EDMC: Efficient Multi-View Clustering via Cluster and Instance Space Learning
IEEE TRANSACTIONS ON MULTIMEDIA(2024)
摘要
Multi-view subspace clustering aims to cluster the data lying in a union of subspaces with low dimensions. The commonly used spectral clustering performs the final clustering based on an n x n affinity graph, which suffers from relative high time and space complexity. Some existing works have chosen key anchors with uniform sampling strategy or K-means for dealing with large-scale datasets. However, few of them pay attention to the physical meaning of cluster representation in the column of the dataset for learning informative anchors, which is independent from the instance representation. In this paper, we propose efficient dual multi-view clustering (EDMC) with relative low complexity. To be specific, EDMC makes full use of cluster representation space in the column of the dataset to help produce informative anchors, which has a clear physical meaning and is independent of instance representation in the row. It simultaneously explores the cluster and instance subspace representations to learn anchors for large-scale datasets. We perform anchor learning and efficient multi-view clustering in a unified framework and then adopt an alternative optimization strategy for solving the formulated problem. Extensive experiments performed on different datasets in terms of several metrics validate the superiority of the proposed method.
更多查看译文
关键词
Clustering algorithms,Tensors,Scalability,Representation learning,Optimization,Dimensionality reduction,Complexity theory,Multi-view subspace clustering,cluster representation,instance representation,anchor learning,a unified framework
AI 理解论文
溯源树
样例
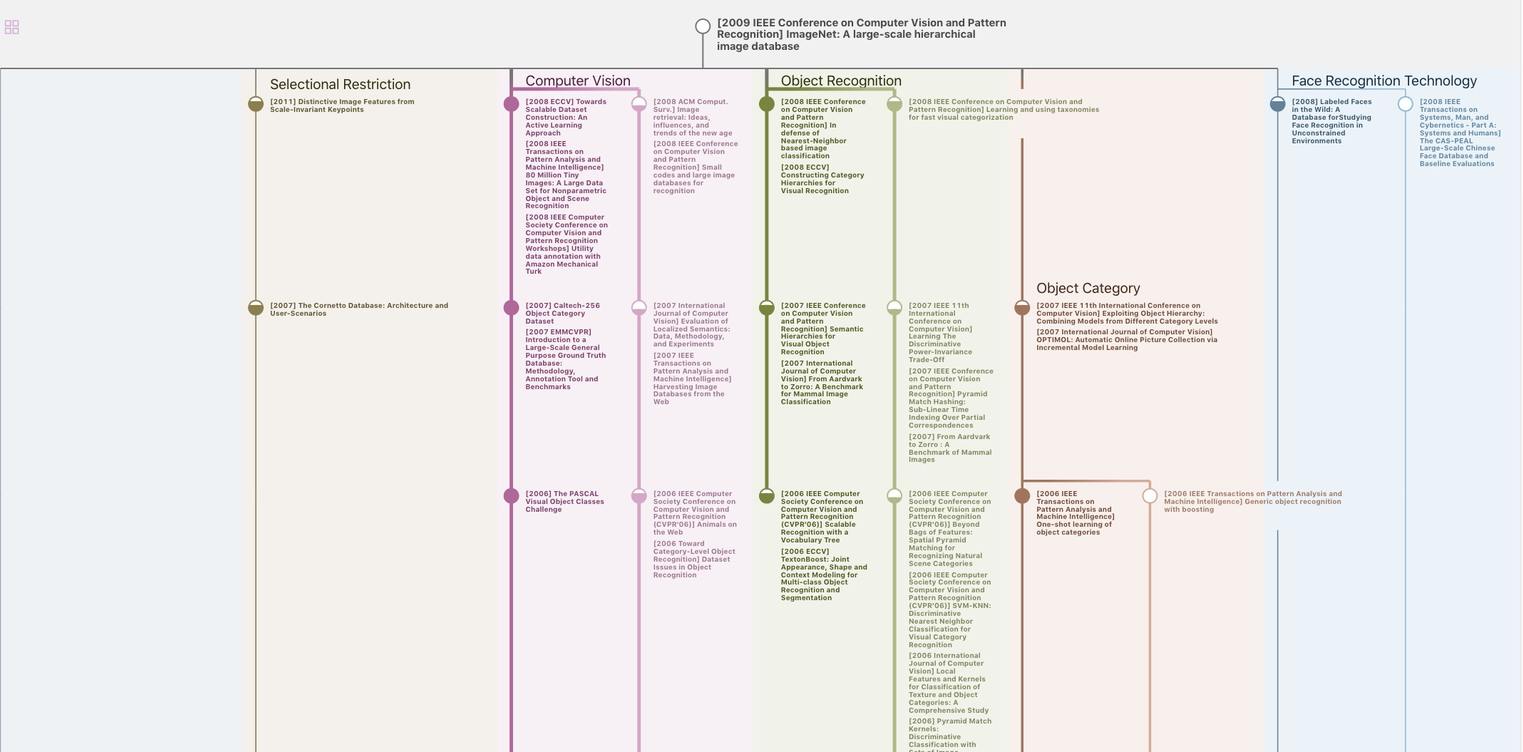
生成溯源树,研究论文发展脉络
Chat Paper
正在生成论文摘要