Constrained Bayesian Optimization under Partial Observations: Balanced Improvements and Provable Convergence
AAAI 2024(2024)
摘要
The partially observable constrained optimization problems (POCOPs) impede data-driven optimization techniques since an infeasible solution of POCOPs can provide little information about the objective as well as the constraints. We endeavor to design an efficient and provable method for expensive POCOPs under the framework of constrained Bayesian optimization. Our method consists of two key components. Firstly, we present an improved design of the acquisition functions that introduce balanced exploration during optimization. We rigorously study the convergence properties of this design to demonstrate its effectiveness. Secondly, we propose Gaussian processes embedding different likelihoods as the surrogate model for partially observable constraints. This model leads to a more accurate representation of the feasible regions compared to traditional classification-based models. Our proposed method is empirically studied on both synthetic and real-world problems. The results demonstrate the competitiveness of our method for solving POCOPs.
更多查看译文
关键词
ML: Optimization,CSO: Constraint Optimization,CSO: Constraint Learning and Acquisition
AI 理解论文
溯源树
样例
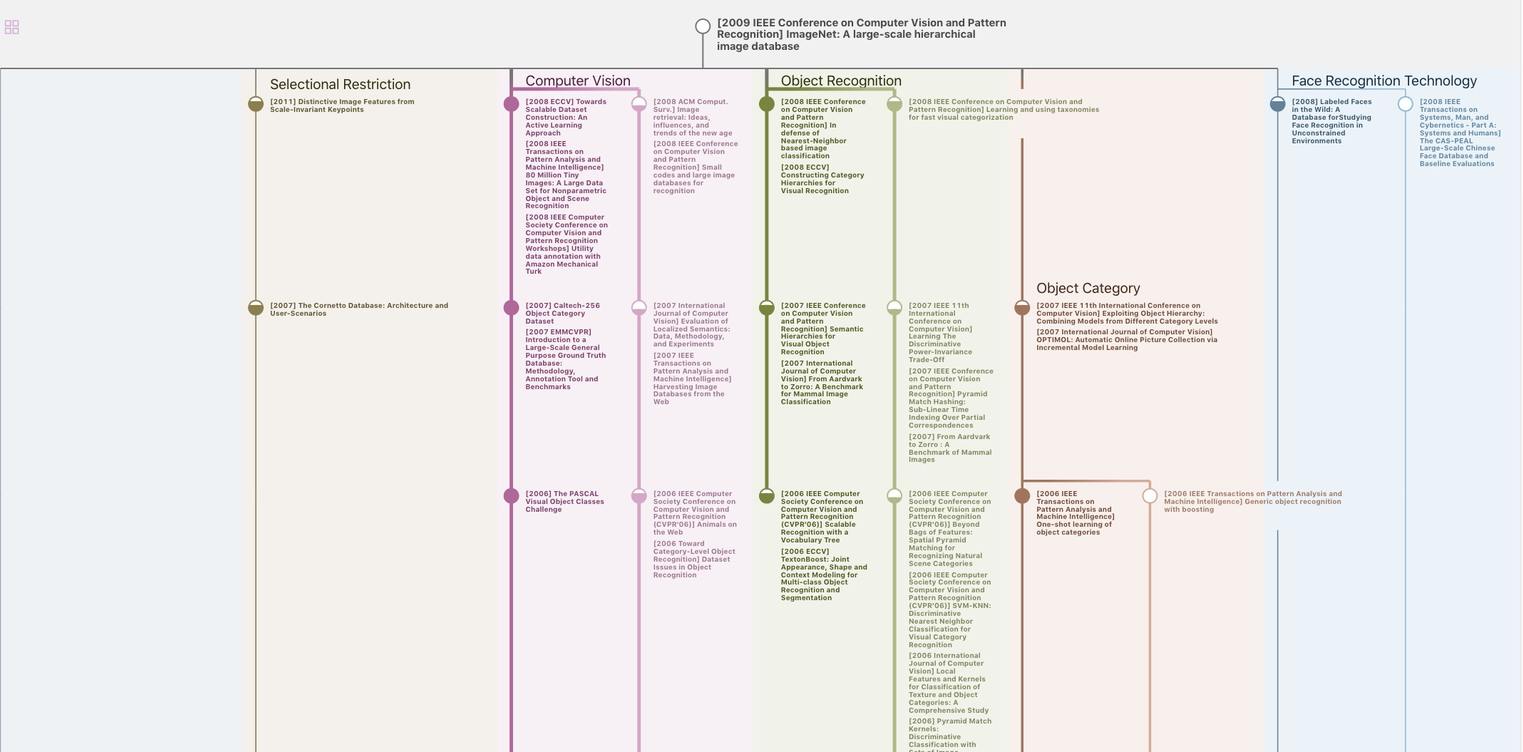
生成溯源树,研究论文发展脉络
Chat Paper
正在生成论文摘要