Detecting anomalous electricity consumption with transformer and synthesized anomalies
PEERJ COMPUTER SCIENCE(2023)
摘要
Non-technical losses are consistently a troubling issue for power suppliers. With the application and popularization of smart grid and advanced measurement systems, it has become possible to use data-driven methods to detect anomalous electricity consumption to reduce non-technical losses. A range of machine learning models have been utilized for detecting anomalous electricity consumption and have achieved promising results. However, with the evolution of techniques like electricity theft, coupled with the exponential increase in electricity consumption data, new challenges are constantly being posed for anomalous electricity consumption detection. We propose a Transformer-based method for detecting anomalous electricity consumption. The Transformer is composed of multi-head attention, layer normalization, point-wise feed-forward network, etc., which can effectively handle electricity consumption timeseries data. Meanwhile, to alleviate the problem of imbalanced training data between anomalous and normal electricity consumption, we propose a method for synthesizing anomalies. The experimental results demonstrate that our proposed Transformer-based method outperforms the state-of-the-art methods in detecting anomalous electricity consumption, achieving a precision of 93.9%, a recall of 96.3%, an F1-score of 0.951, and an accuracy of 95.6% on a dataset released by the State Grid Corporation of China.
更多查看译文
关键词
Electricity consumption,Transformer,Detection,Synthesized anomalies
AI 理解论文
溯源树
样例
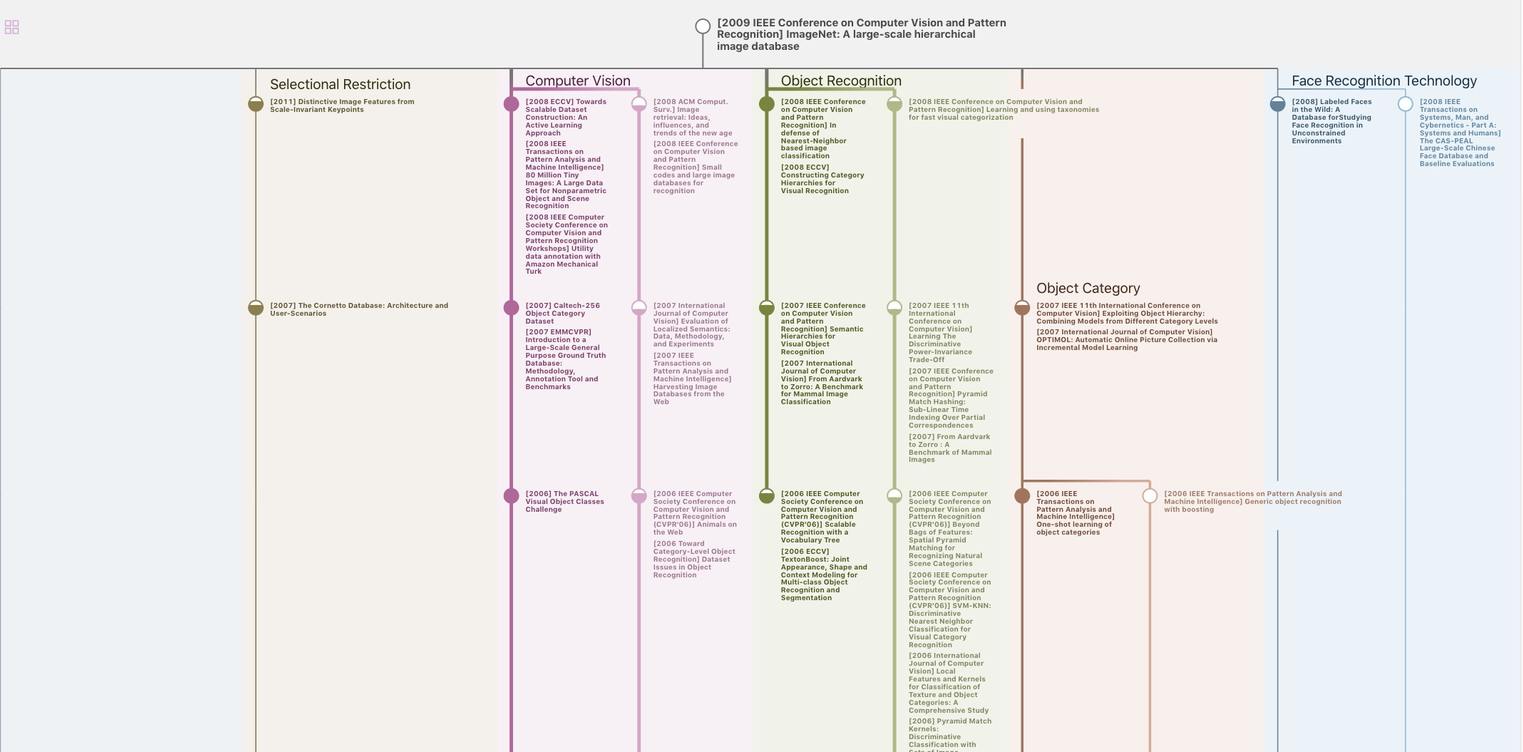
生成溯源树,研究论文发展脉络
Chat Paper
正在生成论文摘要