Exploring Continual Learning and Self-learning for Historical Digit Recognition
2023 International Conference on Cyberworlds (CW)(2023)
摘要
Self-learning has demonstrated remarkable performance in computer vision tasks. However, dealing with unlabeled data that needs to be recognized based on previously acquired knowledge is crucial. Specifically, in Continual Learning where new data is incrementally learned, without the need for training from scratch, models often suffer from Catastrophic forgetting. In this study, we investigate the potential of continual self-learning, using LeNet architecture, to address the issue of learning and recognizing unlabeled historical digits by transferring the knowledge gained from the Split-MNIST dataset. The application of Continual self-learning to unlabeled historical digits unlocks the potential for machines to gain a deeper understanding of our digit-based history and provide increasingly insightful interpretations. To the best of our knowledge, this is the first work that investigates Continual Learning in the context of historical handwritten text recognition.
更多查看译文
关键词
Historical digit recognition,Continual learning,Self-learning,Catastrophic forgetting
AI 理解论文
溯源树
样例
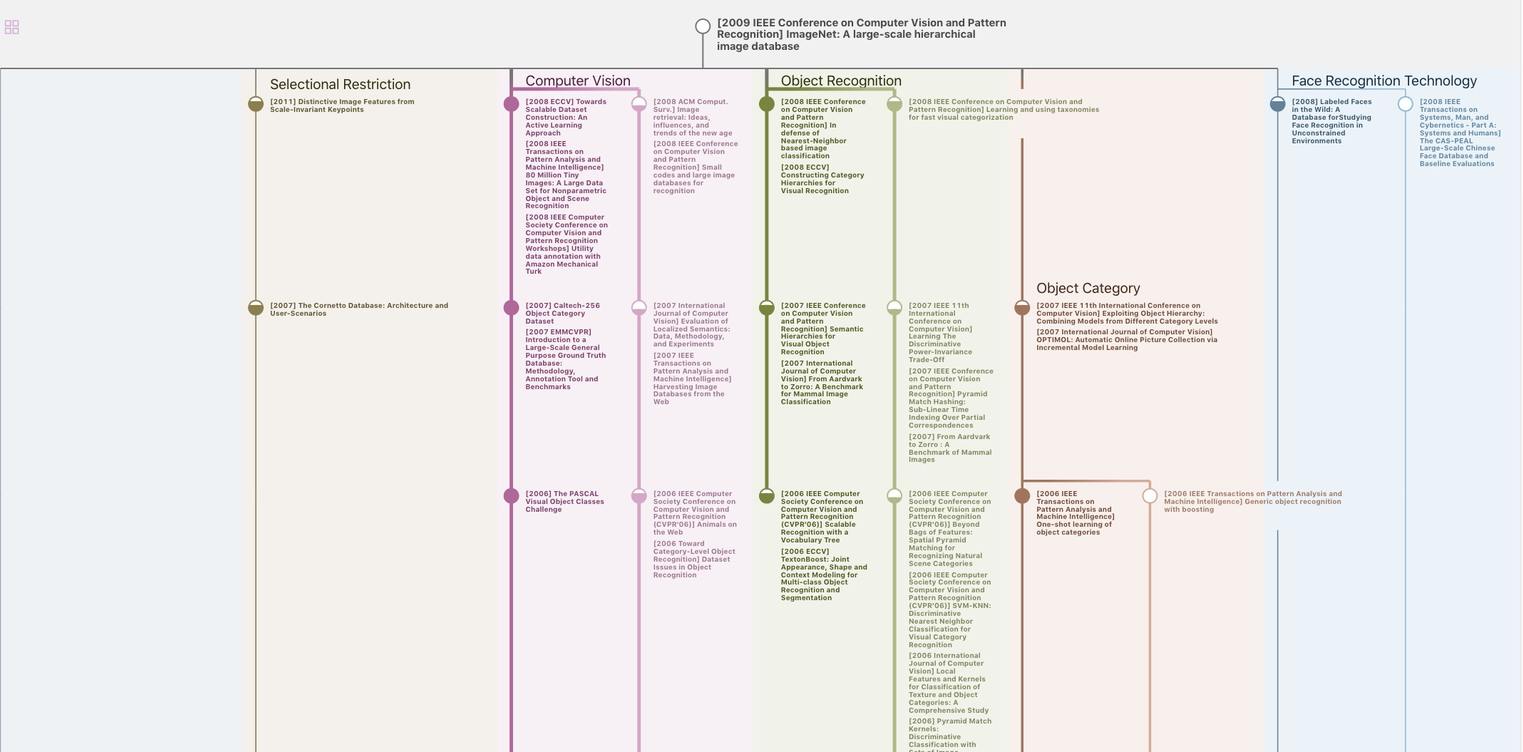
生成溯源树,研究论文发展脉络
Chat Paper
正在生成论文摘要