Discriminative fusion of moments-aligned latent representation of multimodality medical data
PHYSICS IN MEDICINE AND BIOLOGY(2024)
摘要
Fusion of multimodal medical data provides multifaceted, disease-relevant information for diagnosis or prognosis prediction modeling. Traditional fusion strategies such as feature concatenation often fail to learn hidden complementary and discriminative manifestations from high-dimensional multimodal data. To this end, we proposed a methodology for the integration of multimodality medical data by matching their moments in a latent space, where the hidden, shared information of multimodal data is gradually learned by optimization with multiple feature collinearity and correlation constrains. We first obtained the multimodal hidden representations by learning mappings between the original domain and shared latent space. Within this shared space, we utilized several relational regularizations, including data attribute preservation, feature collinearity and feature-task correlation, to encourage learning of the underlying associations inherent in multimodal data. The fused multimodal latent features were finally fed to a logistic regression classifier for diagnostic prediction. Extensive evaluations on three independent clinical datasets have demonstrated the effectiveness of the proposed method in fusing multimodal data for medical prediction modeling.
更多查看译文
关键词
multimodal medical data fusion,latent representation learning,moment matching,feature collinearity,diagnosis and prognosis modeling
AI 理解论文
溯源树
样例
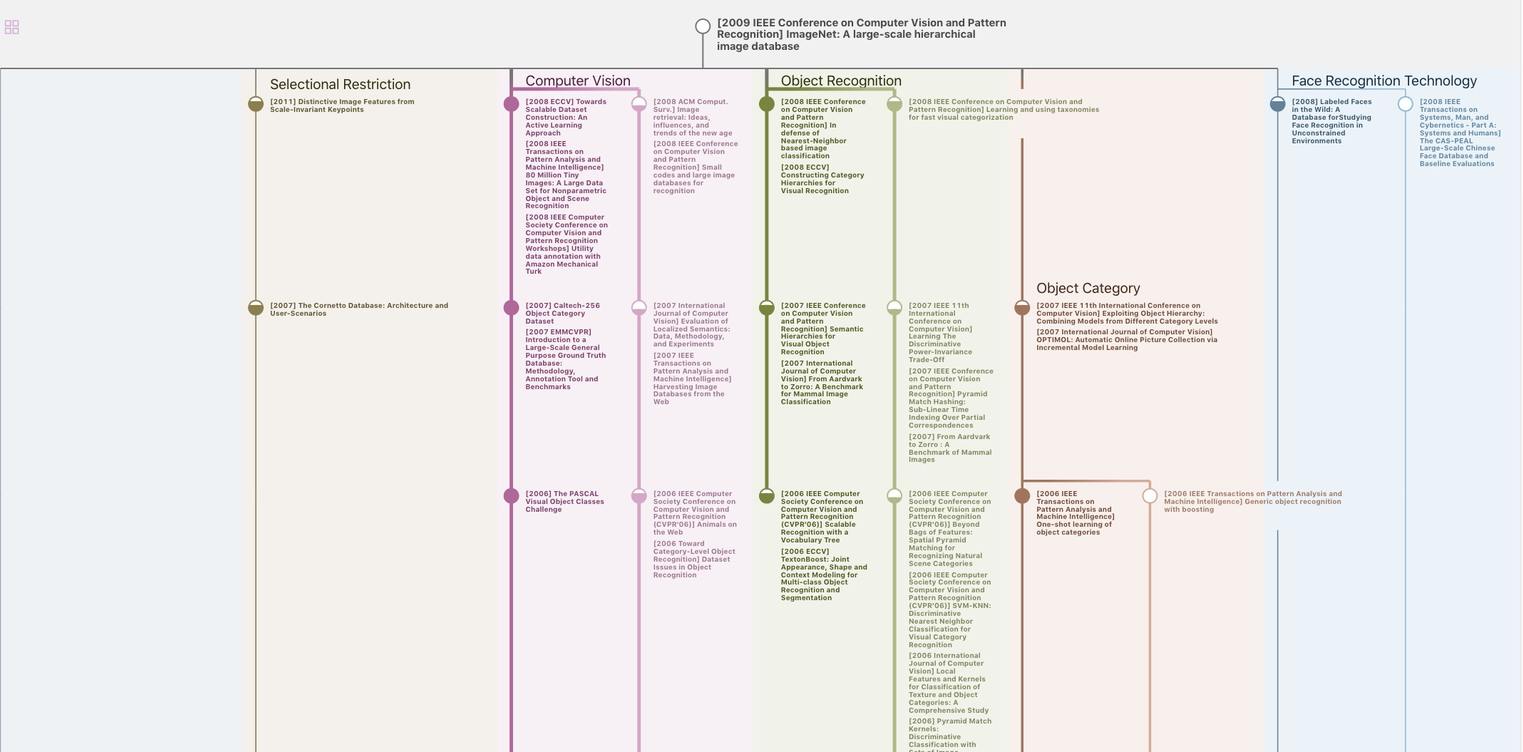
生成溯源树,研究论文发展脉络
Chat Paper
正在生成论文摘要