Discrepancy-based Evolutionary Diversity Optimization
arXiv (Cornell University)(2018)
摘要
Diversity plays a crucial role in evolutionary computation. While diversity has been mainly used to prevent the population of an evolutionary algorithm from premature convergence, the use of evolutionary algorithms to obtain a diverse set of solutions has gained increasing attention in recent years. Diversity optimization in terms of features on the underlying problem allows to obtain a better understanding of possible solutions to the problem at hand and can be used for algorithm selection when dealing with combinatorial optimization problems such as the Traveling Salesperson Problem. We explore the use of the star-discrepancy measure to guide the diversity optimization process of an evolutionary algorithm. In our experimental investigations, we consider our discrepancy-based diversity optimization approaches for evolving diverse sets of images as well as instances of the Traveling Salesperson problem where a local search is not able to find near optimal solutions. Our experimental investigations comparing three diversity optimization approaches show that a discrepancy-based diversity optimization approach using a tie-breaking rule based on weighted differences to surrounding feature points provides the best results in terms of the star discrepancy measure.
更多查看译文
关键词
evolutionary diversity optimization,discrepancy-based
AI 理解论文
溯源树
样例
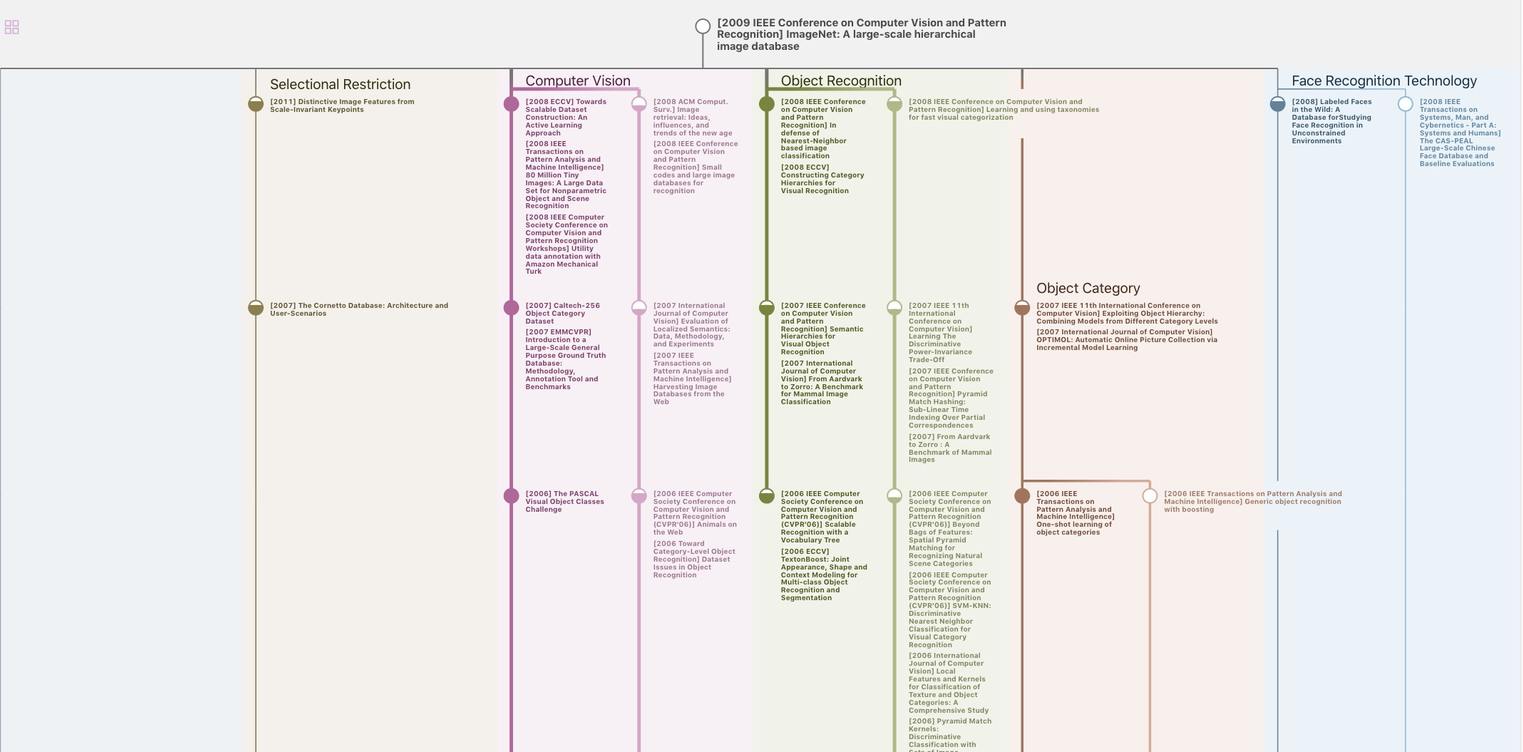
生成溯源树,研究论文发展脉络
Chat Paper
正在生成论文摘要