Boruta extra tree-bidirectional long short-term memory model development for Pan evaporation forecasting: Investigation of arid climate condition
ALEXANDRIA ENGINEERING JOURNAL(2024)
摘要
In this study, two deep learning approaches, bidirectional long short-term memory (BiLSTM) and long short-term memory (LSTM), were used along with adaptive boosting and general regression neural network to forecast multi-step-ahead pan evaporation in two arid climate stations in Iran (Ahvaz and Yazd). Lagged time series of meteorological data and pan evaporation data were input to the machine learning models. Two feature selection methods, i.e., the Boruta extra tree and XGBoost, were used to select significant inputs to reduce the number of inputs and model complexity. Different statistical metrics were used to investigate the model performance. The results demonstrated that Boruta-extra-tree-based models were more accurate than XGBoost-based models. Compared with the machine learning techniques, the combination of Boruta extra tree and BiLSTM enabled more accurate one-day-ahead forecasting of pan evaporation for both sites (Root Mean Square Error (RMSE) = 1.6857, for the Ahvaz station, and RMSE = 1.3996 for the Yazd station). The proposed model was used to forecast up to 30 days ahead of pan evaporation in both stations. The results showed that the Boruta-BiLSTM model could accurately forecast the pan evaporation for up to 30 days in both stations.
更多查看译文
关键词
Pan Evaporation,Forecasting,Machine Learning,Deep Learning,Boruta Feature Selection
AI 理解论文
溯源树
样例
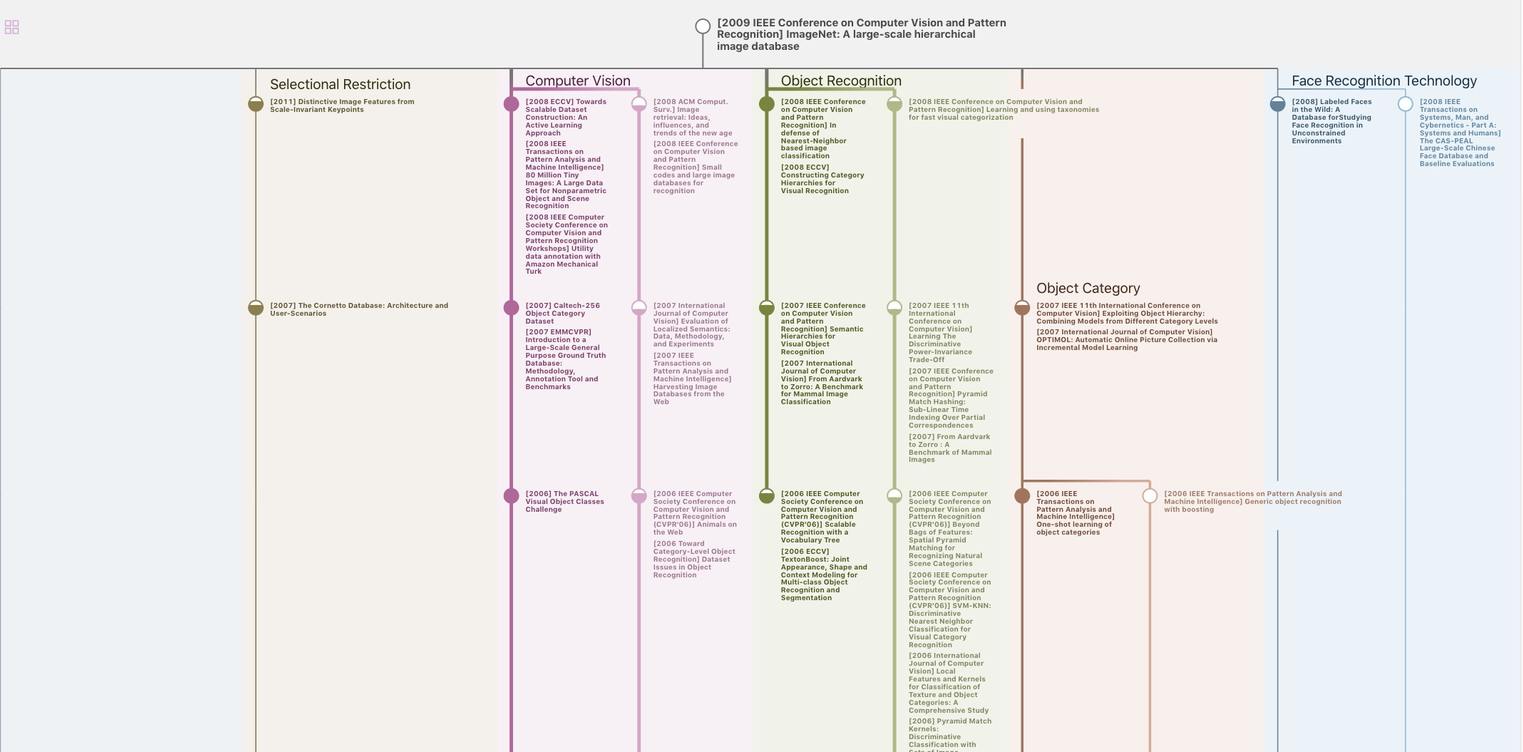
生成溯源树,研究论文发展脉络
Chat Paper
正在生成论文摘要