Asynchronous FDRL-Based Low-Latency Computation Offloading for Integrated Terrestrial and Non-Terrestrial Power IoT
IEEE NETWORK(2023)
摘要
Integrated terrestrial and non-terrestrial power internet of things (IPIoT) has emerged as a paradigm shift to three-dimensional vertical communication networks for power systems in the 6G era. Computation offloading plays key roles in enabling real-time data processing and analysis for electric services. However, computation offloading in IPIoT still faces challenges of coupling between task offloading and computation resource allocation, resource heterogeneity and dynamics, and degraded model training caused by electromagnetic interference (EMI). In this article, we propose an asynchronous federated deep reinforcement learning (AFDRL)-based computation offloading framework for IPIoT, where models are uploaded asynchronously for federated averaging to relieve network congestion and improve global model training. Then, we propose an Asynchronous fedeRated deep reinforcemenT learnIng-baSed low-laTency computation offloading algorithm (ARTIST) to realize low-latency computation offloading through joint optimization of task offloading and computation resource allocation. Particularly, ARTIST adopts EMI-aware federated set determination to remove aberrant local models from federated averaging and improve training accuracy. Next, a case study is developed to validate the excellent performance of ARTIST in reducing task offloading and total queuing delays.
更多查看译文
关键词
Task analysis,Training,Computational modeling,Optimization,Resource management,Real-time systems,Delays,Low latency communication,Power transmission,Internet of Things,6G mobile communication,Power systems,Communication networks,Reinforcement learning,Telecommunication congestion control,Queueing analysis,6G,integrated terrestrial and non-terrestrial power internet of things,computation offloading,asynchronous federated deep reinforcement learning,electromagnetic interference awareness
AI 理解论文
溯源树
样例
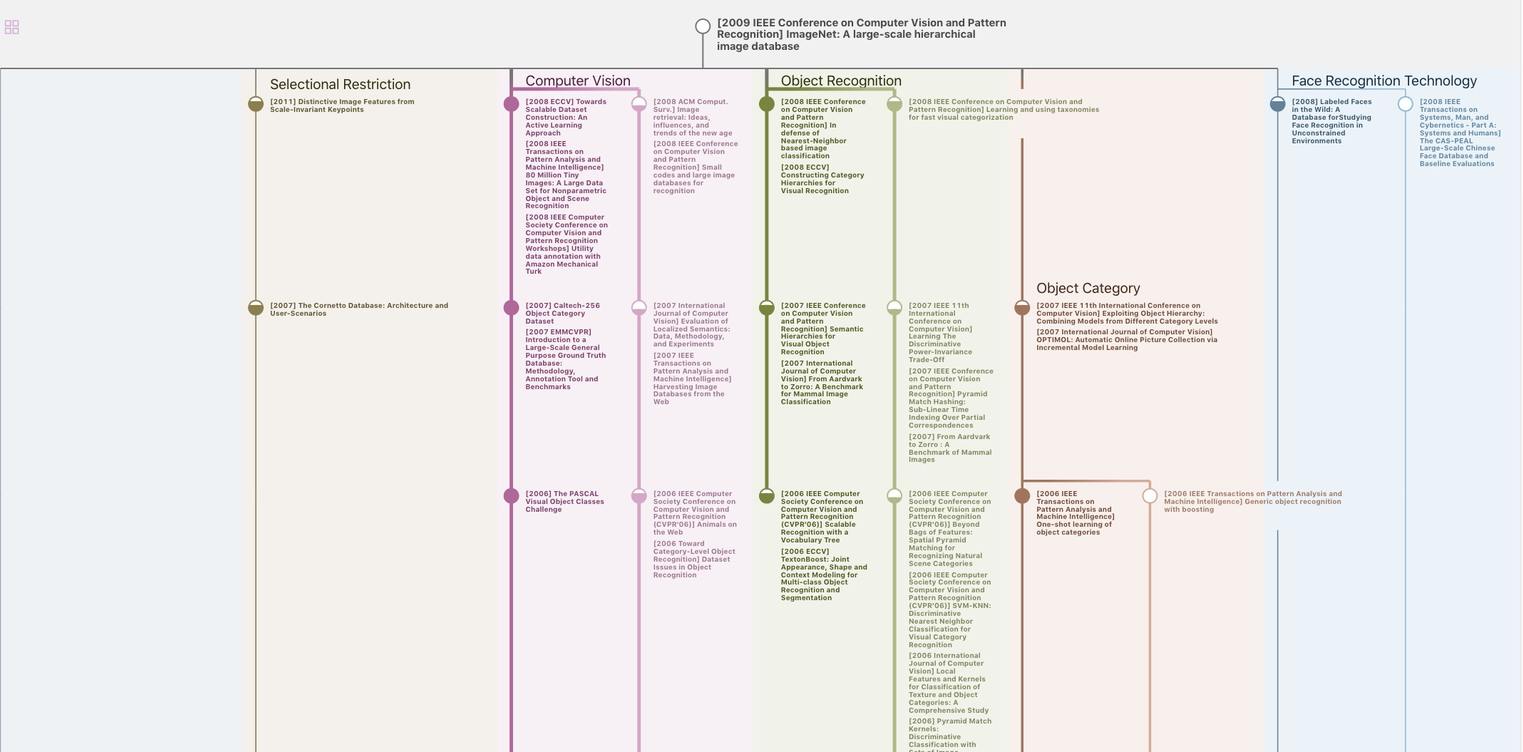
生成溯源树,研究论文发展脉络
Chat Paper
正在生成论文摘要