Semi-parametric inference for large-scale data with temporally dependent noise
ELECTRONIC JOURNAL OF STATISTICS(2023)
摘要
Temporal dependence is frequently encountered in large-scale structured noisy data, arising from scientific studies in neuroscience and meteorology, among others. This challenging characteristic may not align with existing theoretical frameworks or data analysis tools. Motivated by multi-session fMRI time series data, this paper introduces a novel semi parametric inference procedure suitable for a broad class of "non-stationary, non-Gaussian, temporally dependent" noise processes in time-course data. It develops a new test statistic based on a tapering-type estimator of the large-dimensional noise auto-covariance matrix and establishes its asymptotic chi-squared distribution. Our method not only relaxes the consistency requirement for the noise covariance matrix estimator but also avoids direct matrix inversion without sacrificing detection power. It adapts well to both stationary and a wider range of temporal noise processes, making it particularly effective for handling challenging scenarios involving very large scales of data and large dimensions of noise covariance matrices. We demonstrate the efficacy of the proposed procedure through simulation evaluations and real fMRI data analysis.
更多查看译文
关键词
Auto-covariance matrix,false discovery rate,fMRI,neuroimaging,partially-linear model
AI 理解论文
溯源树
样例
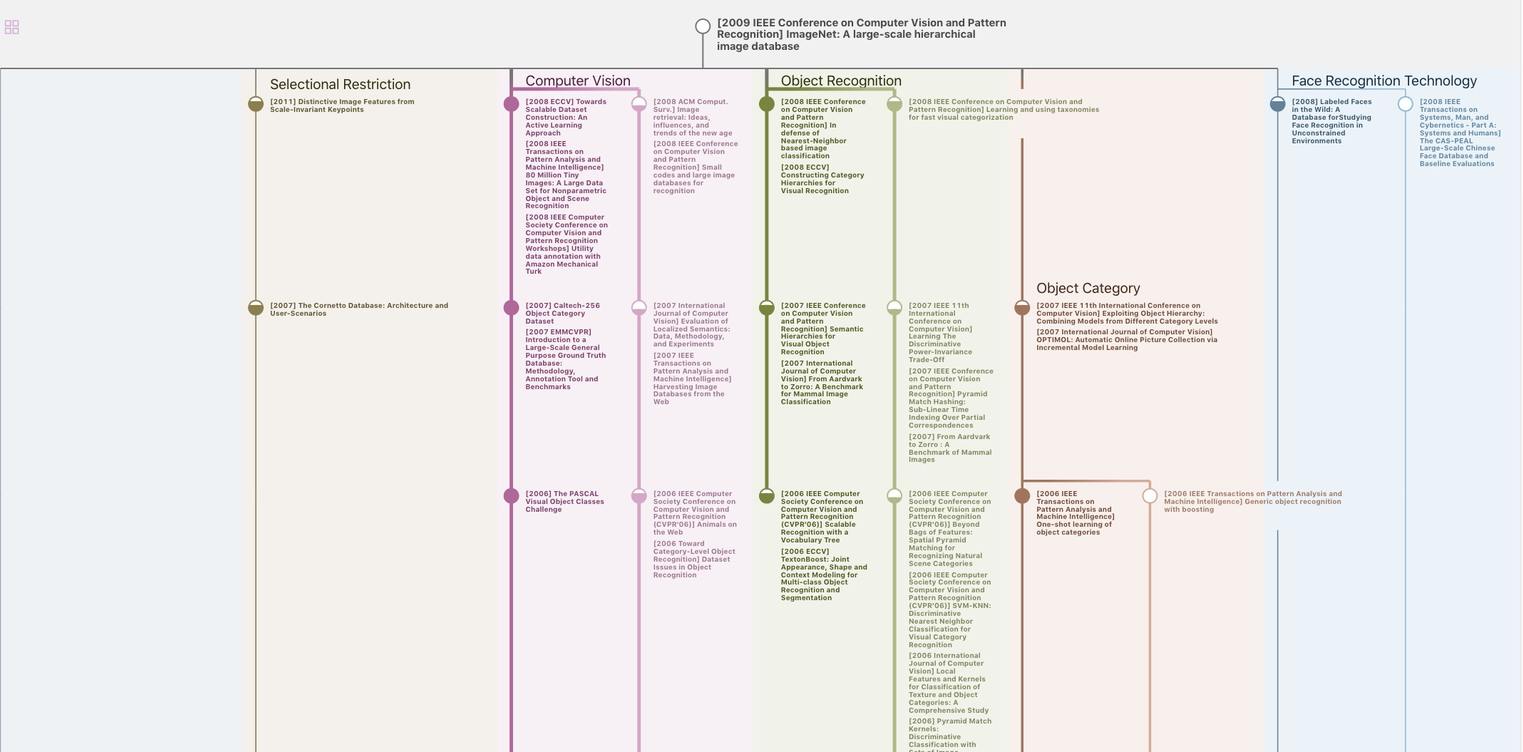
生成溯源树,研究论文发展脉络
Chat Paper
正在生成论文摘要