Sparse factor models of high dimension
arXiv (Cornell University)(2023)
摘要
We consider the estimation of factor model-based variance-covariance matrix when the factor loading matrix is assumed sparse. To do so, we rely on a system of penalized estimating functions to account for the identification issue of the factor loading matrix while fostering sparsity in potentially all its entries. We prove the oracle property of the penalized estimator for the factor model when the dimension is fixed. That is, the penalization procedure can recover the true sparse support, and the estimator is asymptotically normally distributed. Consistency and recovery of the true zero entries are established when the number of parameters is diverging. These theoretical results are supported by simulation experiments, and the relevance of the proposed method is illustrated by an application to portfolio allocation.
更多查看译文
关键词
sparse factor models,high dimension
AI 理解论文
溯源树
样例
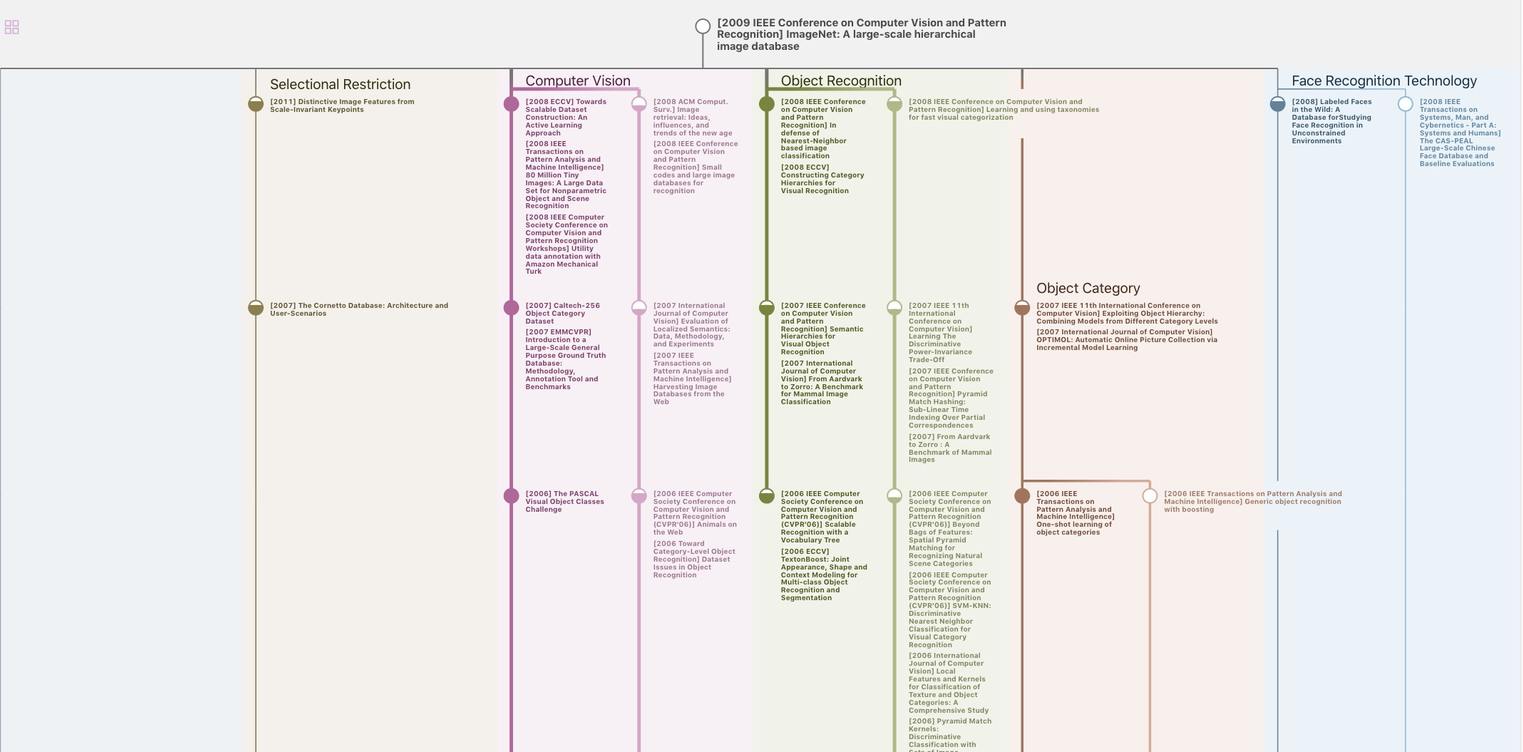
生成溯源树,研究论文发展脉络
Chat Paper
正在生成论文摘要