Universal Object-Level Adversarial Attack in Hyperspectral Image Classification
IEEE TRANSACTIONS ON GEOSCIENCE AND REMOTE SENSING(2023)
摘要
The vulnerability of deep neural networks (DNNs) has garnered significant attention. Various advanced adversarial attack methods have been proposed. However, these methods exhibit higher attack performance on three-band natural images while struggling to handle high-dimensional attacks in terms of attack transferability and robustness. Hyperspectral images (HSIs), unlike natural images, possess high-dimensional and redundant spectral information. On the one hand, different classification models focus on distinct discriminative spectral bands, leading to poor transferability. On the other hand, most existing attack methods are implemented at the pixel level, making them less resilient to image-processing-based defenses. In this article, we address the improvement of transferability and robustness in high-dimensional attacks and introduce a universal object-level adversarial attack method in HSI classification. We found that perturbations with higher similarity in a local region can decrease the sensitivity of adversarial attacks to various discriminative spectral patterns and enhance resistance to image-processing-based defenses. Consequently, we construct spatial and spectral oversegmented templates by utilizing the local smooth properties of HSIs, aiming to promote similarity among perturbations within a local region. Extensive experiments conducted on two real HSI datasets validate that our method enhances the attack transferability and robustness of several existing attack methods. By incorporating the object-level adversarial attack with the baseline fast gradient sign method (FGSM), momentum iterative FGSM (MI-FGSM), and variance tuning MI-FGSM (VMI-FGSM), the average transferability success rate of the proposed method has increased by 7.38% on the PaviaU dataset and 9.30% on the HoustonU 2018 dataset than the baselines. Meanwhile, the proposed method outperforms the baselines by an average of 6.19% on the PaviaU dataset and 10.05% on the HoustonU 2018 dataset in attacking image-processing-based defense models. The code is available at https://github.com/ AAAA-CS/SS_FGSM_HyperspectralAdversarialAttack.
更多查看译文
关键词
Perturbation methods,Robustness,Hyperspectral imaging,Iterative methods,Training,Sensitivity,Closed box,Adversarial attack,adversarial defense,hyperspectral image (HSI) classification
AI 理解论文
溯源树
样例
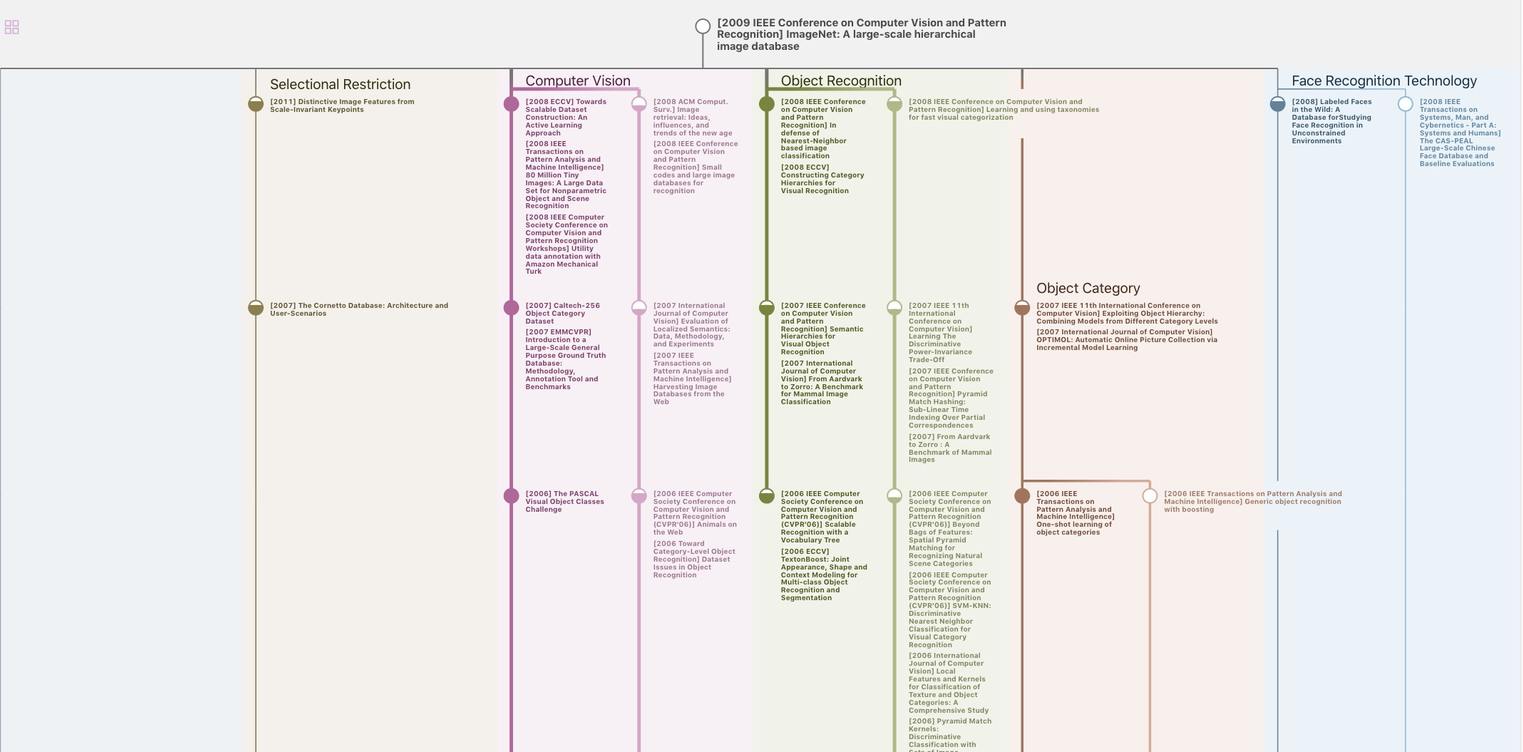
生成溯源树,研究论文发展脉络
Chat Paper
正在生成论文摘要