Individualized Coexpression Network Strategies Employing Transcriptomic Data to Address Challenges in Stratification
Research Square (Research Square)(2023)
摘要
Abstract The traditional network approach in gene expression analysis focuses on identifying relationships among gene expression patterns and associating them with metadata. In contrast, individualized coexpression networks offer a unique perspective by detecting underlying differences in gene expression datasets without known classifications, enabling sample stratification and providing insights into distinct phenotypes. This study aims to identify the strengths and limitations of two main approaches for generating individualized networks to study heterogeneous populations using easily accessible gene expression data. Two distinct families of methods were compared: the first method, based on statistical dependency and commonly used in single-cell genomics, successfully distinguished cell types in various datasets. The second approach, difference of correlation, has been applied to different cancer types and metabolic networks. We used two datasets with known classifications to experiment with scenarios where individualized networks apply. The evaluation involved neuronal cell types and breast cancer datasets. Our results revealed significant differences between the two approaches, suggesting dependencies on sample size, which determine the optimal method for specific applications. Through these evaluations, the dependency method demonstrated superior performance in capturing cell type variations and patient stratification compared to the difference correlation method. Nevertheless, both methods have limitations, including the need for multiple samples to assess correlations and potential challenges in handling heterogeneous sample groups. Individualized coexpression network methods offer valuable insights into biological systems, aiding in cell type classification, disease stratification, and personalized medicine applications. The results indicate their potential for precision medicine; however, researchers should consider their limitations and applicability to specific data types. Addressing sample diversity and size challenges will be pivotal in maximizing the benefits of individualized coexpression networks for future research and clinical applications.
更多查看译文
关键词
stratification
AI 理解论文
溯源树
样例
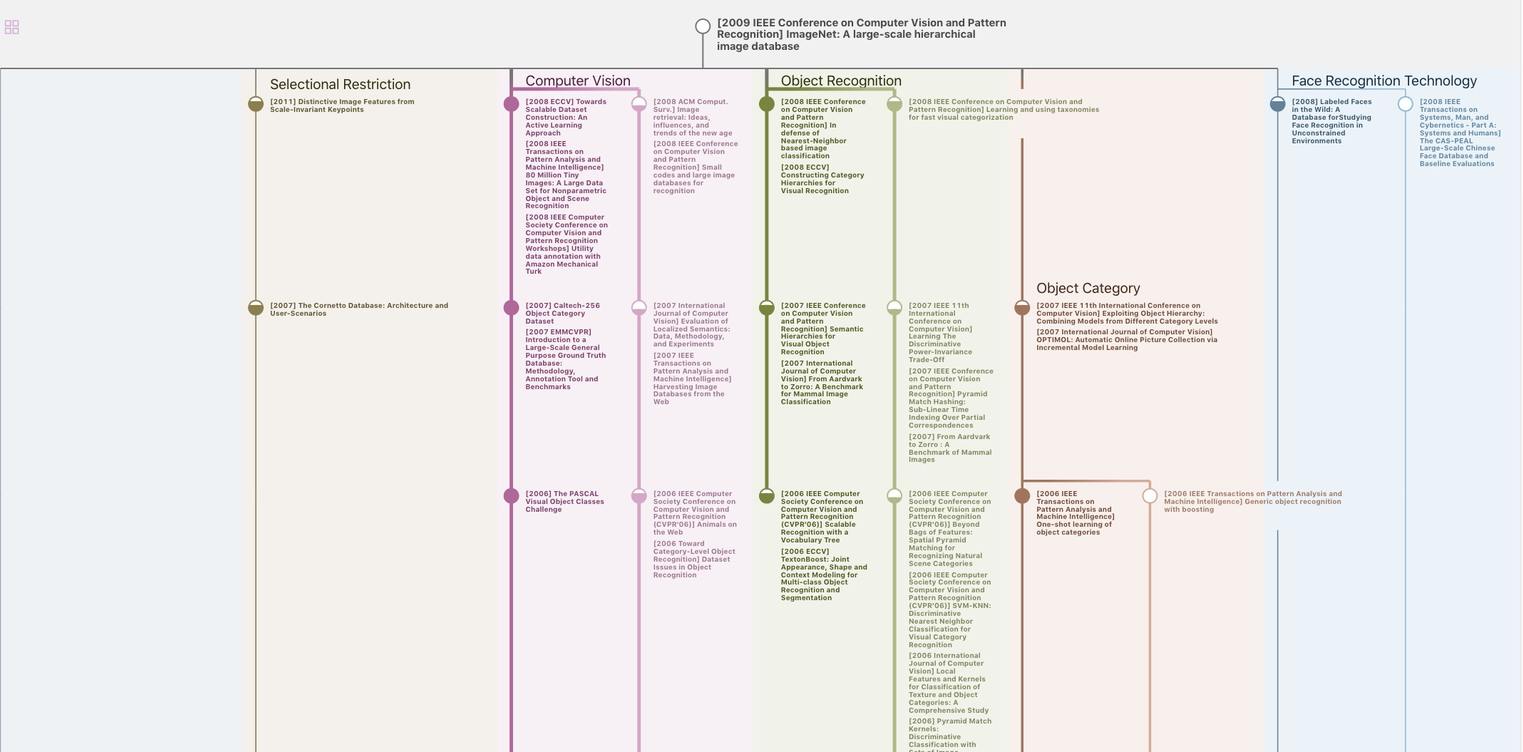
生成溯源树,研究论文发展脉络
Chat Paper
正在生成论文摘要