Proportionality-based association metrics in count compositional data
bioRxiv (Cold Spring Harbor Laboratory)(2023)
摘要
Abstract Motivation Compositional data comprise vectors that describe the constituent parts of a whole. Data arising from various -omics platforms such as 16S and RNA-sequencing are compositional in nature. However, correlations between features on raw counts have no meaningful interpretation. Metrics of proportionality were formulated to address this problem. However, there is an inherent bias that arises when calculating these metrics empirically on count-based measures due to variability in read depths. Results We quantify the bias introduced by empirically calculating proportionality-based association metrics in count data. Additionally, we propose a means of estimating these metrics within a logit-normal multinomial model in pursuit of more accurate estimates. The model-based estimates are shown to outperform empirical estimates in simulated data, and are additionally applied to a mouse embryonic stem-cell single-cell sequencing dataset as well as a pediatric-onset multiple sclerosis metagenomic dataset. Availability and Implementation An R package is available at https://CRAN.R-project.org/package=countprop . Supplementary information Supplementary data are available at Bioinformatics online.
更多查看译文
关键词
association metrics,compositional data,count,proportionality-based
AI 理解论文
溯源树
样例
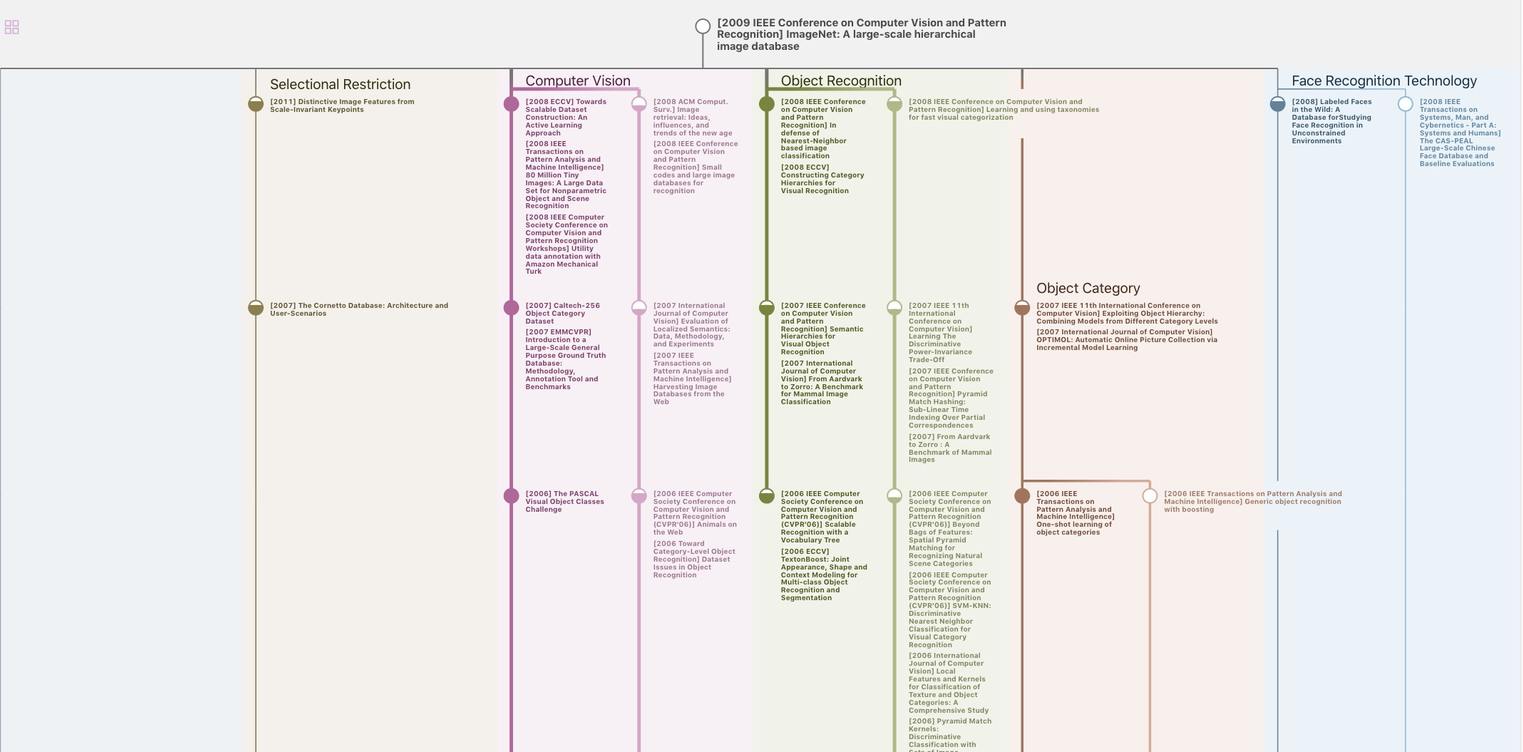
生成溯源树,研究论文发展脉络
Chat Paper
正在生成论文摘要