional neural network methods. Therefore, this study proposes multifeatured independent component analysis UNet3+ for landslide inventory mapping based on optical images, which combines co-feature, independent component analysis (ICA), and UNet3+. First, normalized difference vegetation index (NDVI) and gray level co-occurrence matrix (GLCM) are extracted from remote sensing images acquired pre- and postearthquake event and then processed by change vector analysis. Then, ICA is implemented for NDVI, GLCM, and three elevation factors. Finally, the three principal components and the postevent images are fed into UNet3+ to generate LIM by multiscale features and deep supervision. Finally, we validate the proposed method by using the coseismic landslide of Jiuzhaigou earthquake as an experiment. The results show that the performance of recall, F1-score and mIoU are 0.13, 0.22, and 0.11 higher than those of the postevent-only images, respectively, indicating that the proposed method can effectively solve the problems of landslide identification in terms of multiscale features and complex background.
更多查看译文
关键词
Deep learning,independent component analysis,landslide inventory mapping,remote sensing,UNet3+
AI 理解论文
溯源树
样例
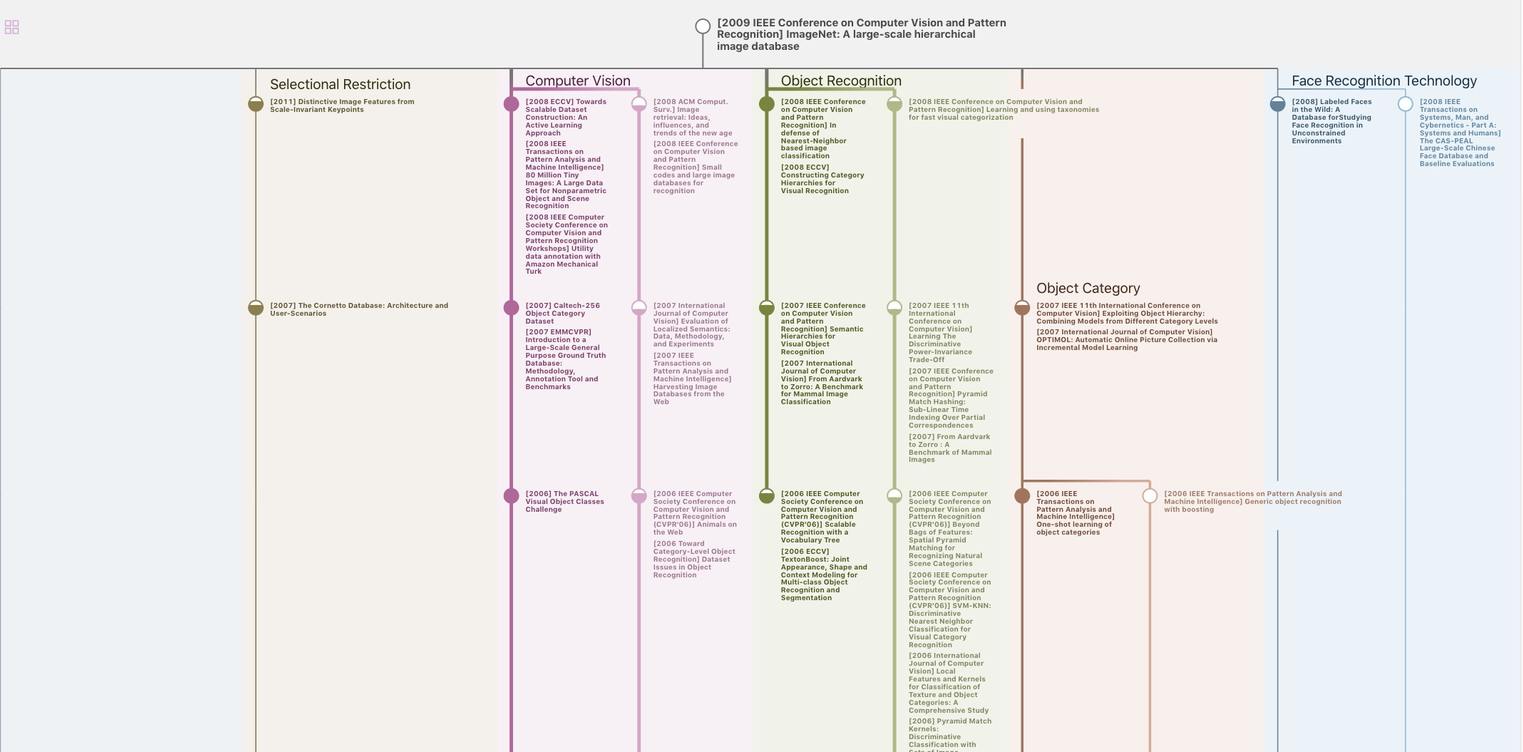
生成溯源树,研究论文发展脉络
Chat Paper
正在生成论文摘要