A Survey on Neural Data-to-Text Generation
IEEE TRANSACTIONS ON KNOWLEDGE AND DATA ENGINEERING(2024)
摘要
Data-to-text Generation (D2T) aims to generate textual natural language statements that can fluently and precisely describe the structured data such as graphs, tables, and meaning representations (MRs) in the form of key-value pairs. It is a typical and crucial task in natural language generation (NLG). Early D2T systems generated texts with the cost of human engineering in designing domain specific rules and templates, and achieved acceptable performance in coherence, fluency, and fidelity. In recent years, the data-driven D2T systems based on deep learning have reached state-of-the-art (SOTA) performance in more challenging datasets. In this paper, we provide a comprehensive review on existing neural data-to-text generation approaches. We first introduce available D2T resources, including systematically categorized D2T datasets and mainstream evaluation metrics. Next, we survey existing works based on the taxonomy along two axes: neural end-to-end D2T and neural modular D2T. We also discuss the potential applications and the adverse impacts. Finally, we present readers with the challenges faced by neural D2T and outline some potential future directions in this area.
更多查看译文
关键词
Online services,Internet,Encyclopedias,Task analysis,Natural languages,Sports,Surveys,Natural language processing,natural language generation,data-to-text generation,survey,deep learning
AI 理解论文
溯源树
样例
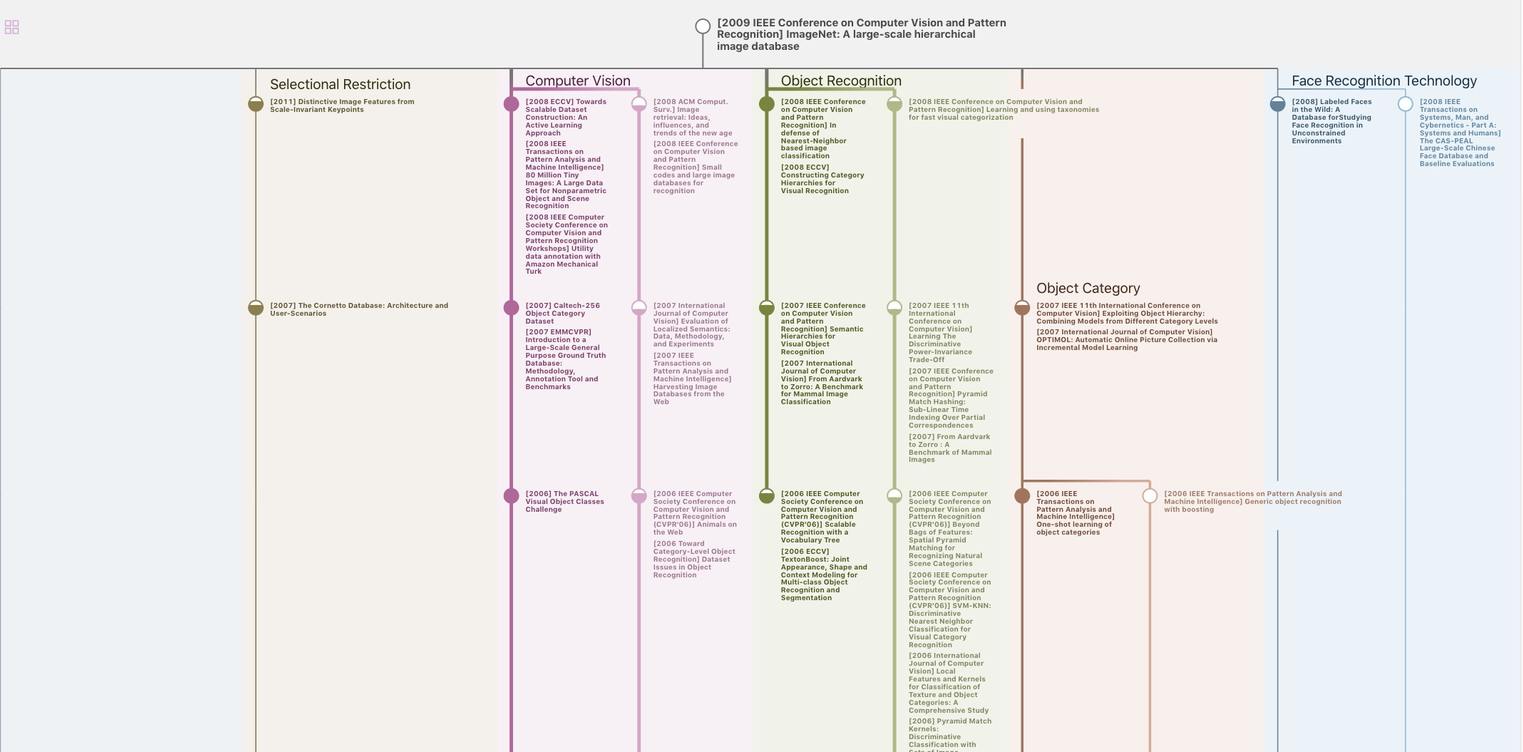
生成溯源树,研究论文发展脉络
Chat Paper
正在生成论文摘要