Human Action Recognition Based on Hierarchical Multi-Scale Adaptive Conv-Long Short-Term Memory Network
APPLIED SCIENCES-BASEL(2023)
摘要
Recently, human action recognition has gained widespread use in fields such as human-robot interaction, healthcare, and sports. With the popularity of wearable devices, we can easily access sensor data of human actions for human action recognition. However, extracting spatio-temporal motion patterns from sensor data and capturing fine-grained action processes remain a challenge. To address this problem, we proposed a novel hierarchical multi-scale adaptive Conv-LSTM network structure called HMA Conv-LSTM. The spatial information of sensor signals is extracted by hierarchical multi-scale convolution with finer-grained features, and the multi-channel features are fused by adaptive channel feature fusion to retain important information and improve the efficiency of the model. The dynamic channel-selection-LSTM based on the attention mechanism captures the temporal context information and long-term dependence of the sensor signals. Experimental results show that the proposed model achieves Macro F1-scores of 0.68, 0.91, 0.53, and 0.96 on four public datasets: Opportunity, PAMAP2, USC-HAD, and Skoda, respectively. Our model demonstrates competitive performance when compared to several state-of-the-art approaches.
更多查看译文
关键词
multi-scale analysis,attention mechanism,feature fusion,human action recognition
AI 理解论文
溯源树
样例
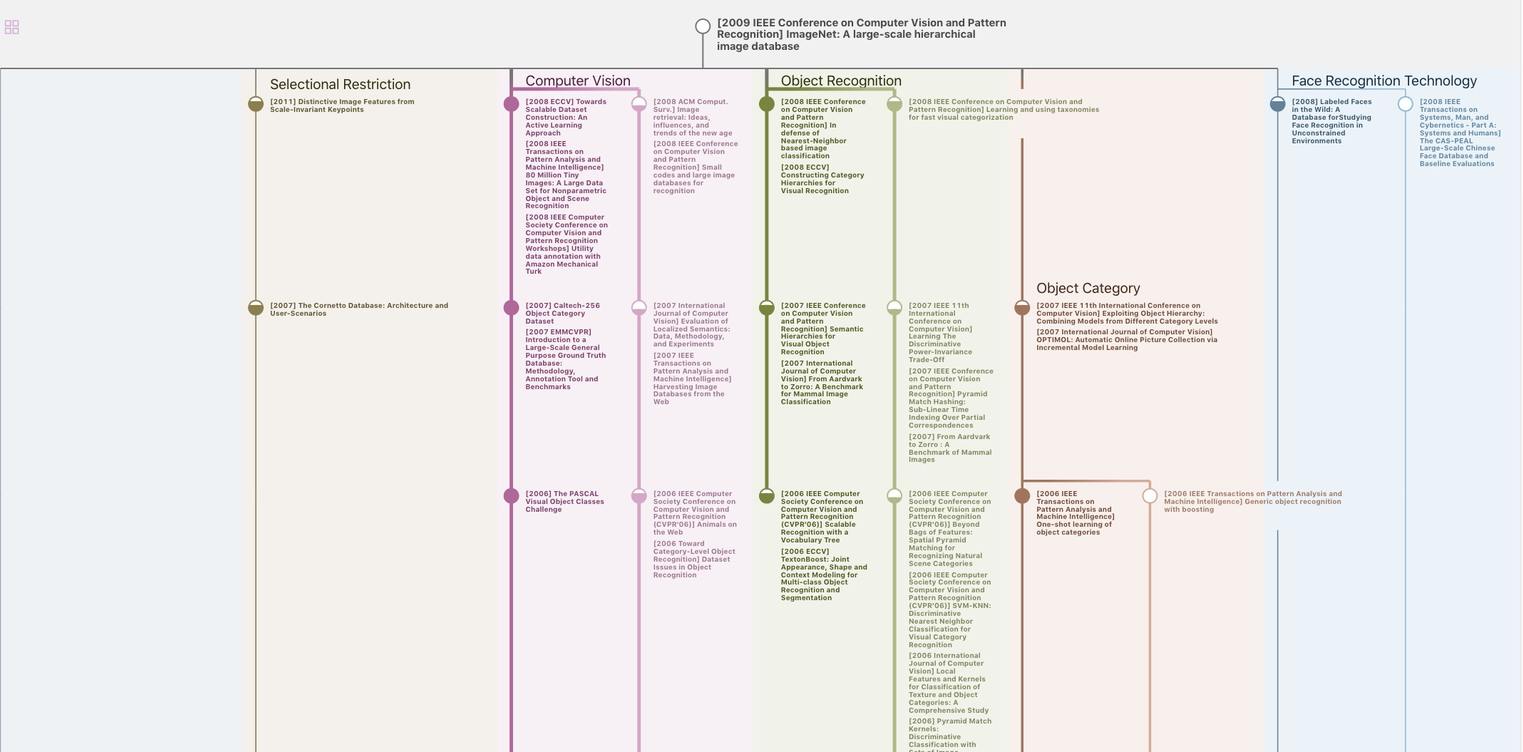
生成溯源树,研究论文发展脉络
Chat Paper
正在生成论文摘要