Collaborative optimisation of lane change decision and trajectory based on double-layer deep reinforcement learning
INTERNATIONAL JOURNAL OF VEHICLE DESIGN(2023)
摘要
In this paper, lane change process of vehicle is divided into two stages: lane change decision and lane change movement, and put forward the double-layer deep reinforcement learning architecture, the use of upper structure deep Q network (DQN) exchange way to control decision, and send the lane changing information to the lower deep deterministic policy gradient (DDPG) of vehicle trajectory control, After the lane change process the collaborative optimisation of DQN was completed through the feedback of vehicle position information before and after lane change., the collaborative optimisation of DQN is completed. The results show that, the proposed two-layer deep reinforcement learning architecture can increase the average velocity of the agent vehicle by 2-5% and reduce the average lateral speed and lateral acceleration by 12.5% and 12.2% respectively in the lane-changing process. Compared with no collaborative optimisation, the optimal lane change timing of the effectively cooptimised two-layer architecture is 34.64%.
更多查看译文
关键词
deep reinforcement learning,lane change decision,lane change action,collaborative optimisation,deep reinforcement learning,lane change decision,lane change action
AI 理解论文
溯源树
样例
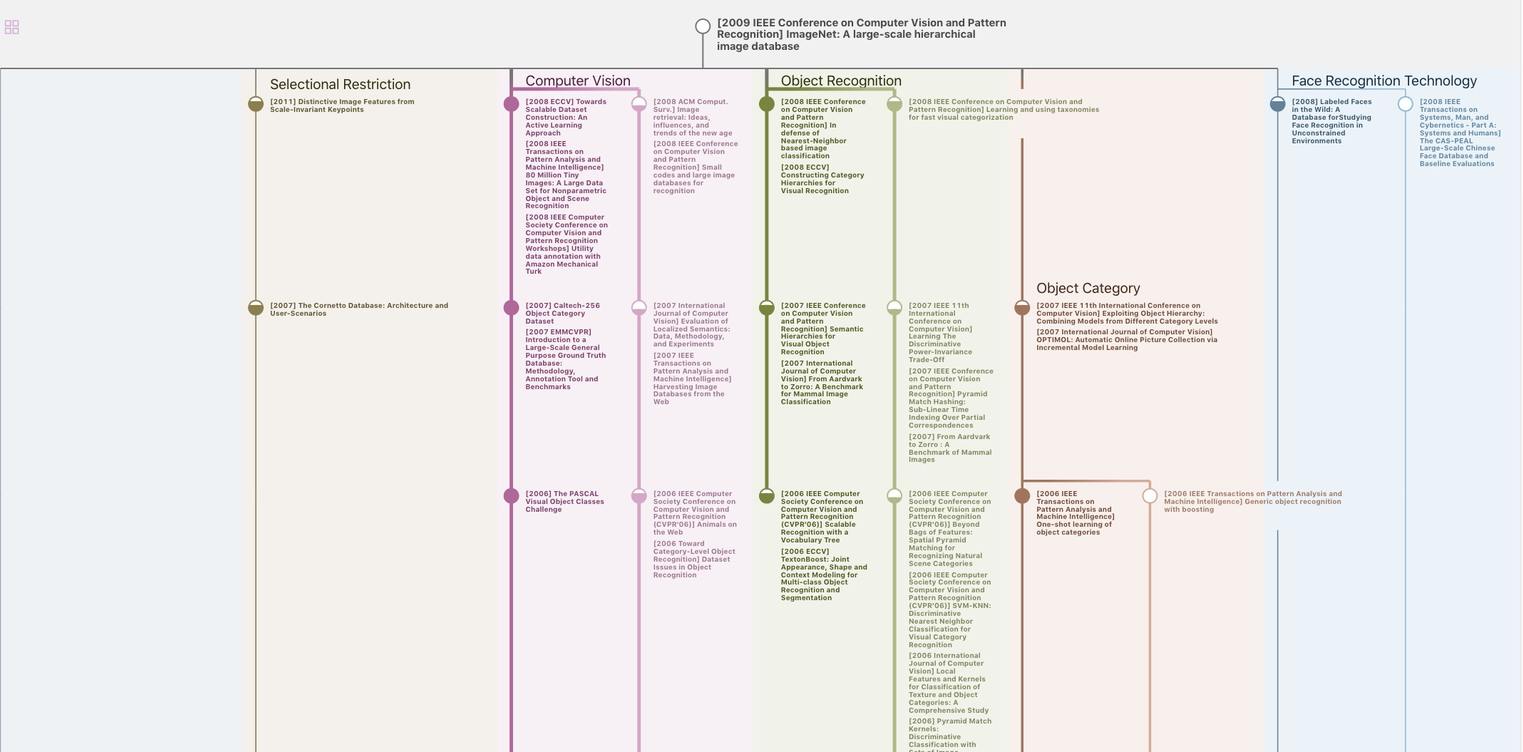
生成溯源树,研究论文发展脉络
Chat Paper
正在生成论文摘要