Privacy-Preserving Multi-Label Propagation Based on Federated Learning
IEEE TRANSACTIONS ON NETWORK SCIENCE AND ENGINEERING(2024)
摘要
Multi-label propagation algorithms (MLPAs) aim to find vertex communities in a complex network or a cloud system by propagating and updating vertex labels, which have been widely applied in customer recommendation, protein molecule discovery, and criminal tracking. As more and more people are concerned about the leakage of their sensitive information, detecting communities without disclosing personal privacy has become a hot topic in complex network analysis. The existing anonymization-based community detection methods have to modify the network structure to protect the sensitive vertices or links, which complicates the recognition of true communities and incurs substantial accuracy loss. In this article, we first propose a federated graph learning model (FGLM) for distributed privacy-preserving network data mining. Second, a federated MLPA for distributed and attributed networks is implemented by adapting a standalone MLPA to FGLM to verify the model's effectiveness. We develop a label perturbation strategy to conceal vertex degrees in distributed label updating and employ a homomorphic encryption system to protect label weights exchanged between the participants. The experiments on real-world and synthetic datasets demonstrate that the new algorithm achieves zero accuracy loss and more than 200% higher accuracy than the simple distributed MLPA without federated learning.
更多查看译文
关键词
Privacy,Data privacy,Federated learning,Homomorphic encryption,Social networking (online),Perturbation methods,Information integrity,Multi-label propagation,Community detection,Graph learning,Privacy-preserving data mining
AI 理解论文
溯源树
样例
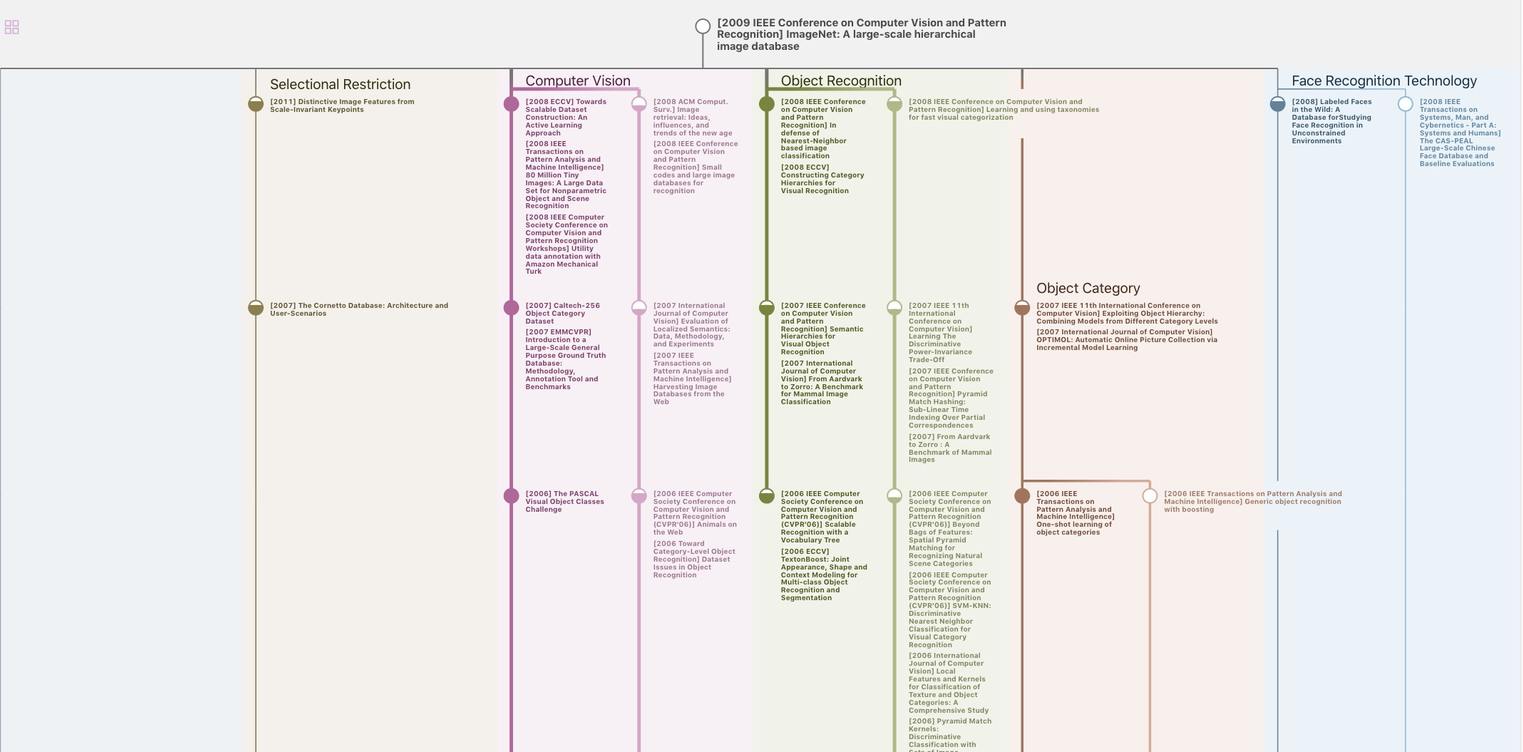
生成溯源树,研究论文发展脉络
Chat Paper
正在生成论文摘要