Online News Emotion Prediction With Bidirectional Lstm
WEB-AGE INFORMATION MANAGEMENT, PT II(2016)
摘要
Recent years have brought a significant growth in the volume of user generated data. Sentiment analysis is a crucial tool in the mining of such data, which is of great value for both improving particular services and assisting organizations' decision making process. Existing research focuses on identifying sentiment polarity on subjective text, such as tweets and product reviews. Sentiment analysis on news still remains a challenge: identifying effective emotion-differentiated features automatically from the more objective content, and modeling the longer document semantically. In this paper, we tackle this problem by improving document representations. From the word level, we implemented skip-gram model to learn the word representations with rich contextual information. Moreover, we propose two document representation approaches based on neural networks. We first introduce bidirectional long short-term memory (BLSTM) neural network to capture the complete contextual information in long news articles. In order to extract more salient information from document, we integrate a convolutional neural network with BLSTM to augment the document representations. Extensive experiments show the proposed model outperforms the other baseline methods.
更多查看译文
关键词
Sentiment analysis, Document representation, Bidirectional LSTM
AI 理解论文
溯源树
样例
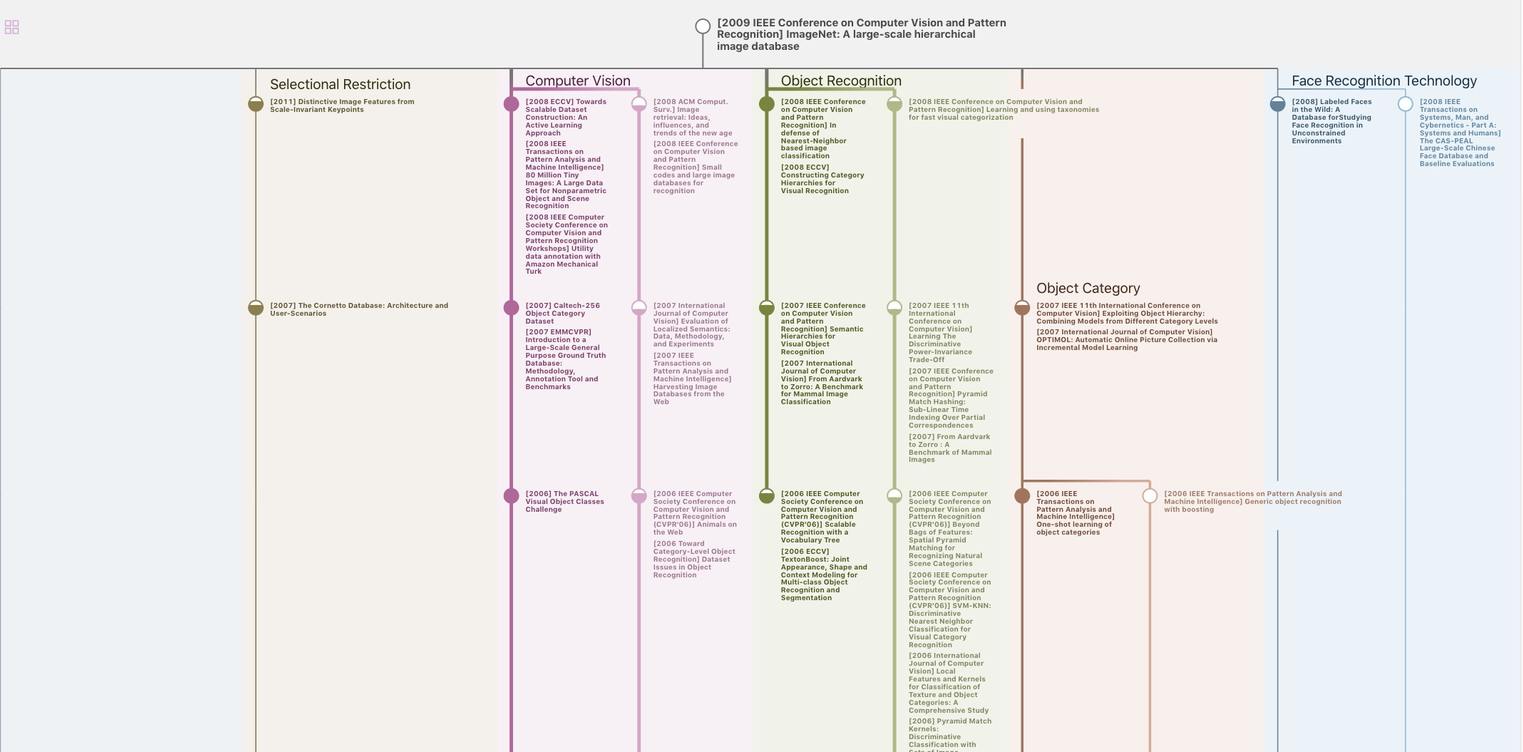
生成溯源树,研究论文发展脉络
Chat Paper
正在生成论文摘要