A review on graph neural networks for predicting synergistic drug combinations
Artificial Intelligence Review(2024)
摘要
Combinational therapies with synergistic effects provide a powerful treatment strategy for tackling complex diseases, particularly malignancies. Discovering these synergistic combinations, often involving various compounds and structures, necessitates exploring a vast array of compound pairings. However, practical constraints such as cost, feasibility, and complexity hinder exhaustive in vivo and in vitro experimentation. In recent years, machine learning methods have made significant inroads in pharmacology. Among these, Graph Neural Networks (GNNs) have gained increasing attention in drug discovery due to their ability to represent complex molecular structures as networks, capture vital structural information, and seamlessly handle diverse data types. This review aims to provide a comprehensive overview of various GNN models developed for predicting effective drug combinations, examining the limitations and strengths of different models, and comparing their predictive performance. Additionally, we discuss the datasets used for drug synergism prediction and the extraction of drug-related information as predictive features. By summarizing the state-of-the-art GNN-driven drug combination prediction, this review aims to offer valuable insights into the promising field of computational pharmacotherapy.
更多查看译文
关键词
Graph neural networks,Drug combination,Synergy prediction,Cancer treatment
AI 理解论文
溯源树
样例
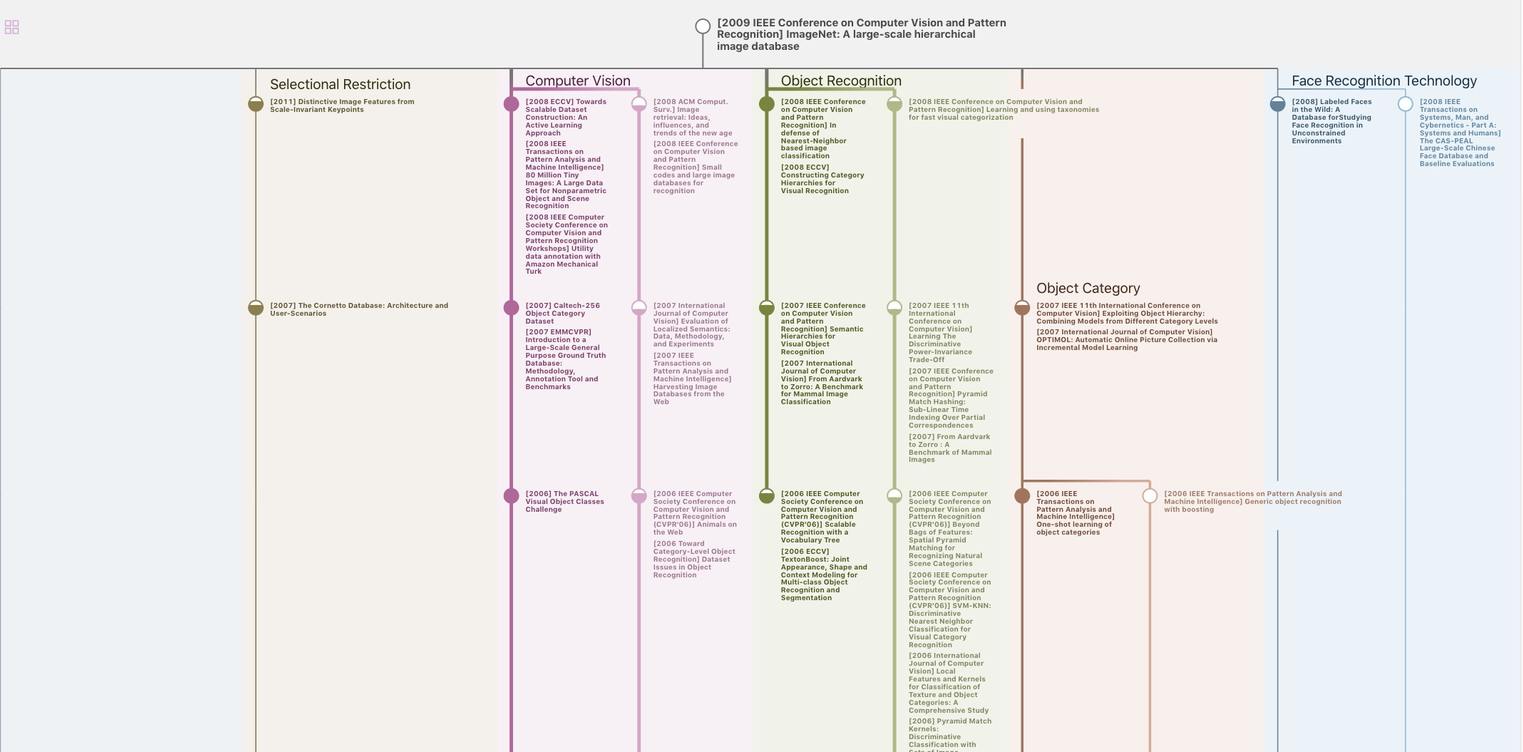
生成溯源树,研究论文发展脉络
Chat Paper
正在生成论文摘要