A multiparametric MRI-based machine learning to distinguish between uterine sarcoma and benign leiomyoma: comparison with 18F-FDG PET/CT
Clinical Radiology(2019)
摘要
•The AUCs of univariate models using MRI parameters were not good. •The AUC of multivariate LR model was superior to that of SUVmax. •And it was comparable to the AUC of the two radiologists. AIM To compare the performance of machine learning using multiparametric magnetic resonance imaging (mp-MRI) and positron-emission tomography (PET) to distinguish between uterine sarcoma and leiomyoma. MATERIALS AND METHODS This retrospective study was approved by the institutional review board and informed consent was waived. Sixty-seven consecutive patients with uterine sarcoma or leiomyoma who underwent pelvic 3 T MRI and PET were included. Of 67 patients, 11 had uterine sarcomas and 56 had leiomyomas. Seven different parameters were measured in the tumours, from T2-weighted, T1-weighted, contrast-enhanced, and diffusion-weighted MRI, and PET. The areas under the receiver operating characteristic curves (AUC) with a leave-one-out cross-validation were used to compare the diagnostic performances of the univariate and multivariate logistic regression (LR) model with those of two board-certified radiologists. RESULTS The AUCs of the univariate models using MRI parameters (0.68–0.8) were inferior to that of the maximum standardised uptake value (SUVmax) of PET (0.85); however, the AUC of the multivariate LR model (0.92) was superior to that of SUVmax, and comparable to that of the board-certified radiologists (0.97 and 0.89). CONCLUSION The diagnostic performance of the machine learning using mp-MRI was superior to PET and comparable to that of experienced radiologists. To compare the performance of machine learning using multiparametric magnetic resonance imaging (mp-MRI) and positron-emission tomography (PET) to distinguish between uterine sarcoma and leiomyoma. This retrospective study was approved by the institutional review board and informed consent was waived. Sixty-seven consecutive patients with uterine sarcoma or leiomyoma who underwent pelvic 3 T MRI and PET were included. Of 67 patients, 11 had uterine sarcomas and 56 had leiomyomas. Seven different parameters were measured in the tumours, from T2-weighted, T1-weighted, contrast-enhanced, and diffusion-weighted MRI, and PET. The areas under the receiver operating characteristic curves (AUC) with a leave-one-out cross-validation were used to compare the diagnostic performances of the univariate and multivariate logistic regression (LR) model with those of two board-certified radiologists. The AUCs of the univariate models using MRI parameters (0.68–0.8) were inferior to that of the maximum standardised uptake value (SUVmax) of PET (0.85); however, the AUC of the multivariate LR model (0.92) was superior to that of SUVmax, and comparable to that of the board-certified radiologists (0.97 and 0.89). The diagnostic performance of the machine learning using mp-MRI was superior to PET and comparable to that of experienced radiologists.
更多查看译文
关键词
uterine sarcoma,benign leiomyoma,machine learning,mri-based,f-fdg
AI 理解论文
溯源树
样例
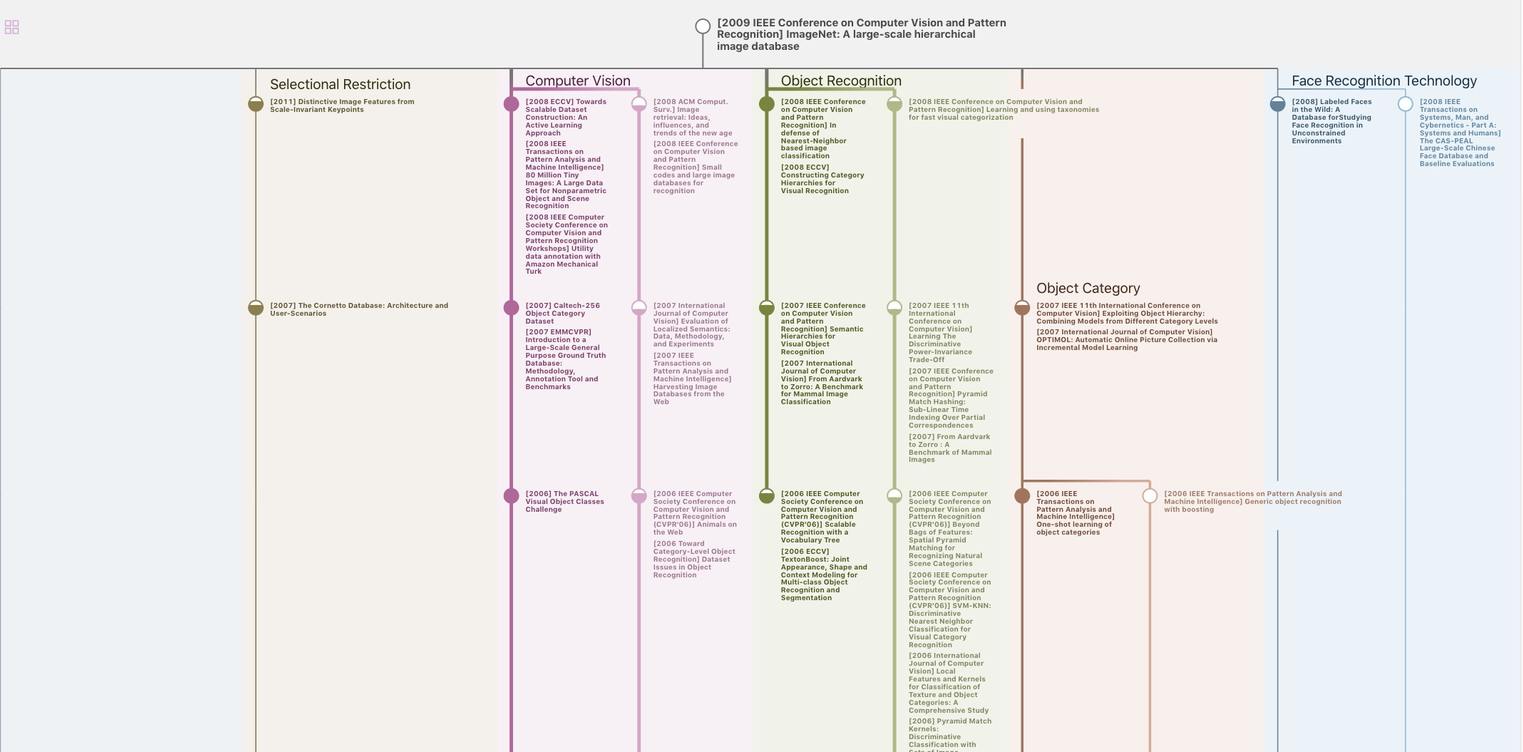
生成溯源树,研究论文发展脉络
Chat Paper
正在生成论文摘要