Deep learning for classification of late gadolinium enhancement lesions based on the 16-segment left ventricular model
PHYSICA MEDICA-EUROPEAN JOURNAL OF MEDICAL PHYSICS(2024)
摘要
Purpose: This study aimed to develop and validate a deep learning-based method that allows for segmental analysis of myocardial late gadolinium enhancement (LGE) lesions. Methods: Cardiac LGE data from 170 patients with coronary artery disease and non-ischemic heart disease were used for training, validation, and testing. Short-axis images were transformed to polar space after identification of the left ventricular (LV) center point and anterior right ventricular (RV) insertion point. Images were obtained after dividing the polar transformed images into segments based on the 16-segment LV model. Five different deep convolutional neural network (CNN) models were developed and validated using the labeled data, where the image after the division corresponded to a segment, and the lesion labeling was based on the 16-segment LV model. Unseen testing data were used to evaluate the performance of the lesion classification. Results: Without manual lesion segmentation and annotation, the proposed method showed an area under the curve (AUC) of 0.875, and a precision, recall, and F1-score of 0.723, 0.783, and 0.752, respectively for the lesion class when the pretrained ResNet50 model was tested for all slice images. The two pretrained models of ResNet50 and EfficientNet-B0 outperformed the three non-pretrained CNN models in terms of AUCs (0.873-0.875 vs. 0.834-0.841). Conclusion: The proposed method is based on learning a deep CNN model from polar transformed images to predict LGE lesions with good accuracy and does not require time-consuming annotation procedures such as lesion segmentation.
更多查看译文
关键词
Magnetic resonance imaging,Late gadolinium enhancement,Cardiac imaging,Medical image analysis,Deep learning
AI 理解论文
溯源树
样例
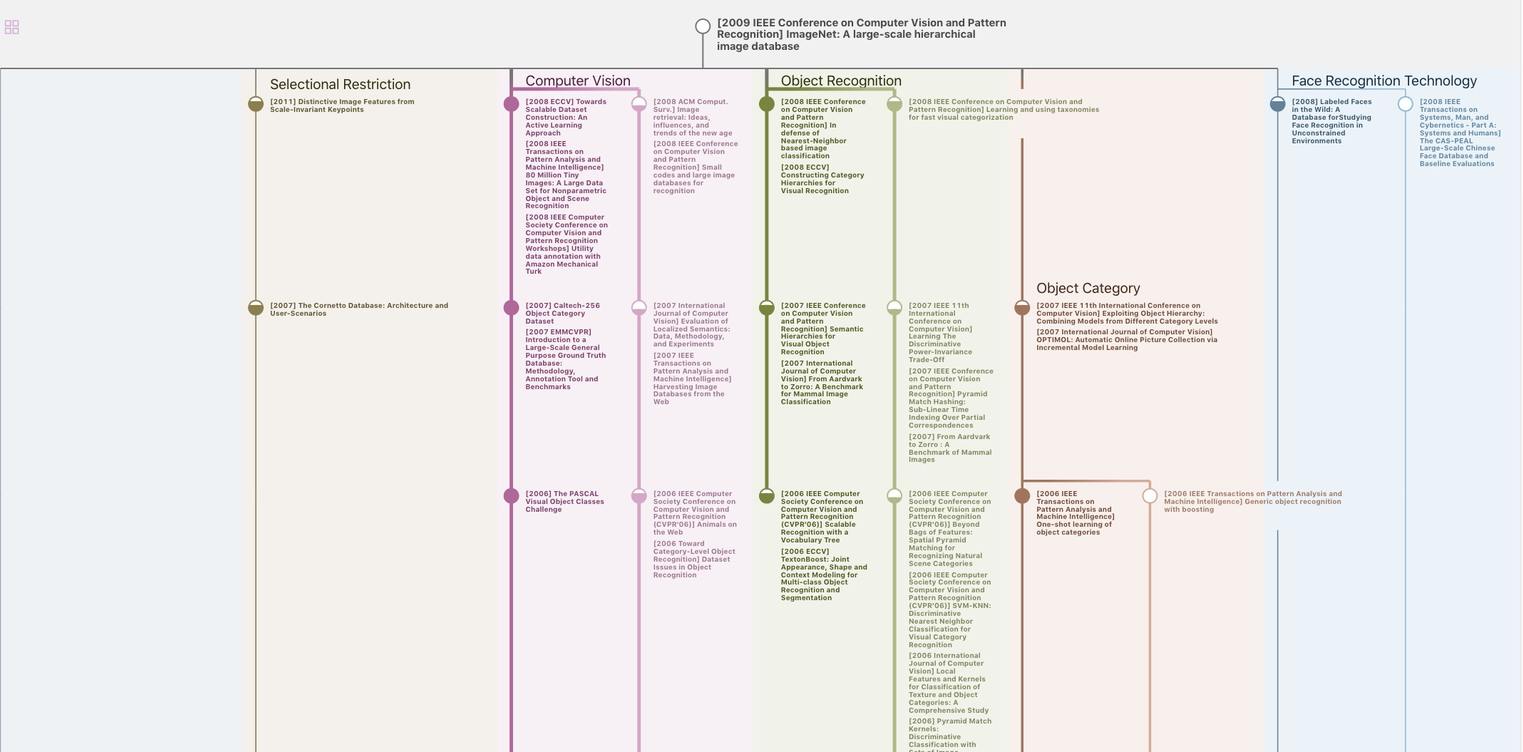
生成溯源树,研究论文发展脉络
Chat Paper
正在生成论文摘要