SpeedAdv: Enabling Green Light Optimized Speed Advisory for Diverse Traffic Lights
IEEE Transactions on Mobile Computing(2023)
摘要
Green Light Optimized Speed Advisory (GLOSA) systems have emerged to allow drivers to pass traffic lights during a green interval. However, various adaptive and intelligent traffic light control approaches have been adopted in many cities, resulting in the development of current GLOSA technologies lagging behind that of traffic light technologies. When taking diverse dynamic traffic lights into account, it is difficult to model the interactions between vehicles and traffic lights, which is further exacerbated by the hybrid control strategies of traffic lights. To this end, we design a new GLOSA system
SpeedAdv
to provide optimal speed advisory for addressing diverse traffic lights. We formulate the problem as a Multi-Agent Markov Decision Process (MAMDP) with an implicit common goal and propose a heterogeneous-agent collaborative framework based on reinforcement learning. Three main modules are used in the system: i) a spatio-temporal relation reasoning module based on the phase-aware attention mechanism pays more attention to the traffic rules and traffic flow diversion of adjacent intersections to predict traffic conditions for a few seconds later; ii) a behavior approximating module based on imitation learning is introduced to approximate the phases of diverse traffic lights; iii) a speed advisory module provides the optimal speed advisory based on policy gradient reinforcement learning relying on the above two modules and other information collected by vehicles. We implement and evaluate
SpeedAdv
with a real-world trajectory dataset, together with a field test based on a prototype system, demonstrating that
SpeedAdv
improves the overall performance by at least
$24.1\%$
in terms of travel time, energy consumption, safety and comfort compared to the state-of-the-art
GreenDrive
method.
更多查看译文
关键词
cooperative vehicle-infrastructure system,GLOSA,deep reinforcement learning,heterogeneous agents
AI 理解论文
溯源树
样例
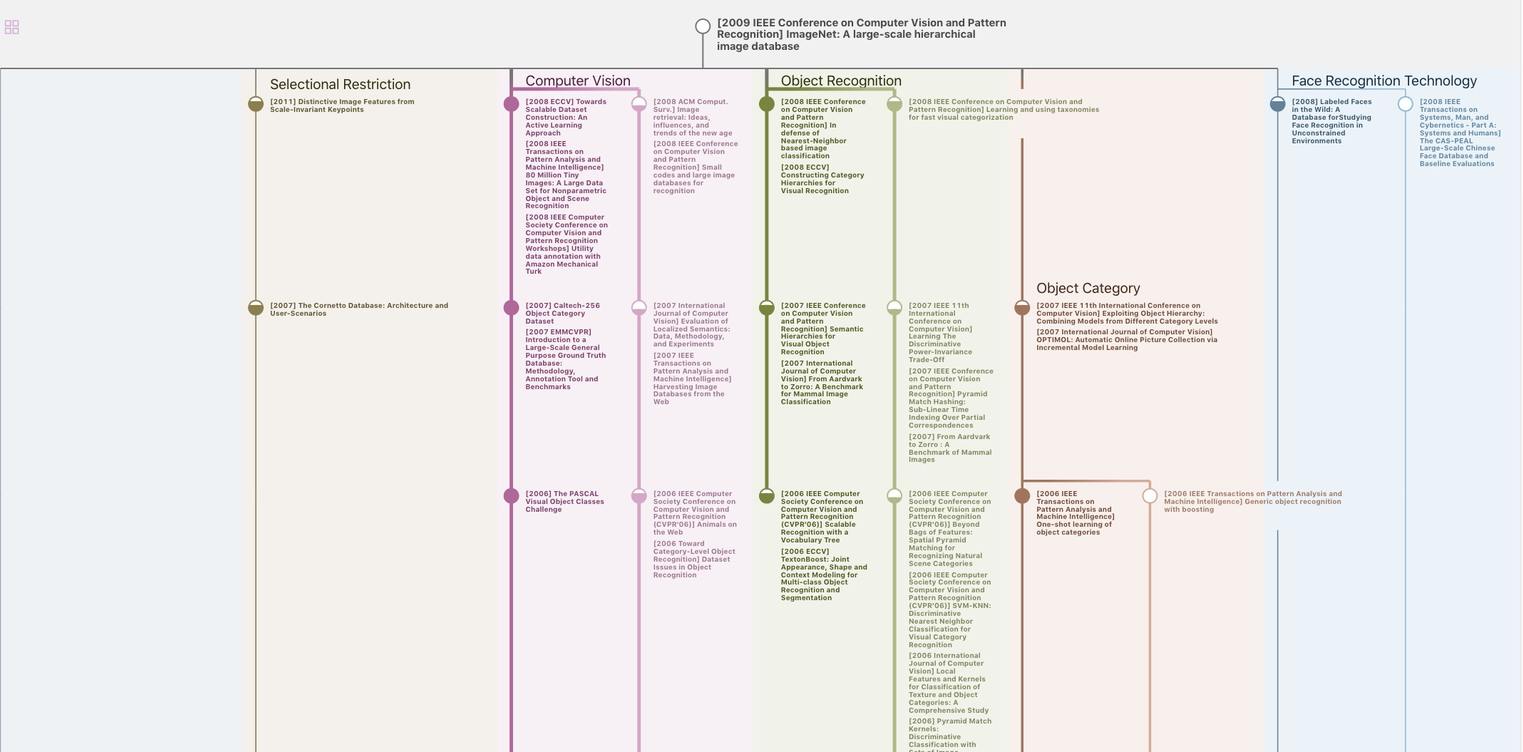
生成溯源树,研究论文发展脉络
Chat Paper
正在生成论文摘要