Propagating moments in probabilistic graphical models for decision support systems
arxiv(2023)
摘要
Probabilistic graphical models are widely used to model complex systems with
uncertainty. Traditionally, Gaussian directed graphical models are applied for
analysis of large networks with continuous variables since they can provide
conditional and marginal distributions in closed form simplifying the
inferential task. The Gaussianity and linearity assumptions are often adequate,
yet can lead to poor performance when dealing with some practical applications.
In this paper, we model each variable in graph G as a polynomial regression of
its parents to capture complex relationships between individual variables and
with utility function of polynomial form. Since the marginal posterior
distributions of individual variables can become analytically intractable, we
develop a message-passing algorithm to propagate information throughout the
network solely using moments which enables the expected utility scores to be
calculated exactly. We illustrate how the proposed methodology works in a
decision problem in energy systems planning.
更多查看译文
AI 理解论文
溯源树
样例
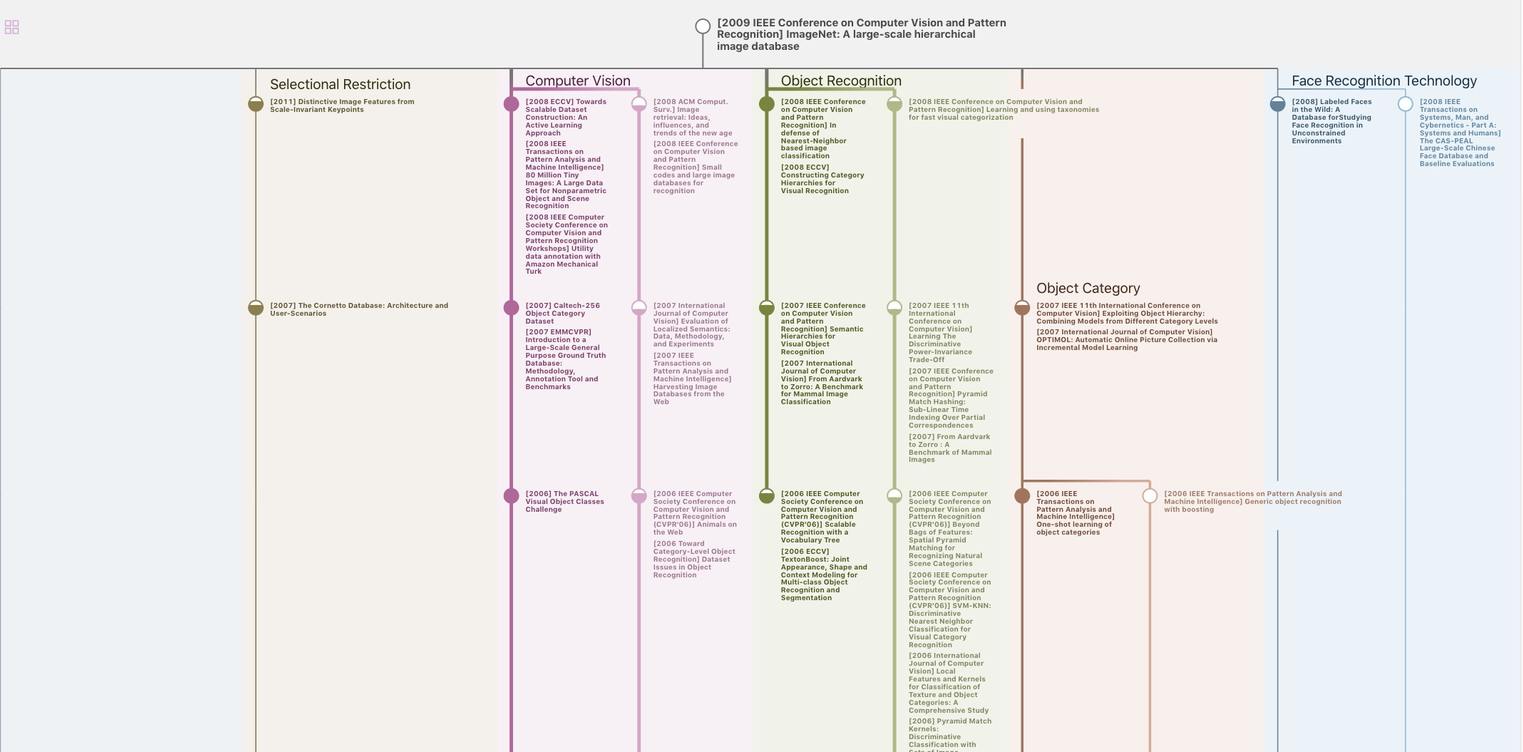
生成溯源树,研究论文发展脉络
Chat Paper
正在生成论文摘要