GAN Memory with No Forgetting
arXiv (Cornell University)(2020)
摘要
As a fundamental issue in lifelong learning, catastrophic forgetting is directly caused by inaccessible historical data; accordingly, if the data (information) were memorized perfectly, no forgetting should be expected. Motivated by that, we propose a GAN memory for lifelong learning, which is capable of remembering a stream of datasets via generative processes, with \emph{no} forgetting. Our GAN memory is based on recognizing that one can modulate the "style" of a GAN model to form perceptually-distant targeted generation. Accordingly, we propose to do sequential style modulations atop a well-behaved base GAN model, to form sequential targeted generative models, while simultaneously benefiting from the transferred base knowledge. The GAN memory -- that is motivated by lifelong learning -- is therefore itself manifested by a form of lifelong learning, via forward transfer and modulation of information from prior tasks. Experiments demonstrate the superiority of our method over existing approaches and its effectiveness in alleviating catastrophic forgetting for lifelong classification problems. Code is available at https://github.com/MiaoyunZhao/GANmemory_LifelongLearning.
更多查看译文
关键词
forgetting,memory
AI 理解论文
溯源树
样例
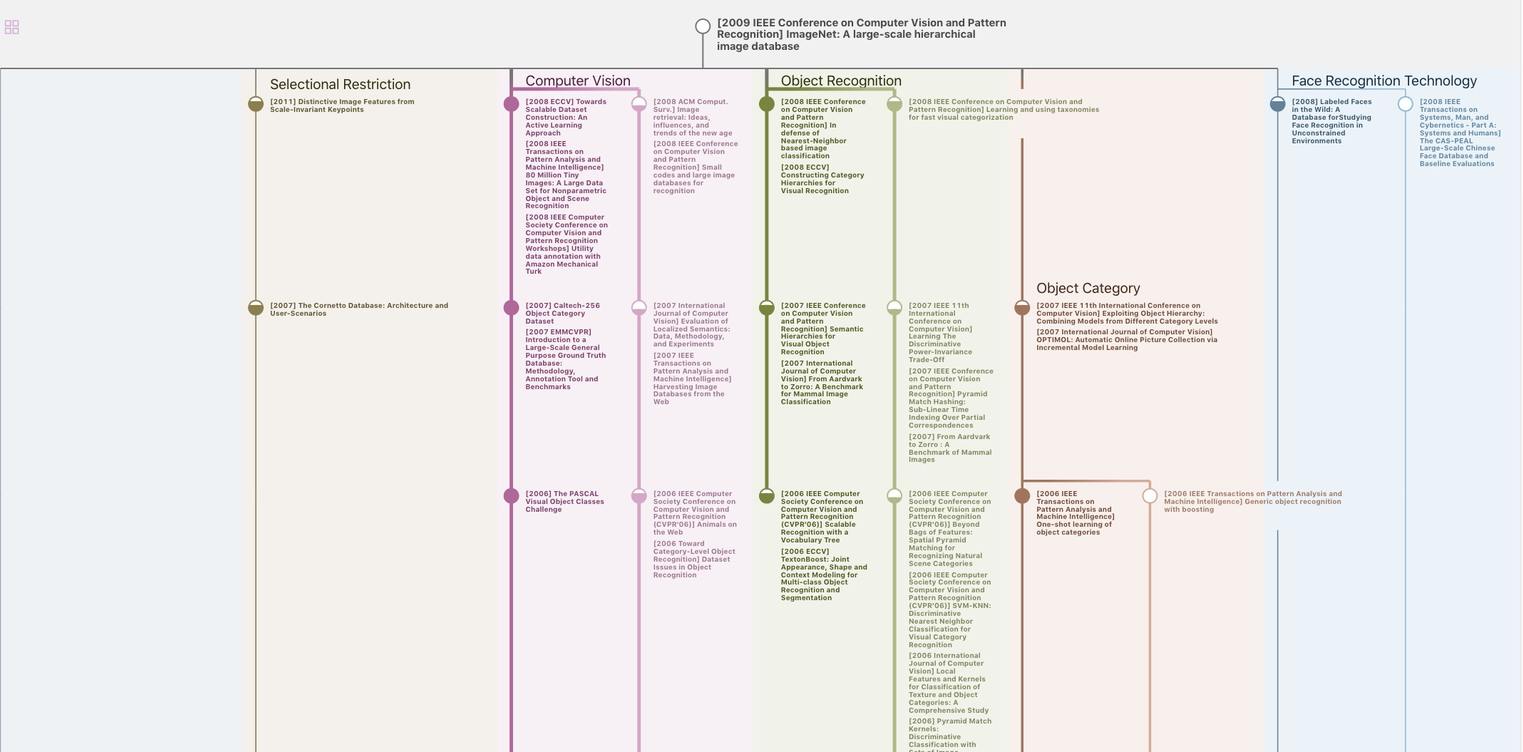
生成溯源树,研究论文发展脉络
Chat Paper
正在生成论文摘要